Frameworks for Understanding Financial Markets
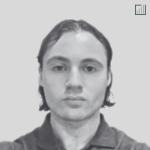
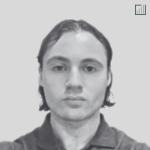
Financial markets are complex, dynamic systems that have long challenged our understanding.
To make sense of these environments, researchers and practitioners have developed various frameworks and theories.
These approaches try to better understand market behavior, price movements, and the interconnectedness of financial systems.
Here’s we’ll explore several key frameworks for understanding financial markets, discussing their core concepts and potential value for traders.
Key Takeaways – Frameworks for Understanding Financial Markets
- Chaos Theory
- Network Theory
- Percolation Theory
- Behavioral Finance
- Efficient Market Hypothesis (EMH)
- Fractal Market Hypothesis
- Adaptive Market Hypothesis
- Complex Adaptive Systems
- Game Theory
- Quantum Finance
- Econophysics
- Stochastic Control
- General Equilibrium Theory
- Arbitrage Pricing Theory (APT)
- Information Theory in Finance
- Rational Expectations Theory
- Incomplete Markets Theory
- Extreme Value Theory
- Market Microstructure Theory
- Bayesian Methods
Key Highlights
- Chaos theory reminds traders that markets are inherently unpredictable.
- Small events can trigger large movements.
- Empgasizes the importance of strong risk management and adaptability rather than relying on precise long-term forecasts.
- Network theory helps traders understand market interconnectedness.
- It’s valuable for assessing systemic risks, counterparty exposure, and how shocks might propagate through the financial system.
- Behavioral finance insights enable traders to recognize cognitive biases in themselves and the market.
- This awareness can improve decision-making and help in timing entries and exits based on market sentiment.
- Complex adaptive systems view encourages traders to consider the holistic market environment.
- It highlights how different participants interact, adapt, and co-evolve.
- This influences overall market dynamics.
- Game theory provides a framework for anticipating other market participants’ actions.
- Most useful in strategic situations like mergers and acquisitions, central bank interventions, or high-frequency trading environments.
Chaos Theory
Chaos theory examines complex systems that are highly sensitive to initial conditions.
In financial markets, this theory suggests that small changes can lead to significant and unpredictable outcomes.
Key Aspects
- Butterfly Effect – Minor events can trigger major market movements
- Fractals – Market patterns repeat at different scales
- Strange Attractors – Markets tend to move around certain states but never settle
- Level 1 and Level 2 Chaos – Level 1 chaotic systems don’t change in response to predictions about them (e.g., the weather), while level 2 chaotic systems change based on forecasts (e.g., financial markets)
Value for Traders
Chaos theory reminds traders that markets have lots of unknowns that can’t be known.
It encourages a focus on risk management, diversification, and adaptability rather than attempting to make precise long-term forecasts.
Network Theory
Overview
Network theory analyzes the structure and dynamics of interconnected systems.
In finance, it helps understand how different market participants, assets, or institutions are linked and how these connections influence market behavior.
Key Aspects
- Nodes and Edges – Representing market participants and their relationships
- Centrality – Identifying key players in the market
- Contagion – Understanding how shocks can propagate through the system
- Clusters – Identifying groups of closely connected nodes in the network
- Degree Distribution – Analyzing the number of connections each node has
- Network Resilience – Assessing the network’s ability to withstand shocks
- Path Length – Measuring the shortest path between two nodes
Value for Traders
Network analysis can help traders identify potential systemic risks and understand how news or events might spread through markets.
It’s useful for assessing counterparty risk and understanding market liquidity dynamics.
For traders who use agent-based modeling, they can model how money and credit flows are likely to transpire based on the relative sizes and motivations of the buyers and sellers in the markets they track.
Percolation Theory
Overview
Percolation theory, originally from physics, studies how information or events spread through a system.
In finance, it can model how market sentiment or crises propagate.
Key Aspects
- Threshold Effects – Critical points where small changes lead to system-wide shifts
- Clustering – Formation of connected groups within the market
- Phase Transitions – Sudden changes in market state or behavior
- Connectivity – How individual elements become interconnected (leading to large-scale network formation)
- Fractality – The self-similar patterns that emerge as the system evolves.
