Why Is Trading So Complex?
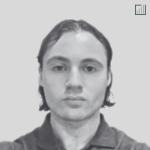
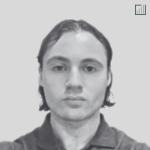
The complexity of trading stems from various factors, ranging from the nature of financial systems to human psychology and technological advancements.
This complexity isn’t merely due to the vast amount of information traders must process but also comes from the various feedback loops and dependencies inherent in financial systems.
We’ll look in more depth at the reasons behind the complexity of trading, concepts like levels in chaotic systems, the discounting mechanism in markets, human psychological influences, and the increased sophistication of traders over time.
Key Takeaways – Why Is Trading So Complex?
- Financial markets are prime examples of Level 2 chaotic systems, where predictions and actions within the system directly influence its behavior.
- Unlike Level 1 chaotic systems – such as weather patterns – where predictions do not affect outcomes, Level 2 systems are characterized by feedback loops that make market dynamics inherently complex and less predictable.
- Trading involves analyzing multidimensional data across interconnected global markets.
- Metrics like inflation comprise data from thousands of variables, requiring nuanced interpretation relative to market expectations.
- Human psychology and algorithmic behaviors introduce unpredictable elements.
- Cognitive biases, herd mentality, and high-frequency trading create market movements that often defy purely rational analysis.
- Markets are probabilistic, a form of thinking that humans traditionally struggle at.
- There are a very large range of unknowns relative to the range of knowns relative to what’s discounted in the price.
- Human limitations in awareness, processing, and the difficulty in thinking probabilistically have led to the continued rise of quantitative methods in finance, where computers excel at memory, processing speed, calculation, and following logic in a disciplined way, while humans focus more on creativity and strategy.
- Tactical trading can be hard because you’re trying to outsmart a very complex system and highly skilled professionals and automated system that can process information faster, more accurately, and less emotionally than any human can achieve.
- Quantitative approaches involve mathematical modeling and risk management.
- Traders use various risk metrics and quality portfolios structuring to optimize performance.
The Nature of Financial Markets as “Level 2” Chaotic Systems
Markets are a very public thing, so there are lots of opinions on them.
But it’s not just a lot of material you have to become familiar with, but a very different way of thinking compared to most things.
Let’s explain.
Feedback Loops
Financial markets are prime examples of “level 2” chaotic systems, where predictions about the system directly influence its behavior.
This characteristic sets trading apart from many other fields, ways of studying other things, and contributes a lot to its complexity.
In these markets, expectations and forecasts become integral parts of the system itself, creating various feedback loops and dependencies.
Level 1 Chaotic Systems
Level 1 Chaotic Systems are those where predictions about the system don’t influence its behavior.
While they may be incredibly complex and sensitive to initial conditions, the act of observing or predicting the system doesn’t change the system itself.
Examples:
- Weather. Meteorologists can make predictions about the weather, but those predictions don’t change what the weather will actually be.
- Many engineering systems are like this – e.g., a bridge’s structural integrity isn’t influenced by predictions about its stability.
- The physical laws that govern the world around us. Those aren’t influenced by what humans and human-made entities think about them.
Level 2 Chaotic Systems
Level 2 Chaotic Systems are those where predictions about the system can directly influence its behavior.
The act of observing or predicting the system becomes part of the system itself, leading to feedback loops where outcomes are influenced by discounted expectations, forecasts, and actions made within them.
Financial markets are level 2 because they’re driven by expectations and decisions.
This self-fulfilling nature of market predictions adds layers of complexity to trading decisions.
Traders can’t consider only fundamental factors, theoretical pricing, and notional equilibrium values but also the impact of the discounted expectations built into the pricing and derivative factors that people trade (e.g., volatility, delta, gamma, etc.) and decisions of other entities within the system that influence the outcome.
- Self-Fulfilling Prophecies – Traders’ expectations can drive market movements. For instance, if a significant number of traders believe a stock’s price will rise, their collective buying can actually cause the price to increase.
- Reflexivity – Introduced by George Soros, this concept suggests that market participants’ biased views can affect the fundamentals they try to understand, creating a continuous feedback loop between perception and reality.
