Financial Markets as Level 2 Chaotic Systems
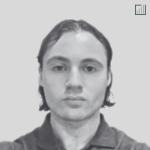
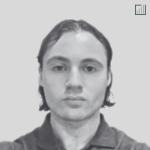
Financial markets are complex systems that have long fascinated economists, mathematicians, and market participants.
There are many conceptual frameworks and mathematical approaches to understanding financial markets.
One of the frameworks for understanding these markets is through the lens of chaos theory, particularly the concept of level 2 chaotic systems.
This approach helps us understand conceptually into why financial markets are notoriously difficult to predict and why traditional forecasting methods often fall short.
Key Takeaways – Financial Markets as Level 2 Chaotic Systems
- Market predictions can become self-fulfilling or self-defeating, as traders react to forecasts and alter market behavior.
- This feedback loop makes perfect prediction impossible.
- Small events can trigger large market movements due to complex interactions between numerous factors.
- Traders should stay alert to seemingly minor news or shifts.
- Traditional forecasting models often fall short because markets don’t follow assumptions of rationality or normal distribution.
- Human behavior and emotions play a significant role in market movements, making purely mathematical models insufficient.
- Understanding psychology is valuable for traders.
- Risk management and diversification are essential in chaotic markets.
- Traders should focus on building resilience – e.g., diversification, risk balancing and other risk management practices – rather than seeking perfect predictions.
Defining Chaos Theory
Chaos theory is a branch of mathematics that deals with complex systems whose behavior is highly sensitive to initial conditions.
In chaotic systems, small changes in input can lead to drastically different outcomes, making long-term prediction extremely challenging.
The Two Levels of Chaos
To understand financial markets, it’s important to distinguish between two levels of chaotic systems:
- Level 1 Chaos = Systems that do not react to predictions about them
- Level 2 Chaos = Systems that react to predictions about them
Level 1 Chaotic Systems: The Weather Analogy
Characteristics of Level 1 Chaos
Level 1 chaotic systems are characterized by their indifference to predictions.
The classic example of a level 1 chaotic system is weather.
The Weatherman’s Dilemma
Weather systems don’t care about forecasts.
If a meteorologist predicts rain, it doesn’t influence whether it’ll actually rain or not.
The weather operates independently of human predictions and expectations.
Another way to put it is that the weather isn’t an adversarial market.
Improving Predictions in Level 1 Systems
While level 1 chaotic systems are complex, we can improve our ability to predict them by:
- Gathering more data
- Developing more sophisticated models
- Increasing computational power
As we incorporate more factors into our weather models and use more powerful computers, our weather forecasts become increasingly accurate – at least in the short term (i.e., the weather forecast over the next few hours is more likely to be accurate than the forecast 10 days from now)..
Level 2 Chaotic Systems: Financial Markets
Defining Level 2 Chaos
Level 2 chaotic systems are fundamentally different from their level 1 counterparts.
These systems react to predictions made about them.
This creates a feedback loop that alters the system’s behavior.
The Self-Fulfilling (or Self-Defeating) Prophecy
In financial markets, predictions can become self-fulfilling or self-defeating prophecies.
Take bank research as an example.
If a respected analyst predicts a stock will rise, traders might buy the stock, causing its price to increase.
Conversely, if many predict a stock will do poorly, just the act of saying that could cause it to fall.
The Paradox of Perfect Prediction
Consider a hypothetical scenario:
- What if we could develop a computer program that predicts the S&P 500 with 100% accuracy?
The moment such a prediction is made public, market participants would react to this information, immediately altering the price of the index.
This reaction would invalidate the original prediction, creating a paradox.
This paradox illustrates why perfect prediction is impossible in level 2 chaotic systems.
The act of prediction itself becomes a factor that influences the system’s behavior.
Complexity and Sensitivity in Financial Markets
The Butterfly Effect in Finance
The concept of the “butterfly effect” – e.g., the metaphorical thought experiment of how a butterfly flapping its wings in Brazil could set off a tornado in Texas – is relevant in financial markets.
Small, seemingly insignificant events can trigger larger-scale market movements.
A statement from a prominent CEO praising a little-known technology can lead to a surge in investor interest, driving up its price significantly.