- Criticality – The point at which the system is poised for large-scale shifts – often preceding a major change in behavior or state
- Robustness – The system’s ability to maintain functionality despite disruptions or failures in parts of the network
Value for Traders
Percolation theory can help traders anticipate potential market tipping points.
It’s useful for understanding how trends might suddenly accelerate or reverse, and for identifying potential scenarios where volatility flares up.
Behavioral Finance
Overview
Behavioral finance incorporates psychological concepts to explain market anomalies and trader behavior that traditional finance theories struggle to account for.
Key Aspects
- Cognitive Biases – Systematic errors in judgment affecting investment decisions
- Herd Behavior – Tendency of investors to follow the crowd
- Prospect Theory – How traders perceive and evaluate risk and reward
- Anchoring – Relying too heavily on the first piece of information encountered
- Overconfidence – Overestimating one’s own ability to predict market movements
- Loss Aversion – The tendency to prefer avoiding losses over acquiring equivalent gains
Value for Traders
Understanding behavioral finance can help traders recognize their own biases and those of the market.
It helps understand market sentiment and can help in timing entry and exit points based on psychological factors.
Efficient Market Hypothesis (EMH)
Overview
The Efficient Market Hypothesis posits that asset prices fully reflect all available information, making it hard to consistently outperform the market.
Key Aspects
- Weak, Semi-Strong, and Strong Forms – Different levels of market efficiency
- Random Walk – Price movements are essentially unpredictable
- Information Absorption – Markets quickly incorporate new information
Value for Traders
While many traders try to beat the market, understanding EMH encourages a focus on cost-effective, diversified strategies, such as index funds held passively.
It also highlights the importance of reacting quickly to new information and the challenges of consistently generating alpha.
Fractal Market Hypothesis
Overview
The Fractal Market Hypothesis suggests that markets have a fractal structure, with similar patterns occurring at different time scales.
Key Aspects
- Self-Similarity – Market patterns repeat across different timeframes
- Multi-Frequency Trading – Different investor groups operate on various time horizons
- Market Stability – Liquidity is maintained by the interaction of different time horizons
Value for Traders
Sometimes used for market liquidity and potential instability when certain investor groups dominate.
Adaptive Market Hypothesis
Overview
The Adaptive Market Hypothesis combines principles from EMH with evolutionary and cognitive psychology.
Suggests that market efficiency is not constant but varies over time and across markets.
Key Aspects
- Market Ecology – Markets as a competitive evolutionary environment
- Adaptive Behavior – Strategies that work in one period may fail in another
- Time-Varying Risk Premia – Expected returns vary with the market environment
- Survivor Bias – Only successful strategies and participants persist over time.
- Behavioral Flexibility – The ability of market participants to adjust their strategies
- Innovation – New financial instruments and strategies evolve as markets change
- Market Efficiency as Dynamic – Market efficiency isn’t static but fluctuates over time with the adaptation of participants
- Competition and Cooperation – Relationship between competitive and cooperative behaviors among market participants
Value for Traders
This hypothesis encourages traders to adapt their strategies to changing markets.
It suggests arbitrage opportunities exist but are temporary.
Emphasizes the need for learning and adaptation.
Complex Adaptive Systems
Overview
This framework views financial markets as systems composed of many interacting agents, capable of learning and adapting to changes in their environment.
Key Aspects
- Emergence – Complex behaviors arise from simple rules
- Feedback Loops – Actions of agents influence the overall system, which in turn affects individual behavior
- Non-linearity – Outcomes are not proportional to inputs
- Self-Organization – Systems spontaneously form structured patterns without central control
- Adaptation – Agents within the system modify their behavior in response to changes in the environment
- Co-evolution – Agents and the environment evolve together, influencing each other’s development
- Diversity – Variety among agents leads to adaptability in the system (lots of different types of participants with different means and what drives them to do what they do)
- Path Dependence – The system’s history influences its current and future behavior
- Actions, Sizes, Motivations – In financial markets, who’s buying and selling, how big are they, and what are they motivated by?
Value for Traders
Understanding markets as complex adaptive systems can help traders anticipate potential regime changes and recognize the limitations of linear forecasting models.
It encourages a holistic view of market dynamics.