Examples of Level 2 Systems Outside of the Financial Markets:
- Social Media Trends – Predictions about what will go viral can influence people’s sharing behavior, leading to the predicted trend becoming reality.
- Elections – Polls and predictions about election outcomes can influence voter behavior. People might be more likely to vote for a candidate they believe is likely to win, creating a self-fulfilling prophecy. If people believe their vote won’t matter, they might not vote.
- Traffic Patterns – Real-time traffic apps that predict congestion can influence driver route choices, potentially shifting the congestion to different areas or even alleviating it if enough people change their routes.
- Pandemics – Predictions about the spread of a disease can influence people’s behavior (e.g., wearing masks, social distancing), which can in turn impact the actual spread of the disease.
- Economic Bubbles – Predictions of rising prices in a market (e.g., housing, cryptocurrency) can fuel speculative buying in a self-fulfilling way, driving prices even higher and potentially creating a bubble that eventually bursts.
- Social Movements – Predictions about the success or failure of a social movement can influence participation. If people believe a movement is gaining momentum, they might be more likely to join, and hence further fuel its growth.
In short, the distinction between level 1 and level 2 chaotic systems lies in whether predictions about the system become part of the system itself, creating feedback loops that alter its behavior.
Level 2 systems are often found in social contexts where human behavior and expectations are important.
Unlike level 1 systems, where predictions don’t affect outcomes, financial markets are in a constant state of flux influenced by the very act of prediction and analysis.
This dynamic nature makes trading inherently more complex than dealing with systems where cause and effect are more directly observable and less influenced by decision-making about the system itself.
So, financial markets aren’t about “how things are” but how things transpire and how discounted conditions transpire relative to what’s discounted in the price.
It also means that markets are adversarial in terms of adding value relative to a representative benchmark (because one person’s gain is another’s loss).
In these types of systems, you tend to see a small fraction of the players be really good at that, with most underperforming relative to what they could do by simply indexing to broad market indices.
The Multitude of Variables
Markets are a case of high dimensionality.
The Complexity of Metrics
Economic indicators are important for trading decisions are often far more complex than they appear on the surface.
Inflation, for instance, is not a simple, singular number but a metric, in some measures, composed of over 100,000 products and services, each with different weightings, procedures, and adjustments.
This composition makes interpreting inflation data a complex task requiring nuanced understanding and careful analysis.
And, as mentioned, markets don’t transpire based on what a particular data point is right now but how it transpires relative to what’s discounted.
And inflation is just one of many variables that affect asset prices at the macro level (e.g., discounted growth, changes in risk premiums, changes in discount rates), as well as their volatility and all the various multi-order factors that people can trade through things like derivatives.
Global Variations in Economic Metrics
Adding to this complexity is the fact that different countries have various versions of these economic indicators, each calculated and weighted according to local economic conditions and priorities.
Traders have to understand these differences when making decisions based on them, further complicating the analysis process.
The Discounting Mechanism in Markets
Markets don’t simply react to current data points but to how these data points compare to what has already been discounted or expected over time by market participants.
This discounting mechanism means that traders must constantly assess not just the current point of various data and changes in discounted value but their relative value compared to market expectations over time, adding another layer of complexity to trading decisions.
You really have to know what’s already baked in the price and know how the many influences impacting prices come in or are expected to come in relative to that.
The range of what you know is going to be low relative to the range of what you don’t know relative to what’s factored into the price.
Human Psychology in Trading
Mental Demands
It’s impossible for the human brain to know everything that’s going on.
The brain has limited awareness, limited processing capacity, and is subject to various harmful biases and emotions.
Markets are also probabilistic, and humans don’t necessarily like thinking in a probabilistic way.
When someone makes a prediction, that’s just one small slice of the distribution out of many outcomes that are possible.
This is why computers and the quantitative aspect of the profession have taken off.
They can be used for memory, processing speed, brute-force calculation, and following instructions.
Humans are central to the creative and strategic part.
Cognitive Biases and Emotional Decision-Making
Human psychology is important when it comes to trading.
Cognitive biases such as confirmation bias, loss aversion, and overconfidence can heavily impact trading decisions.
These psychological factors introduce unpredictable elements into market behavior, making rational analysis and prediction even more challenging.
