Why AI & Machine Learning Will Never Solve the Markets
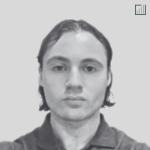
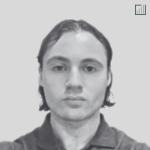
Artificial intelligence (AI) and machine learning (ML) have been touted as powerful tools for analyzing financial markets, with the potential to revolutionize the way traders/investors make investment decisions.
While AI and ML have shown great promise in some areas, there are inherent limitations that make it unlikely that they will ever be able to fully solve something as complex and dynamic as the financial markets.
Key Takeaways – Why AI & Machine Learning Will Never Solve the Markets
- Financial markets are open systems, making it difficult for AI and ML algorithms to fully predict market movements due to the complexity of factors and the influence of market participants.
- AI and ML algorithms often rely on historical data, which may not capture real-time market conditions accurately, and financial data can be noisy and subject to errors and biases.
- Market inefficiencies and the dynamic nature of financial markets pose challenges for AI and ML algorithms in accurately predicting market behavior.
- However, AI decision-making that focuses on cause-effect relationships can provide more accurate predictions by understanding the underlying drivers of market behavior.
- We also discuss how the development of LLMs can influence the understanding of markets and how combining the creativity of LLMs with the accuracy and precision of statistical methods can create a path to greater understanding at scale.
Open Systems and the Challenges for AI and Machine Learning
One of the key reasons for this is that financial markets are “open systems”.
This means that they are complex and dynamic, with many different factors influencing market movements at any given time.
These factors can include economic indicators, political events, natural disasters, corporate news, and many others.
In addition, the actions of market participants, such as traders, investors, and speculators, can also have a significant impact on market behavior, which can’t be predicted with accuracy.
The complexity of financial markets means that it is difficult, if not impossible, for any single model or algorithm to accurately predict market movements with any degree of certainty.
Reliance on historical data
While AI and ML can help to identify patterns and trends in market data, they are limited by the fact that they often rely on historical data to make predictions.
This means that they may not be able to fully capture the nuances and complexities of real-time market conditions, which can change rapidly and unpredictably.
Data quality
Another challenge for AI and ML in financial markets is the issue of data quality.
Financial data is often noisy, incomplete, and subject to errors and biases.
This can make it difficult for AI and ML algorithms to accurately identify meaningful patterns and trends in the data.
In addition, financial markets are subject to frequent changes in regulations, market structure, and other factors, which can also impact the quality and availability of data.
Moreover, when something gets programmed into systems widely it can also affect future data.
Market inefficiencies
There is also the issue of market inefficiencies, such as a lack of liquidity.
Financial markets are not always efficient, meaning that market prices do not always accurately reflect the true value of a security or asset.
This can create opportunities for traders and investors to exploit market inefficiencies, but it also makes it difficult for AI and ML algorithms to accurately predict market movements.
Comparisons with Closed Systems
Open systems like financial markets are significantly more challenging to model with AI and machine learning than closed systems, such as chess.
In a closed system like chess, the rules are well-defined, and there are a finite number of possible moves and outcomes. Even if the total number is gargantuan, with enough computing power it is theoretically solvable.
This makes it easier to develop AI models that can analyze past games, learn from them, and predict optimal moves in new games.
On the other hand, financial markets are open systems characterized by dynamic and complex interactions between multiple participants with different objectives, sizes, strategies, and decision-making processes.
The data generated by financial markets is vast and unstructured, with many variables and factors that can influence prices and outcomes.
This makes it challenging to develop AI models that can accurately capture all the variables that go into financial market pricing and make reliable predictions.
Furthermore, financial markets are subject to significant changes, such as policy shifts, geopolitical events, and technological innovations, that can affect market behavior and render past data flawed or irrelevant.
Therefore, AI models need to be adaptive and continuously updated to remain relevant and effective in modeling financial markets.
AI Decision-Making That Focuses on Cause-Effect Relationships
AI decision-making that focuses on cause-effect relationships in financial markets can produce better results than focusing solely on past data because it seeks to understand the underlying drivers of market behavior.
By identifying the root causes of market movements, AI systems can make more accurate predictions about future market trends and behavior.