There are many buyers and sellers with all sorts of motivations with varying degrees of buying and selling power.
Game Theory
Overview
Game theory analyzes strategic decision-making in competitive situations.
In finance, it can model interactions between market participants and inform trading strategies.
Key Aspects
- Nash Equilibrium – Stable state where no participant can gain by unilaterally changing strategy
- Prisoner’s Dilemma – Situations where individual rationality leads to collective irrationality
- Information Asymmetry – Differences in knowledge between market participants
- Zero-Sum Games – Situations where one participant’s gain is exactly another’s loss (e.g., alpha in financial markets)
- Dominant Strategy – A strategy that is best for a player, no matter what the opponents do
- Pareto Efficiency – Outcomes where no one can be made better off without making someone else worse off
- Mixed Strategy – Randomizing over possible moves to keep opponents uncertain (common in games with imperfect information like poker)
- Backward Induction – Solving a game by analyzing it from the end and reasoning backward to the beginning
- Cooperative Games – Games where players can form coalitions and negotiate binding agreements
- Sequential Games – Games where players make decisions one after another, with each aware of the previous actions
- Repeated Games – Games that are played multiple times, allowing strategies to evolve based on past outcomes
Value for Traders
Game theory can help traders understand market dynamics in situations like mergers and acquisitions, central bank interventions, high-frequency trading environments, among any number of market behaviors.
Some quantitative trading firms have hired game theory specialists.
It provides a framework for anticipating other market participants’ actions.
Quantum Finance
Overview
Quantum finance applies principles from quantum mechanics to financial modeling.
Offers new ways to understand uncertainty and non-linear relationships in markets.
Key Aspects
- Superposition – Multiple states existing simultaneously
- Entanglement – Correlated behavior between separate entities
- Wave Function Collapse – How observation affects outcomes
- Quantum Tunneling – The possibility of transitioning between states that would be classically forbidden
- Quantum Uncertainty – The inherent unpredictability and probabilistic nature of outcomes (i.e., dovetails with markets where probabilistic thinking is key)
- Quantum Annealing – Optimization of complex financial problems using quantum computation techniques
Value for Traders
While still a developing field, quantum finance offers potential for more sophisticated risk management and options pricing models.
It may provide new ways to understand and quantify market uncertainty.
Quantum algorithms also may give the potential to solve things faster, which can provide certain advantages.
Econophysics
Overview
Econophysics applies theories and methods from physics to economic and financial problems, often focusing on statistical mechanics and complex systems.
Key Aspects
- Power Laws – Describing the frequency of certain events in financial markets
- Phase Transitions – Sudden changes in market states
- Agent-Based Modeling – Simulating market behavior from individual interactions
- Scaling Laws – Relationships that remain consistent across different scales in financial systems
- Fat Tails – The occurrence of extreme events more frequently than predicted by normal distributions
- Stochastic Processes – Random processes that model the probabilistic behavior of financial markets, as opposed to deterministic processes
- Market Microstructure – The study of how market mechanisms impact price formation and liquidity
- Lévy Flights – A model of financial market movements characterized by large jumps or “flights” interspersed with smaller steps
- Thermodynamic Analogies – Applying concepts from thermodynamics, such as entropy, to understand market dynamics and efficiency
Value for Traders
Econophysics can provide analytical methods to better understand market crashes, bubble formation, and the distribution of returns.
Its models can help in risk assessment and in understanding extreme market events.
Stochastic Optimal Control
Overview
Stochastic control provides a mathematical framework for decision-making in financial markets characterized by the unknown.
It applies control theory principles to systems where random factors influence outcomes.
In finance, this approach helps model and optimize trading strategies, risk management, and asset allocation.
The framework acknowledges that financial markets can only be known within the context of probability distributions, with asset prices and returns following stochastic processes.
Incorporating this randomness into models allows for more realistic representations of market dynamics and behavior.
As humans, we tend to prefer more determinism when that simply doesn’t align with reality.
This approach enables the development of strategies that can adapt to changing markets and optimize performance over time.
Key Aspects
- State variables – In financial applications, these often represent wealth, portfolio value, or asset prices.
- Control variables – These typically include trading decisions, such as asset allocation or trading strategies.
- Stochastic processes – Used to model the unpredictable nature of financial markets, including asset returns and price movements.