Always, someone somewhere is making decisions in markets that won’t be reflected by models that could have known in advance.
This is what makes markets probabilistic and not deterministic like many prefer outcomes to be.
Herd Mentality and Market Sentiment
The tendency for traders to follow the crowd, known as herd mentality, can lead to market movements that defy logical analysis.
Humans tend to care about what other people think about their opinions, which in turn influences what they think and do.
Understanding and predicting these sentiment-driven shifts adds another layer of complexity to trading strategies.
This is a lot of what momentum trading strategies are built on.
And not just human tendencies but also algorithmic tendencies, such as how market maker hedging operations influence the underlying asset and concepts like momentum ignition.
Technological Advancements and Trading Complexity
High-Frequency Trading and Algorithmic Strategies
The advent of high-frequency trading strategies and sophisticated algorithmic uses in markets has dramatically increased the speed and complexity of market operations.
These technologies can execute trades in microseconds, reacting to market changes better, faster, and less emotionally than any human trader could.
In the old days, markets were purely discretionary and humans manually did all the trades.
The first steps toward automation began in the early 1970s with the introduction of electronic order routing systems like the New York Stock Exchange’s DOT system.
The 1980s saw the rise of program trading, where large baskets of stocks were bought or sold automatically based on pre-programmed instructions. This marked a big shift toward more systematic and automated trading.
The 2000s saw the explosive growth of HFT and algorithmic trading, driven by advances in computing power and connectivity.
By the mid-2000s, it’s estimated that around half of all trades in US equity markets were executed by automated systems.
The percentage today depends heavily on the market and country.
Today, algorithmic trading accounts for an estimated 60-75% of trading volume in major developed markets like the US, Europe, and Asia. This percentage is even higher in certain asset classes like futures and options.
Big Data and Advanced Analytics
The availability of vast amounts of data and advanced analytical models and software has both enhanced trading capabilities and increased complexity.
Traders have to now navigate and interpret enormous datasets, using machine learning and artificial intelligence to generate insights and make decisions.
Trading for the discretionary, non-data-dependent trader is very difficult accordingly.
Regulatory Environment and Compliance Challenges
Evolving Legal Frameworks
The regulatory landscape for trading is constantly changing, with new rules and regulations being introduced regularly.
Staying compliant with these changing legal requirements adds another layer of complexity to trading operations.
Cross-Border Trading Challenges
For traders operating in multiple jurisdictions, navigating different regulatory environments adds significant complexity.
Understanding and adhering to various national and international regulations requires extensive knowledge and careful management.
The Relationship Between Different Asset Classes
Cross-Asset Correlations
Understanding the relationships between different asset classes, such as stocks, bonds, commodities, and currencies, can be important for effective trading.
These correlations can shift over time, adding complexity to portfolio management and risk assessment.
Alternative Investments and Exotic Instruments
The proliferation of alternative investments (e.g., private equity, venture capital, reinsurance) and exotic financial instruments, such as cryptocurrency, structured products, and complex derivatives, further complicates trading.
Most brokers available to everyone represent just a fraction of the available instruments to trade.
Each of these asset classes comes with its own set of rules, risks, and market dynamics.
Market Microstructure and Liquidity Considerations
Order Book Dynamics
Understanding market microstructure, including order book dynamics and trade execution strategies, is essential for efficient trading.
The complexity of modern market structures, with multiple venues/exchanges and dark pools, adds layers to trading operations.
Liquidity Risk Management
Managing liquidity risk, especially in less liquid markets or during periods of market stress, requires another type of analysis and risk management.
The ability to enter or exit positions without significantly impacting market prices is an important consideration, especially as traders become larger in size.
Global Interconnectedness and Geopolitical Factors
24/7 Global Markets
The interconnectedness of global financial markets means that events in one part of the world can have immediate impacts elsewhere.
It also means that some things are more correlated than they were in the past.
Geopolitical Risk Assessment
Assessing and pricing in geopolitical risks adds another layer of complexity to some trading decisions.
Traders must consider how political events, international relations, and policy changes might impact markets across various regions and asset classes.
Geopolitical risks and realities might cause some traders to structure their portfolios differently.