Focusing solely on past data can be problematic because it assumes that historical patterns will continue to repeat themselves in the future.
However, markets are dynamic and subject to a wide range of influences that can cause patterns to shift and change over time.
This means that relying solely on past data can lead to inaccurate predictions and poor trading/investment decisions.
By contrast, an AI system that focuses on cause-effect relationships seeks to understand the fundamental drivers of market behavior.
This involves analyzing a wide range of data sources, including economic indicators, market sentiment, geopolitical developments, and other factors that can influence market behavior.
By identifying the underlying causes of market movements, AI systems can make more accurate predictions about future market trends and behavior.
For example, an AI system that is trained to identify the causal relationship between interest rates and stock prices could use this information to make more accurate predictions about future market behavior.
We know that the price of anything is the money and credit spent on it divided by the quantity.
So if market participants have data on who the buyers and sellers are, how big they are, and what their motivations are, they can gain an understanding of how they’re likely to react when certain variables change (e.g., growth, inflation, regulatory, etc.).
By understanding the impact of interest rate changes on corporate earnings, consumer spending, and other economic factors, the AI system can make more informed investment decisions.
AI decision-making that focuses on cause-effect relationships can also be more flexible and adaptable than traditional investment strategies.
Because AI systems are constantly learning and updating their models, they can quickly adapt to changing market conditions and identify new cause-effect relationships that may not have been previously recognized.
Of course, it’s important to note that AI decision-making is only as good as the data it is based on and the algorithms used to analyze that data.
In order for AI systems to produce accurate results, they must be properly trained and validated on high-quality data.
Additionally, human oversight and input is essential to ensure that the AI system is making decisions that are in line with the goals and values of the organization.
The Future of ‘Thinking Systems’ in Financial Trading
Benefits
Thinking systems have the potential to revolutionize the way trading is conducted by allowing for faster, more accurate analysis of market data, and the ability to identify and act upon patterns that may be missed by human traders.
One of the most significant advantages of thinking systems in financial market trading is their ability to process vast amounts of data quickly and efficiently. And to do it less emotionally, as they don’t care if the decisions they make are popular or not.
This can allow traders to make decisions based on real-time data that may not have been possible in the past.
Additionally, thinking systems can help identify market trends and patterns that may be difficult or impossible for humans to spot.
Limitations
As we mentioned, there are also limitations to thinking systems in financial market trading.
One major limitation is the risk of over-reliance on these systems, which can lead to a lack of human oversight and potential errors.
Additionally, thinking systems can be vulnerable to bias and may not account for all relevant factors when making trading decisions.
In cases of machine learning where the computer is fed with data, are the algorithms robust or are they simply data-mined gibberish that isn’t causal in any way?
As a result, it is essential for traders to be aware of these limitations and use thinking systems in conjunction with human expertise to make informed trading decisions.
Another limitation is the potential for these systems to be manipulated by malicious actors, such as cybercriminals, who may seek to exploit vulnerabilities in the systems or use them to spread false information and manipulate markets.
They will tend to struggle when it comes to anything requiring creative/original thinking or imagination because they are only as good as they’re trained.
For example, in the case of the popular chatbot ChatGPT, it tends to do well when it comes to information retrieval on historical events or other forms of static content.
But when it comes to various types of tasks, it struggles or is incapable because its breadth and depth of knowledge are weak, it isn’t able to reason or think critically in the same way a human can, its accuracy is often low, and its ability to think creatively is poor. It simply provides responses based on the data it’s been fed.
Lack of Data
Another problem in markets is a lack of data that makes a statistical approach (e.g., basic regressions or machine learning techniques) difficult to apply effectively.
Business cycles are normally 5-10 years each. Debt cycles (interest rates hitting zero and necessitating new policies, like 1929 and 2008) can take 50-100 years to play out. And most financial data doesn’t go back all that far.
Moreover, the game changes as participants learn. Algorithms can change markets, such that the history that preceded it became less and less relevant to the world we’re currently living in.
LLMs and Trading
LLMs have thus far shown to be relatively good at various tasks, such as scoring in the 80th to 90th percentile in various knowledge-based tasks (e.g., taking the LSAT, MCAT, and other tests).
But they’re only in those percentiles for a reason – they still make too many errors and often just make stuff up.