- Optimization – The goal is to maximize or minimize an objective function, like expected returns or risk-adjusted performance, over a given time horizon.
- Dynamic programming – This technique is used to solve complex optimization problems by breaking them down into smaller, more manageable subproblems.
- Continuous-time models – Often employing stochastic differential equations to represent the evolution of state variables over time.
Value for Traders
Stochastic control offers several benefits for traders and financial professionals:
- Risk management – Explicitly modeling uncertainty helps develop stronger risk management strategies.
- Portfolio optimization – Enables the creation of dynamic asset allocation strategies that adapt to changing market environments.
- Algorithmic trading – Provides a foundation for developing trading algorithms that can react to real-time market data – or whenever they choose to run the system. For example, one popular quantitative hedge fund runs its algorithmic trading system three times per day.
- Performance evaluation – Offers a framework for assessing trading strategies under various market scenarios. Stress testing and forward testing are a big part of modern finance.
- Long-term planning – Assists in developing trading strategies that balance short-term fluctuations with long-term goals.
Leveraging stochastic control techniques enables traders can make more informed decisions, better manage risk, and potentially achieve superior risk-adjusted returns.
General Equilibrium Theory
Overview
General Equilibrium Theory, developed by economists like Kenneth Arrow and Gérard Debreu, provides a mathematical model for understanding how prices, production, and consumption of goods and services are determined in an economy with multiple interacting markets.
This theory tries to explain the functioning of the entire economy as a system of interdependent markets.
Key Aspects
- Interconnectedness – All markets in an economy are interconnected, with changes in one market affecting others.
- Price mechanism – Prices adjust to balance supply and demand across all markets simultaneously.
- Pareto efficiency – The equilibrium state achieved is often Pareto efficient, meaning no individual can be made better off without making someone else worse off.
- Perfect competition – The model often assumes perfect competition in all markets.
- Walrasian auctioneer – A conceptual device used to explain how markets clear simultaneously.
- No arbitrage – In equilibrium, there are no opportunities for riskless profit. Used to simplify
Value for Traders
General Equilibrium Theory provides traders with a holistic view of the economy, helping them understand:
- How changes in one market can ripple through the entire economy.
- The importance of considering multiple markets when making trading decisions.
- The concept of market clearing and its impact on asset prices.
- The role of prices in allocating resources efficiently.
- The limitations of partial equilibrium analysis.
- The potential long-term equilibrium state of markets, which can inform long-term strategies.
The theory’s assumptions may not always hold in real-world markets, but understanding these concepts can help traders develop a view of market dynamics and potential long-term trends.
Arbitrage Pricing Theory (APT)
Overview
Arbitrage Pricing Theory (APT), developed by Stephen Ross, is a multi-factor model that provides an alternative to the Capital Asset Pricing Model (CAPM) for explaining asset returns.
APT posits that an asset’s returns can be predicted using the linear relationship between the asset’s expected return and a number of macroeconomic variables that capture systematic risk.
Key Aspects
- Multi-factor model – APT considers multiple factors that can affect an asset’s return, unlike CAPM which only uses market risk.
- Linear relationship – The theory assumes a linear relationship between risk factors and expected returns.
- Risk factors – Common factors might include GDP growth, inflation, interest rates, and market indices.
- Factor sensitivities – Each asset has different sensitivities (betas) to these risk factors.
- Flexibility – The theory doesn’t specify which or how many factors should be used, allowing for customization.
Value for Traders
APT offers several benefits for traders:
- More thorough risk assessment – APT can provide a more nuanced understanding of an asset’s risk profile given it considers multiple factors.
- Improved portfolio construction – Traders can use APT to build portfolios that are hedged against specific macroeconomic risks.
- Identifying mispriced assets – APT can help identify assets that are mispriced relative to their factor exposures.
- Customization – Traders can adapt the model to include factors they believe are most relevant to their specific markets or strategies.
- Performance attribution – APT can be used to understand the sources of a portfolio’s returns.
- Risk management – By understanding an asset’s factor sensitivities, traders can better manage and hedge their risk exposures.
APT requires more inputs and is more complex than CAPM and its flexibility and multi-factor approach can help provide a framework for more customized trading strategies.