For instance, if a trader believes that the Chinese renminbi will have a larger international role, gold will be more valued in foreign currency reserves, and countries will increasing want key natural resources in addition to financial reserves, this might change the way they structure their portfolio.
Quantitative Finance
Quantitative finance and trading are increasingly relevant over time.
This area represents one of the most difficult and mathematically rigorous areas in the financial industry.
Quant traders leverage mathematical models, statistical techniques, and computational algorithms to identify trading opportunities, make markets, manage risk, and optimize portfolio performance.
Complexities in Quantitative Finance and Trading
Data Complexity
- High Dimensionality – As mentioned at the beginning, quant traders deal with large datasets comprising numerous variables (price, volume, economic indicators) across different time frames.
- Noisy Data – Financial data is inherently noisy due to market volatility, requiring sophisticated filtering techniques (e.g., smoothing techniques, Kalman filters).
- Non-Stationarity – Markets change over time, making it challenging to build models that remain valid in different regimes/environments.
Mathematical Modeling
- Stochastic Processes – Modeling asset prices often involves stochastic calculus, including Brownian motion and Ito’s lemma (provides a way to differentiate functions of stochastic processes, important for modeling financial asset prices and derivatives).
- Partial Differential Equations (PDEs) – Used in option pricing models like the Black-Scholes equation.
- Optimization Problems – Portfolio optimization requires solving complex optimization problems under constraints.
Computational Requirements
- Algorithmic Trading – High-frequency trading algorithms require low-latency systems and real-time data processing.
- Parallel Computing – Simulating models (e.g., Monte Carlo simulations) often necessitates parallel computing resources.
Specific Metrics Used by Quant Traders
Risk-Adjusted Performance Metrics
- Sharpe Ratio – Measures the excess return per unit of risk (standard deviation). It’s used for comparing the efficiency of different investment strategies.
- Formula: Sharpe Ratio = E(Rp – Rf) / σp
- Sortino Ratio – Similar to the Sharpe ratio but focuses on downside risk (semi-standard deviation). It’s used for better assessment when the return distribution is not symmetrical.
- Formula: Sortino Ratio = E(Rp – Rf) / σd
Risk Metrics
- Value at Risk (VaR) – A risk metric that estimates the potential loss in value of a portfolio over a defined period for a given confidence interval. It sets risk limits and influences capital allocation.
- Conditional Value at Risk (CVaR) – Expected loss exceeding the VaR threshold. Understanding tail risk and extreme events.
- Maximum Drawdown – The largest peak-to-trough decline in portfolio value. Assessing historical risk and vulnerability to losses.
Performance Attribution Metrics
- Alpha – The excess return of a portfolio relative to a benchmark index. Measuring active return on investment.
- Beta – Measures the volatility of a security or portfolio relative to the market. Understanding systematic risk exposure.
- Information Ratio – Ratio of portfolio returns above the returns of a benchmark to the volatility of those returns, evaluating the consistency of excess returns.
- Formula: Information Ratio = (Rp – Rb) / σ(Rp – Rb)
Statistical Metrics
- P-values and t-statistics – Assess the statistical significance of model coefficients. Validating hypotheses and model robustness.
- Autocorrelation and Partial Autocorrelation – Measure the correlation of a time series with its own past and future values. Identifying patterns and mean-reverting behavior.
Portfolio Metrics
- Risk Parity Weights – Allocating capital so that each asset contributes equally to portfolio risk or equally to various predefined market environments. For diversification and risk management.
- Expected Shortfall – The average of the worst losses (beyond the VaR threshold). Understands potential extreme losses.
Dynamic Leverage Adjustment
Some quant approaches will dynamically adjust leverage based on predefined criteria.
Mechanism
- Leverage Ratio (L): Defined as: L = Total Exposure / Equity
- Adjustment Triggers:
- Volatility Scaling – Increase leverage when volatility is low, decrease when high.
- Tail Risk Considerations – Risk goes beyond volatility to various tail risk considerations like drawdowns, preventing what’s unacceptable, skew, kurtosis, and higher-order moments.
- Risk Targeting – Maintain a constant level of portfolio risk by adjusting exposure.