This initial price spike can attract more attention, leading to widespread media coverage and further investment.
The sudden influx of capital into the particular investment or investments can then trigger broader market movements, affecting related assets and causing ripple effects across the entire financial market.
Factors Influencing Market Behavior
Financial markets are influenced by an enormous number of factors, including (but not limited to):
- Economic data and indicators
- Political events
- Technological advancements
- Natural disasters
- Trader psychology
- Regulatory changes
- Global trade dynamics
The Challenge of Interaction
Not only are there numerous factors at play, but the way these factors interact is also highly complex.
There are many interdependencies and things dependent on other things.
The strength of each factor and its relationships with others can vary over time, making it extremely difficult to model or predict market behavior accurately.
The Limitations of Traditional Forecasting Methods
Why Traditional Models Fall Short
Traditional financial models often rely on assumptions of rationality, efficiency, and normal distribution of returns.
However, these assumptions often break down in real-world markets, especially during periods of crisis or rapid change.
The Problem with Historical Data
History helps inform us of what’s happened in the past, how the mechanics of certain situations plays out or can play out, and is important for practices like backtesting.
But past performance isn’t always indicative of future results.
In level 2 chaotic systems, the relationships between variables can change over time, so historical patterns may be less reliable.
The Role of Human Behavior
Human behavior, with all its irrationality and unpredictability, is important in financial markets because ultimately it’s human behavior that causes buying and selling – or human-created technologies and algorithms that do it.
Emotions like fear and greed can drive market movements in ways that are difficult to model mathematically.
Implications for Traders, Analysts, and Policymakers
The Illusion of Control
Understanding financial markets as level 2 chaotic systems challenges the notion that we can exert significant control over market outcomes.
We often want things to be deterministic or have overly simplistic reasons for doing what they’re doing (e.g., “the economy is bad”) when that’s simply inconsistent with reality.
This realization has important implications for both individual traders and policymakers.
Risk Management in a Chaotic Environment
Given the inherent unpredictability of financial markets, risk management is important.
Diversification, stress testing, and scenario analysis take on increased importance.
Understand signal vs. noise.
Not every wiggle is important.
The Need for Adaptive Strategies
In a level 2 chaotic system, static strategies are likely to become obsolete quickly.
Successful traders, investors, and policymakers need to develop adaptive strategies that can respond to changing markets and new information.
Thinking Probabilistically
Understanding financial markets requires thinking probabilistically.
There’s inherent uncertainty and randomness, which can help avoid overconfidence and improve our ability to design strategies to help us deal with this reality.
For most, it’s better to be more strategic than tactical.
Think of what a great asset allocation might look like.
Trading tactically is also valid, but doing it within the context of structure is what successful traders are often doing.
For example, if they have a portfolio that 40% in stocks, 45% in bonds, and 15% in precious metals and commodities, if they want to tactically trade stocks it’s done within the context of their stock allocation not getting significantly above or below that threshold.
Other Types of Level 2 Systems Outside Financial Markets
Financial markets are an often-cited example of Level 2 systems, but similar dynamics can be found in other areas of social life, from elections to traffic patterns.
Below, we look at various examples of Level 2 systems outside of financial markets, how predictions shape outcomes, and how human behavior is inherently tied to this complexity.
Social Media Trends
Social media trends are a prime example of a Level 2 system.
Platforms like Twitter/X, Instagram, and TikTok thrive on viral content, and predictions about what will go viral can heavily influence user behavior.
If a piece of content is predicted to trend or be widely shared, users are more likely to engage with it, often sharing or liking the content because they anticipate it will be popular.
This creates a feedback loop where the very prediction of virality contributes to making it viral.
For instance, influencers, marketers, or social media algorithms might project that a certain meme or video will take off.
As users see this content gaining traction, they reinforce the trend by sharing it with their own networks, causing a compounding effect.
This process highlights how human behavior, driven by expectations of social approval or relevance, directly shapes the outcome, and makes the prediction itself a component of the system’s dynamics.
Elections
Elections are another classical example of a Level 2 system.
Polls, media coverage, and political forecasts are important in shaping voter expectations and behavior.