Information Theory in Finance
Overview
Information Theory in Finance applies concepts from Claude Shannon’s information theory to understand market efficiency, price discovery, and information flow in financial markets.
This framework provides a quantitative approach to measuring and analyzing the role of information in financial decision-making and market dynamics.
Key Aspects
- Entropy – Measures the uncertainty or randomness in a system, applied to price movements or market states.
- Mutual Information – Quantifies the amount of information shared between different financial variables or time series.
- Information Flow – Analyzes how information propagates through markets and affects prices.
- Efficient Market Hypothesis – Relates to how quickly and accurately markets incorporate new information.
- Signal-to-Noise Ratio – Helps in distinguishing meaningful market signals from random fluctuations.
- Information Asymmetry – Quantifies differences in information possession among market participants.
Value for Traders
Information Theory in Finance offers several insights for traders:
- Improved market analysis – Helps in potentially quantifying market inefficiencies and potential trading opportunities.
- Better understanding of price movements – Analyzes the information content of price changes.
- Optimized information usage – Helps in determining the value of different information sources and strategies. What information sources and data are most important and how do they affect decision-making?
- Improved algorithmic trading – Informs the design of trading algorithms that can better process and act on market information.
- Market microstructure insights – Provides a framework for understanding how information is incorporated into prices at a micro level.
Rational Expectations Theory
Overview
Rational Expectations Theory, introduced by John Muth and further developed by Robert Lucas, posits that economic agents’ predictions of the future value of economically relevant variables aren’t systematically wrong.
This theory suggests that individuals use all available information and economic theories to make decisions, and their expectations influence economic outcomes.
Key Aspects
- Unbiased predictions – On average, people’s expectations are correct, although individual predictions may be wrong.
- Efficient use of information – Economic agents are assumed to use all available relevant information in forming expectations.
- Model consistency – Expectations are consistent with the economic model being used.
- Policy ineffectiveness – Systematic monetary or fiscal policy may be ineffective if it’s anticipated by economic agents.
- Learning and adaptation – Agents learn from past mistakes and adapt their expectations accordingly.
- Forward-looking behavior – Economic decisions are based on expectations about the future.
Value for Traders
How can the Rational Expectations Theory traders?
- Market efficiency – Helps understand how quickly markets may incorporate new information.
- Anticipating market reactions – Encourages traders to think about how other market participants might react to new information.
- Long-term perspective – Emphasizes the importance of considering long-term economic fundamentals.
- Contrarian opportunities – Identifying situations where market expectations might be systematically biased can lead to profitable trades.
The theory’s assumptions of perfect rationality and information use may not always hold in reality, but understanding Rational Expectations Theory can help traders develop more sophisticated models of market behavior and improve their decision-making processes.
Incomplete Markets Theory
Overview
Incomplete Markets Theory, developed by economists like Michael Magill and Martine Quinzii, deals with situations where the number of tradable assets is insufficient to span all possible states of the world.
This framework acknowledges that in real-world financial markets, it’s often impossible to hedge against all potential risks.
Leads to important implications for asset pricing, risk management, and market efficiency.
Key Aspects
- Limited risk-sharing – Not all risks can be perfectly hedged or insured against.
- Non-unique equilibrium – Multiple equilibrium prices and allocations may exist.
- Constrained Pareto efficiency – Market outcomes may not be fully efficient due to missing markets.
- Endogenous incompleteness – Market incompleteness can arise from informational or institutional constraints.
- Is there sufficient demand – Sometimes products may not exist due to a lack of demand (e.g., general lack of future products for inflation-linked bonds relative to nominal bonds).
- Financial innovation – The introduction of new financial instruments can help complete markets.
- Idiosyncratic risk – Some risks remain uninsurable and affect asset prices.
Value for Traders
Incomplete Markets Theory offers several insights for traders:
- Risk management – Highlights the importance of managing unhedgeable risks.
- Product development – Suggests areas where new financial products could be valuable.
- Pricing models – Informs more realistic asset pricing models that account for market incompleteness.
- Portfolio construction – Emphasizes the need for diversification beyond traditional asset classes.
- Market inefficiencies – Helps explain persistent pricing anomalies and excess volatility.