- Margin Requirements – Adjust leverage in response to changes in margin calls.
Basic Math
- Target Volatility Model: Target Exposure = (Target Volatility / Estimated Volatility) * Equity
Considerations
- Transaction Costs – Frequent adjustments incur costs that can erode returns.
- Model Risk – Incorrect volatility estimates can lead to suboptimal leverage.
- Regulatory Constraints – Leverage limits imposed by regulators must be adhered to.
Applications
- Trend-Following Strategies – Adjust leverage based on the strength of a trend.
- Risk Management – Reduce leverage during market stress.
Advanced Portfolio Management Techniques
Factor Models
- Multi-Factor Models – Decompose returns into exposure to various factors (e.g., size, value, momentum).
- Fama-French Three-Factor Model – Incorporates market risk, size, and value factors. Explains portfolio performance and constructing factor-based strategies. (This is an older academic model and what they do in industry is more complex and nuanced.)
Portfolio Optimization
- Mean-Variance Optimization – Balances expected return against variance (risk).
- Efficient Frontier – Set of optimal portfolios offering the highest expected return for a defined level of risk.
- Black-Litterman Model – Incorporates investor views into mean-variance optimization. Overcoming limitations of traditional mean-variance approaches.
- Optimization with Other “Moments” – Traders will optimize based on skew in the projected distribution, kurtosis (thickness in the tails of the distribution), and higher-order moments that look at various features of the tails.
Risk Management Techniques
- Stress Testing – Simulate portfolio performance under extreme market conditions.
- Scenario Analysis – Evaluate portfolio under hypothetical situations.
- Simulation – Running various scenarios over lots of historical and synthetic data.
Algorithmic Trading Strategies
Statistical Arbitrage
- Pairs Trading – Trading two correlated assets when their price relationship diverges.
Market Microstructure Models
- Analyzing the impact of trade execution on prices.
Agent-Based Modeling
Agent-based modeling in finance simulates individual buyers and sellers, representing their sizes, motivations, and behaviors to understand how markets transpire over a wide range of outcomes.
This approach reveals how diverse agents interact, leading to emergent patterns in how markets are likely to trade and how to optimize your own portfolio in light of that.
This also gets into things like game theory and other frameworks.
Decision-Making Processes
Machine Learning and AI
- Supervised Learning – Predict future asset prices or returns using historical data.
- Unsupervised Learning – Identify hidden patterns or clusters in data.
- Reinforcement Learning – Develop trading strategies that adapt and learn from the environment.
High-Frequency Trading Considerations
- Latency Arbitrage – Capitalizing on speed advantages to act on information before competitors.
- Order Book Dynamics – Analyzing the supply and demand at different price levels. Placing orders strategically to improve execution quality.
Behavioral Finance Integration
- Market Sentiment Analysis – Using natural language processing to gauge sentiment from news and social media.
- Cognitive Biases – Recognizing and mitigating biases (e.g., overconfidence, herding) in model assumptions.
Regulatory and Ethical Considerations
- Compliance – Ensuring trading strategies adhere to legal and regulatory requirements.
- Regulatory – How does regulation impact what traders do? Who’s buying and selling for non-economic reasons?
How Can Traders Manage Complexity?
Due to the complexity of financial markets, traders can adopt strategies that balance risk and reward while navigating unknowns.
Here are key actions they can take:
Diversification
Spreading investments across different asset classes (e.g., stocks, bonds, commodities) can reduce exposure to any single market or sector’s volatility, creating a more stable portfolio.
We have example portfolio structures here.
Active traders often trade in the context of a structure rather than thinking of trading as an individual, one-after-the-other type of pursuit.
Tail Risk Management
Traders should examine the potential for extreme, rare market events that could significantly impact their portfolios.
This can be done through stress testing, diversifying, and hedging with options to reduce the risk of outsized losses.
Risk-Adjusted Metrics
For active traders, using performance metrics like the Sharpe ratio, Sortino ratio, among others, traders can look at returns in relation to risk.
This helps traders know that they aren’t taking unnecessary risks for marginal gains.
Regular Rebalancing
Markets fluctuate constantly, so rebalancing portfolios ensures that they stay aligned with the trader’s risk tolerance and objectives.