LLMs for picking stocks, for example, is basically useless. Or for predicting any economic or market phenomenon.
However, LLMs can theorize and can come up with creative ideas.
However, they are not good at actually determining whether those theories are good or not.
So, additional statistical techniques need to be overlaid on their reasoning that focus on precision and accuracy.
That back and forth combination can iterate to capture the good of each and help cancel out what they’re bad at to get at something that genuinely elevates human understanding in a way that’s useful and creates legitimate value. Not only in terms of financial market applications but in other fields as well.
Moreover, it enables doing these things at scale.
Even if an LLM “out of the box” has no value in creating unique, accurate insights about various phenomenon (e.g., where the stock market will go over the next five years), there’s still value in having an analyst who’s read everything there is to read about various subjects up to a certain cutoff point.
And can convey that knowledge back to you based on how it’s prompted.
Example: LLMs Understanding of Greed vs. Statistical Models Understanding of Greed
One example of the difference between LLMs and traditional statistical techniques would be the concept of “greed.”
LLMs understand greed because they’ve read most everything that has been written about greed in financial markets.
However, statistical models don’t particularly understand the concept well because they’re focused on the data of markets, not the labeling of the data.
With LLMs, they can think about statistical results in the context of the human condition that generates those results.
LLMs can help query statistical models so they can actually explain themselves, where humans can actually understand whether the rules embedded in them are accurate and valuable or not.
MIND-BLOWING USES OF AI IN FINANCE
FAQs – AI & Machine Learning in Financial Services
How is AI and machine learning used in financial markets?
AI and machine learning are used in financial markets to analyze vast amounts of data and identify patterns to inform investment decisions, risk management, fraud detection, and algorithmic trading.
AI-powered systems can learn from historical data, news articles, social media sentiment, and other relevant sources to make predictions and generate insights, such as identifying market trends, estimating asset values, and forecasting risk.
AI can also help traders identify anomalies or patterns in trading behavior that may indicate market manipulation or illegal activities, which could lead to better regulatory oversight.
Yes, AI can help in financial market trading. AI-powered trading algorithms can analyze vast amounts of data and identify patterns to inform investment decisions, execute trades, and manage risk.
By leveraging AI, traders can make faster and more informed decisions, react more quickly to market changes, and automate repetitive tasks.
Overall, AI has the potential to improve the efficiency and effectiveness of financial market trading.
What percentage of trades in financial markets are made by algorithms?
It is difficult to provide an exact percentage of trades in financial markets that are made by algorithms, as the use of algorithmic trading varies by market, asset class, and region.
There is also the presence of intermediaries in markets, such as market makers, who are there to help close bid-ask spreads rather than trade with the intent of influencing market direction or other factors.
However, algorithmic trading has been growing in popularity and usage throughout the years, particularly in high-frequency trading and quantitative investing.
However, it’s generally believed that about 60-80 percent of all trading volume in major equity markets globally is done through algorithms. This number continues to grow.
In other markets, such as foreign exchange and fixed income, the use of algorithms is also widespread, particularly for executing large or complex trades.
Overall, while there is no universal figure for the percentage of trades in financial markets that are made by algorithms, it is clear that algorithmic trading has become an increasingly important and prevalent tool for market participants.
Conclusion
While AI and ML have shown great promise in some areas of financial markets, there are inherent limitations that make it unlikely that they will ever be able to fully solve the financial markets.
Financial markets are complex and dynamic, and are subject to a wide range of factors that can influence market behavior.
AI and ML algorithms are often limited by the fact that they rely on historical data, are subject to data quality issues, and may not be able to fully capture market inefficiencies.
In contrast, closed systems like chess, for example, offer a more controlled and structured environment that is easier to model with AI.
AI decision-making that focuses on cause-effect relationships can produce better results than focusing solely on past data because it seeks to understand the underlying drivers of market behavior.
By identifying the root causes of market movements, AI systems can make more accurate predictions about future market trends and behavior, leading to better investment decisions.
Overall, it’s important to recognize the limitations of these systems and use them as a tool to supplement human expertise, rather than as a replacement for it.
On top of that, traders need to take steps to mitigate the risks associated with these systems, such as implementing robust security measures and conducting regular audits of the systems to ensure they’re working as intended.