Understanding Incomplete Markets Theory can help traders:
- identify unhedged risks in their portfolios
- recognize potential market inefficiencies, and
- develop strategies that account for the limitations of real-world financial markets
It also underscores the importance of financial innovation in improving market efficiency and risk management capabilities.
Extreme Value Theory
Overview
Extreme Value Theory (EVT) is a branch of statistics that deals with the modeling and analysis of rare events or extreme deviations from the median.
In finance, it’s useful for understanding and quantifying the risk of extreme market movements, such as crashes or booms.
It’s gotten traction since these events occur more frequently than would be expected under normal distribution assumptions.
Key Aspects
- Tail risk – Focuses on the behavior of the tails of probability distributions.
- Generalized Extreme Value (GEV) distribution – A family of continuous probability distributions developed to combine three simpler distributions.
- Peak Over Threshold (POT) method – Analyzes observations that exceed a certain high threshold.
- Return periods – Estimates how often extreme events are likely to occur.
- Dependence structures – Considers how extreme events in different variables may be related. In terms of mathematical structure, copulas may be used.
- Non-stationarity – Accounts for how the distribution of extreme events may change over time.
Value for Traders
Extreme Value Theory provides several benefits for traders:
- Improved risk management – Offers more accurate estimates of potential extreme losses.
- Stress testing – Provides a framework for designing more realistic stress scenarios.
- Derivative pricing – Helps in more accurate pricing of options and other derivatives, especially those with payoffs dependent on extreme events.
- Portfolio optimization – Allows for portfolio construction that better accounts for tail risks.
- Opportunity identification – Can help identify potential opportunities in mispriced tail risk.
With EVT, traders can gain a more nuanced understanding of market risks, particularly those associated with rare but high-impact events.
This can lead to better or more nuanced risk management strategies (e.g., recognizing that risk in markets goes beyond just volatility and into things like tail risk, among other matters) and potentially identify opportunities where the market may be mispricing extreme risk.
Market Microstructure Theory
Overview
Market Microstructure Theory focuses on the specific processes and outcomes of how exchange occurs in markets.
It looks at how the actual mechanics of trading processes affect the formation of prices, liquidity, transaction costs, and various forms of market friction.
This theory is most relevant in understanding high-frequency trading, order flow, and the impact of market design on trading behavior and outcomes.
Key Aspects
- Order flow – Analyzes the process of how trading orders arrive in the market.
- Bid-ask spread – Examines the difference between the highest price a buyer is willing to pay and the lowest price a seller is willing to accept.
- Market depth – Considers the volume of orders at different price levels.
- Price discovery – Studies how new information is incorporated into asset prices.
- Market impact – Analyzes how individual trades affect market prices.
- Liquidity – Examines factors that contribute to ease of trading without significant price changes.
- Market fragmentation – Explores the effects of trading across multiple venues or platforms.
- Informational asymmetry – Studies how differences in information among market participants affect trading behavior and outcomes.
Value for Traders
Market Microstructure Theory offers several insights for traders:
- Execution strategies – Helps in developing optimal order execution strategies to minimize market impact and trading costs.
- High-frequency trading – Provides a framework for understanding and developing high-frequency trading strategies.
- Liquidity analysis – Offers tools for assessing market liquidity and its impact on trading.
- Order book analysis – Enables more sophisticated interpretation of order book data.
- Transaction cost analysis – Helps in understanding and minimizing various components of trading costs.
- Market making – Provides insights for market-making strategies and understanding market maker behavior.
- Venue selection – Helps in choosing optimal trading venues based on their microstructure characteristics.
- Regulatory impact assessment – Helps in understanding how changes in market regulations might affect trading conditions and strategies.
- Algorithmic trading design – Informs the development of trading algorithms that can effectively navigate various market microstructure considerations to generate profits. HFT is most commonly the type of strategy that focuses heavily on market microstructure.
Market Microstructure Theory helps traders gain a more nuanced view of how prices are formed at the most granular level.
This can lead to more efficient trading strategies, better risk management, and the ability to identify short-term trading opportunities that come from market microstructure effects.
Bayesian Methods
Bayesian methods provide a framework for studying markets by allowing us to update our beliefs and models as new information becomes available.
This approach is common in finance and economics, where uncertainty is pervasive and new data constantly emerges.