Behavioral Awareness
Recognizing psychological biases such as herd mentality, confirmation bias, overconfidence, or loss aversion helps traders avoid irrational decisions.
Combining these techniques can help traders optimize their performance and risk management.
Is Trading a Reasonable Career Given What We’ve Covered?
Trading markets for a living is a challenging yet intriguing pursuit.
It’s not impossible, but it requires a combination of skill, discipline, and resilience that few master quickly.
Active, tactical trading involves making frequent decisions to capitalize on short-term market movements. This contrasts sharply with strategic asset allocation and indexing, which are passive, long-term approaches designed to build wealth steadily by tracking market performance.
Active trading demands far more time, effort, and expertise, as you’re competing in a space dominated by professional people and algorithms that can process information faster, more accurately, and without the emotional biases of humans.
If you’re truly interested in trading, the best way to learn is by trying.
Start small and treat it as a learning experience.
Expect to make mistakes and lose money – this is part of the education.
Each loss can teach you important lessons about risk management, emotional control, and market behavior.
Starting with a manageable amount of capital, you can minimize the financial sting while maximizing the learning opportunities.
Over time, through trial and error, you’ll develop a better understanding of what works and what doesn’t.
If you approach trading with humility, curiosity, and a willingness to adapt, you can grow your skills.
While trading for a living is difficult, for those with passion and perseverance, the challenge itself can be rewarding.
Even if markets don’t ultimately become your career, the experience can be helpful in a wide range of pursuits.
With that said, let’s consider the main two approaches of going about trading markets.
Strategic Asset Allocation
Strategic asset allocation is often the more realistic and sustainable approach for building wealth over time, especially for individual investors.
Unlike tactical trading, which requires attempting to outsmart markets and professionals in a highly competitive and complex environment, strategic allocation focuses on long-term goals and a disciplined investment strategy.
This method involves setting a target mix of asset classes – such as stocks, bonds, and cash – that aligns with your risk tolerance, investment horizon, and financial goals.
Sticking to this allocation and rebalancing periodically, you avoid the emotional decision-making that often leads to poor outcomes in active trading.
One common and effective way to implement strategic asset allocation is through passively indexing.
You gain broad market exposure without trying to pick individual winners by investing in low-cost index funds or exchange-traded funds (ETFs).
This approach allows you to capture the market’s average returns over time, which have historically been strong over the long run.
The key driver of success in this strategy is your savings rate.
Consistently contributing to your investments – regardless of the market – allows the power of compounding to work in your favor.
Over decades, this combination of disciplined saving and passive indexing can help generate wealth with far less stress and complexity than tactical trading.
The Difficulty of Tactical Trading
When trading tactically in financial markets, you’re stepping into one of the most competitive games in the world.
Markets are very complex systems that operate on the combined knowledge and actions of countless participants, from individual traders to institutional firms.
In terms of alpha generation, you’re not only competing against other individuals but also against highly skilled professionals who dedicate their careers to understanding and capitalizing on market movements – as well as computer programs, as much trading is automated these days.
These include hedge fund managers, quantitative analysts, and proprietary traders armed with advanced resources, systems, and access to vast amounts of data.
The complexity of the financial system, as we covered here, can’t be understated.
Prices are influenced by a multitude of factors, which interact in very difficult-to-predict ways.
Tactical trading often involves making quick decisions based on market trends, patterns, or news.
However, what might seem like a clear opportunity to you has likely been analyzed by others with more resources, better analysis, and deeper insights.
Moreover, trading is also about managing risks effectively when you’re wrong.
Professionals excel in this aspect through rigorous risk management strategies (e.g., diversifying, use of options to hedge risks, balancing of positions, careful position sizing) and extensive backtesting of their methods.
They also have the psychological resilience to remain disciplined under pressure – something that many retail traders struggle to achieve.
It’s also important to understand that trading tactically means you’re competing against algorithms capable of processing information and executing trades faster than anyone can blink, as well as seasoned experts who often have access to insider-level insights and market-moving resources.
Success in this environment requires more than just intuition or basic knowledge.
Recognizing the steep competition is the first step toward approaching tactical trading with the respect and caution it demands.