Backtesting
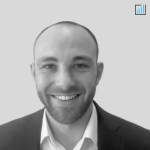
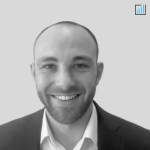
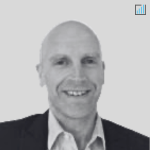
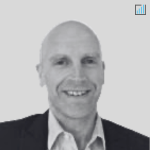
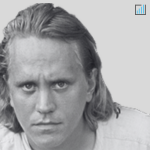
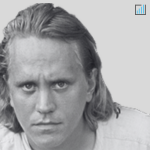
Backtesting is a method for assessing the validity of a trading strategy by using historical data to see how an asset (or portfolio of assets) would have performed in past periods. If results were successful, it might encourage traders to use that strategy going forward.
Top Brokers For Strategy Backtesting
-
1FOREX.comActive Trader Program With A 15% Reduction In Costs
Ratings
$1000.01 Lots1:50NFA, CFTCForex, Stocks, Futures, Futures OptionsMT4, MT5, TradingView, eSignal, AutoChartist, TradingCentralWire Transfer, Credit Card, Debit Card, Visa, Mastercard, Skrill, Neteller, ACH TransferUSD, EUR, GBP, CAD, AUD, JPY, CHF, PLN -
2xChief$100 No Deposit Bonus
Ratings
$100.01 Lots1:1000ASICCFDs, Forex, Metals, Commodities, Stocks, IndicesMT4, MT5Wire Transfer, Bitcoin Payments, Credit Card, UnionPay, Skrill, Neteller, WebMoney, Perfect Money, Debit Card, Ethereum Payments, FasaPay, VoletUSD, EUR, GBP, JPY, CHF -
3Plexytrade120% Cash Welcome Bonus
Ratings
$500.011:2000CFDs, Forex, Indices, Stocks, Commodities, CryptoMT4, MT5Bitcoin Payments, Ethereum PaymentsUSD, EUR -
4Coinexx100% Deposit Welcome Bonus
Ratings
0.001 BTC0.01 Lots1:500Forex, Commodities, Indices, CryptocurrenciesMT4, MT5Bitcoin Payments, Ethereum PaymentsUSD
Backtesting Theory
The underlying theory is that any strategy that worked well in the past is likely to work well in the future, and conversely, any strategy that performed poorly in the past is likely to perform poorly in the future.
But is this true?
In many cases, back-tested strategies fail once applied to the real world, as the sudden collapse of LTCM graphically illustrated. This could be due a number of factors, but the most common are dependence on correlations that disappear or biases in the back-testing process.
Examples of the pitfalls of back-testing include;
- There are as many potential investment models as there are ideas that can be quantified. The temptation therefore, is to devise a trading model that generates the maximum possible returns for a given (chosen) period. This is known as curve-fitting (or data mining), where an analyst creates a portfolio strategy that optimises returns during the period being studied, but which may not translate into superior returns during other, out-of-sample [1] periods. This is because they rely on the continuation of past correlations between the behaviour of assets or asset classes, which do not remain stable over time. A consistent relationship between back-testing, out-of-sample and forward performance testing (e.g. “paper trading”) can reduce the risk of generating misleading results, but generally, the more complicated the investment criteria the worse the performance of a back-tested system in the real world.
- Survivorship Bias, where the returns generated fail to consider the possibility that some firms go bankrupt over time; those that survive thus inevitably have above-average returns, relative to all companies in the sample. Those that do not are excluded from the future dataset and thus from the simulations, thus overstating the potential returns being generated by the investment process.
These problems do not make back-testing useless, but like many things related to markets, they should not be relied on exclusively.
Market risk cannot be measured in an objective way as it is not directly observable, being only inferred from variables that can be directly measured (Value-at-Risk, Probabilities e.g. confidence intervals etc.) Ultimately, there is no substitute for “live” trading, as it incorporates the real-world pressures and biases involved in actual trading.
[1] That is, those investment horizons not originally used to generate the strategy. A 10-year dataset might be used to construct a trading system, but to be useful it would need to have demonstrated its viability in periods other than that 10-year horizon. The data outside of that 10-year period would be out-of-sample data.