Let’s look at how Bayesian methods can be applied to market analysis:
Bayesian Methods
Bayesian methods are based on Bayes’ theorem, which describes the probability of an event based on prior knowledge and new evidence.
In market analysis, this allows us to combine our prior beliefs about market behavior with new data to form updated posterior beliefs.
For example, we might have a prior belief about the distribution of stock returns.
As we observe actual market data, we can update this belief to better reflect reality.
This process of updating beliefs is at the core of Bayesian thinking.
Bayesian Inference
Bayesian inference is the process of using Bayes’ theorem to update the probability for a hypothesis as more evidence becomes available.
In market analysis, this can be used for:
- Asset pricing – Updating our beliefs about the fair value of an asset based on new market information.
- Risk assessment – Refining our estimates of market risk as we observe market volatility.
- Trend prediction – Updating our beliefs about market trends as new economic data is released.
Non-parametric Bayesian Methods
Non-parametric Bayesian methods are useful when we don’t want to make strong assumptions about the underlying distribution of our data.
This is often the case in financial markets, where returns may not follow simple parametric distributions.
Some applications in market analysis include:
- Density estimation – Estimating the probability density function of asset returns without assuming a specific distribution.
- Clustering – Grouping similar financial instruments or market regimes without pre-specifying the number of clusters.
- Time series analysis – Modeling complex, non-linear relationships in market data.
For example, Dirichlet process mixture models can be used to cluster stocks based on their return characteristics without specifying the number of clusters in advance.
Variational Bayesian Methods
Variational Bayesian methods provide approximate inference techniques for complex Bayesian models. They’re particularly useful when dealing with large datasets or complex models where exact inference is computationally intractable.
In market analysis, variational methods can be applied to:
- Portfolio optimization – Approximating the posterior distribution of optimal portfolio weights, such as a portfolio made up of stocks, bonds, and commodities.
- Factor models – Inferring latent factors driving market returns in high-dimensional datasets.
- Volatility modeling – Estimating time-varying volatility in financial time series.
- Risk Management – Estimating the distribution of potential losses in financial portfolios.
- Algorithmic Trading – Inferring market conditions for developing adaptive trading strategies.
- Credit Scoring – Approximating the likelihood of default based on borrower characteristics.
For instance, variational methods can be used to approximate the posterior distribution in stochastic volatility models, allowing for efficient inference of market volatility dynamics.
Bayesian methods offer several advantages in studying markets:
- Handling unknowns – They provide a natural framework for quantifying and updating uncertainty.
- Incorporating prior knowledge – Allowing analysts to include domain expertise in their models.
- Sequential updating – Easily incorporating new data as it becomes available.
- Model comparison – Providing a consistent framework for comparing different models.
Bayesian methods nonetheless also have some challenges:
- Prior specification – The choice of prior can significantly impact results, especially with limited data.
- Computational complexity – Some Bayesian models can be computationally intensive, particularly for large datasets.
- Interpretation – Posterior distributions can sometimes be difficult to interpret, especially for non-technical stakeholders.
Despite these challenges, Bayesian methods continue to gain popularity in market analysis due to their flexibility and ability to handle complex, uncertain environments.
As computational power increases and new algorithms are developed, we can expect to see even more applications of Bayesian techniques in financial modeling and market analysis.
Conclusion
These frameworks for understanding financial markets offer diverse perspectives on market behavior.
Each has its own strengths and limitations.
For traders, the value lies not in adhering strictly to any single framework, but in synthesizing insights from multiple approaches to develop a nuanced understanding of markets and what drives them.
Key takeaways for traders across these frameworks include:
- Markets are complex, adaptive systems with inherent unpredictability.
- Psychological factors are important in market behavior because humans ultimately make the decisions or are designing the systems that do.
- Market environments and effective strategies evolve over time.
- Risk management is paramount given the inherent unknowns in markets and what simply can’t be known not matter how much data is taken in and what analysis is done.
- Understanding interconnections between market participants is important.
- Different timeframes and trader groups interact to create market dynamics.
- Extreme events and non-linear relationships are important features of markets.
Understanding these various frameworks can help traders develop better strategies, improve risk management, and gain a deeper understanding of markets.