For example, if a poll shows a particular candidate leading by a wide margin, it might discourage supporters of the trailing candidate from voting, thinking that their vote won’t make a difference.
Conversely, supporters of the leading candidate might become complacent and also decide not to vote, leading to a closer race than anticipated.
This dynamic introduces the idea of a self-fulfilling prophecy, where predictions about voter behavior and election outcomes affect how individuals act.
People are influenced by their perceptions of who is likely to win, and those perceptions, in turn, alter voter turnout and behavior, potentially making the prediction more or less accurate.
A similar dynamic occurs when voters believe that their preferred candidate is an underdog.
They might be more motivated to vote and rally others to vote, thus altering the election’s outcome based on initial expectations.
Traffic Patterns
Real-time traffic prediction systems, like Waze or Google Maps, are another really good illustration of a Level 2 system.
These systems collect data on traffic conditions and suggest alternative routes to help drivers avoid congested areas.
However, as more drivers follow these recommendations, the congestion can shift to other areas, potentially alleviating or exacerbating traffic in unexpected ways.
In this way, the prediction becomes part of the system itself: by influencing drivers’ decisions, it changes the very traffic patterns it set out to predict.
For example, if a traffic app predicts that a certain highway will be congested and recommends a side road instead, enough drivers might follow that advice to cause congestion on the side road.
This shifts the traffic problem from one area to another, demonstrating how the predictive system interacts with user behavior.
This is also why markets don’t react in ways that we often expect because others have similar ideas.
Similarly, if the app correctly predicts congestion and enough people alter their routes, it may alleviate the problem, but not in a way that was directly anticipated.
Pandemics
Pandemic responses are tied to Level 2 system dynamics.
Predictions about the spread of a disease can materially influence public behavior, which in turn impacts the actual course of the pandemic.
For instance, during the COVID-19 pandemic, early models predicting widespread transmission led to public health campaigns encouraging mask-wearing, social distancing, and lockdowns.
These behavioral changes, driven by the predicted outcomes, altered the actual spread of the virus.
In some cases, if enough people adopt preventive behaviors, the spread of the disease can be reduced, meaning that the dire predictions about exponential transmission may not come to pass.
This creates a feedback loop: the prediction leads to actions that influence the system (the pandemic), potentially making the prediction less accurate.
On the flip side, if predictions are dismissed or ignored, the virus might spread more rapidly, making the prediction accurate.
This demonstrates how human behavior, shaped by expectations and predictions, is important in determining the outcome of such a crisis.
Economic Bubbles
Economic bubbles offer another example of a Level 2 system.
In markets like housing or cryptocurrency, predictions of rising prices can lead to speculative buying, creating a self-fulfilling cycle where prices do indeed rise – at least temporarily.
For instance, if investors believe that a particular asset class (like housing or a specific cryptocurrency) is going to appreciate, they may rush to buy it, driving up demand and, thus, prices.
This speculative behavior can inflate a bubble, where asset prices rise far beyond their intrinsic value.
Eventually, when the bubble bursts – often because reality catches up with inflated expectations – prices crash, leading to significant financial losses for many participants.
Here, the key factor is that the prediction itself fuels the market behavior, demonstrating how expectations and outcomes are interwoven in Level 2 systems.
In the case of bubbles, the feedback loop can be particularly damaging, as it not only drives prices higher but also magnifies the eventual crash.
Social Movements
Social movements are another domain where predictions about success or failure can influence outcomes.
If a movement is perceived as gaining momentum, more people may be inclined to join, fueling its growth.
Conversely, if a movement is seen as unlikely to succeed, potential supporters may stay on the sidelines, which can stunt its development.
This dynamic is evident in various social and political movements throughout history, where early success can attract more supporters and resources, creating a snowball effect.
For instance, a protest movement might start small, but if media outlets and observers predict that it could grow into something larger, more people may be inspired to participate.
This, in turn, increases the movement’s visibility and influence, validating the initial predictions.
On the other hand, if predictions suggest that a movement is doomed to fail, potential supporters might withdraw their involvement, reducing the movement’s chances of success and turning the prediction into a self-fulfilling prophecy.
Emerging Approaches to Market Analysis
Machine Learning and AI
Machine learning algorithms and artificial intelligence offer new ways to analyze market data and identify patterns.
While these tools can’t predict the future with certainty, they can help in processing vast amounts of information and identifying potential trends.
Data dependency is still key.
The markets for a non-data dependent trader are very difficult in the long run (and perhaps the short run as well).
But the implication is also, of course, that AI and machine learning will never “solve” markets.
Behavioral Finance
The field of behavioral finance, which incorporates learning from psychology into financial analysis, provides a framework for understanding the irrational aspects of market behavior.
Complex Systems Theory
Approaches from complex systems theory, including agent-based modeling and network analysis, offer new ways to understand market dynamics and potential systemic risks.
For example, we’ve discussed in other articles that market movements are ultimately determined by who’s selling and who’s buying and for what reasons.
This can better be understood by taking the range of buyers and sellers in each market, understanding how big they are, and knowing what motivates or causes them to make the decisions that they make.
The Future of Financial Markets and Prediction
The Ongoing Quest for Better Models
Despite the challenges posed by level 2 chaos, the quest for better market models continues.
Researchers are constantly developing new approaches that try to capture more of the complexity inherent in financial markets.
The Role of Technology
Advancements in technology, including quantum computing and big data analytics, may provide new ways of analyzing and understanding market behavior.
Embracing Unknowns
Perhaps the most important lesson from understanding financial markets as level 2 chaotic systems is the need to understand that the range of unknowns is always going to be greater than the range of knowns relative to what’s discounted in the price.
Rather than seeking perfect prediction, market participants may need to focus on building resilience and adaptability.
Probabilities of Probabilities
Understand the concept of probabilities of probabilities – i.e., that while we have to think probabilistically about outcomes, even the probabilities themselves are not known.
Diversification and risk management are the key pillars.
Conclusion
The primary distinction between Level 1 and Level 2 chaotic systems lies in whether predictions about the system’s behavior feedback into the system, altering its trajectory.
In Level 1 systems, such as physical processes or natural phenomena, predictions do not influence outcomes.
A meteorologist’s forecast of a storm will not change the storm’s path, just as a physicist’s calculation of a satellite’s orbit won’t change its course.
These systems are driven by cause-and-effect relationships that remain unaffected by predictions or human expectations.
In contrast, Level 2 systems, often found in social and economic contexts, are characterized by feedback loops where predictions themselves become part of the system.
Financial markets are an archetypal Level 2 system, where the act of prediction and expectations – whether through analysis, media coverage, or investor behavior – alters market dynamics.
Trading in such systems isn’t just about understanding the current state of affairs but also anticipating how others will react to predictions and how those reactions will further influence the market.
This makes Level 2 systems inherently more unpredictable, as they are constantly influenced by human decision-making, expectations, and behavior.
In financial markets, for example, one trader’s gain is often another’s loss (in terms of alpha generation), making the system adversarial in nature.
This complexity explains why only a small fraction of market participants consistently outperform, while most struggle to beat the market.
Summary
Level 2 systems represent a fascinating intersection of prediction, behavior, and feedback loops.
Whether in social media, elections, traffic, pandemics, economic bubbles, or social movements, predictions can shape outcomes by influencing how people behave.
These systems highlight the relationship between human expectations and reality, creating a dynamic where outcomes are not fixed but evolve in response to the very predictions made about them.
Financial markets, as level 2 chaotic systems, have unique challenges and opportunities.
They will never be solved in the same way our weather forecasts have gotten increasingly accurate (as a level 1 chaotic system).
Perfect prediction remains an impossibility, but understanding the nature of these systems can lead to more effective strategies for navigating them.
Recognizing the limitations of traditional forecasting methods and embracing new approaches that account for the reactive nature of markets enables us to not be overconfident.
And, accordingly, traders, investors, policymakers, and researchers can develop more nuanced and effective ways of engaging with financial systems.
Ultimately, the study of financial markets as level 2 chaotic systems reminds us of the inherent unpredictability of complex, human-driven systems.
It encourages a shift from a paradigm of control – which the human brain wants to have but simply can’t in a complex system of financial markets – to one of adaptability, resilience, and continuous learning.
In doing so, it offers a more realistic and potentially more fruitful approach to understanding and participating in the markets.