Decision Theory: Applications in Trading & Investing
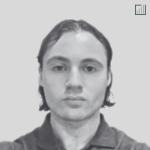
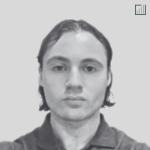
Decision theory, also known as the theory of choice, is a branch of applied probability theory and analytic philosophy that focuses on making decisions based on assigning probabilities to various factors and assigning numerical consequences to outcomes.
Decision theory encompasses three main branches:
- normative decision theory (focused on optimal decisions)
- prescriptive decision theory (describing observed behaviors with conceptual models), and
- descriptive decision theory (analyzing actual decision-making processes)
This theory is interdisciplinary, studied by various professionals from management scientists to computer scientists to traders and investors.
The quality of your decision ultimately determines your trading success, so it pays a lot to consider how we make them.
Key Takeaways – Decision Theory: Applications to Trading & Investing
- Decision Theory and Its Branches: Decision theory is about making choices by assigning probabilities to factors and numerical consequences to outcomes. It has three main branches:
- normative (optimal decisions)
- prescriptive (observed behaviors), and
- descriptive (actual decision processes)
- Application of Decision Theory in Finance: Concepts from decision theory are applied to finance, trading, and investing.
- For instance, Bayesian epistemology updates beliefs with new evidence, Bayesian statistics adjusts predictions based on data, and causal decision theory predicts cause-and-effect relationships.
- These tools can help understand market trends, optimize strategies, and predict events’ impacts.
- Challenges and Complexities of Decision-Making: Decision-making in finance involves complexities and biases.
- Psychological biases, like loss aversion and framing effects, can impact choices.
- Paradoxes like Newcomb’s Paradox challenge traditional decision-making models (expected value vs. strategic dominance).
- Financial professionals must balance risk and return, adapt to changing preferences, and consider various decision-making frameworks.
Let’s look into the applications of various concepts from decision theory to finance, trading, and investing:
Bayesian Epistemology
Bayesian epistemology is the study of using Bayesian probability to understand and analyze knowledge and beliefs.
In finance, it’s applied to update beliefs about market trends or asset values based on new evidence, allowing traders to refine their strategies.
Bayesian Statistics
Bayesian statistics involves updating probabilities based on new data.
In trading and investing, it’s used to adjust predictions about market movements or asset values as new data becomes available, optimizing decision-making processes.
Causal Decision Theory
Causal decision theory focuses on the cause-and-effect relationships of decisions.
Understanding these relationships can help investors predict the impact of specific events on asset prices or market movements.
Choice Modeling
Choice modeling aims to predict decisions an individual might make based on certain factors. This could help in the design of financial products or marketing strategies.
Constraint Satisfaction
Constraint satisfaction involves making decisions within certain constraints or limits.
In portfolio management, it refers to selecting assets that meet specific criteria set by the investor, like risk tolerance or desired returns.
Daniel Kahneman
Daniel Kahneman is a psychologist known for his work on behavioral economics.
His insights, especially regarding cognitive biases, have implications in finance, helping professionals understand irrational behaviors in markets.
We discuss his work on prospect theory later down the page.
Decision Making
Decision making is the process of choosing among alternatives.
In finance, it’s a constant activity, whether deciding on investment strategies, asset allocations, or trading tactics.
It also emphasizes the reality of relative choices.
Decision Quality
Decision quality refers to the effectiveness of a decision in achieving desired outcomes.
In trading/investing, it emphasizes not just returns, but also factors like risk management and long-term sustainability.
Emotional Choice Theory
This theory looks into how emotions influence decision-making.
In trading, understanding emotional biases can prevent rash decisions during volatile market conditions.
Related: Psychological Biases in Trading and How to Avoid Them
Evidential Decision Theory
Evidential decision theory bases decisions on the evidence available. It can guide investment choices by weighing current market data and trends.
Game Theory
Game theory studies strategic interactions among rational decision-makers.
In finance, it’s applied in scenarios like mergers and acquisitions, where entities act based on others’ potential actions.
One could also explore how certain game theory elements, like the prisoner’s dilemma, might influence geopolitics and the risk for financial portfolios.
Multi-criteria Decision Making
This involves making decisions based on multiple criteria.
Traders/investors often use this when considering factors like risk, return, and liquidity before making an investment.
Newcomb’s Paradox
Newcomb’s Paradox involves a game with two boxes:
- Box A contains $1,000.
- Box B either contains $1 million or nothing.
You can take both boxes or just Box B. A predictor, who’s almost always right, has already guessed your choice.
If they predicted you’d take both, they left Box B empty.
If they predicted you’d take only Box B, they put $1 million in it.
The player does not know what the predictor predicted or what box B contains while making the choice.
What’s the best choice?
The best choice in Newcomb’s Paradox is a matter of debate and depends on one’s philosophical stance:
- One-boxers argue that you should choose only Box B. Since the predictor is almost always right, the best way to maximize your money is to act in a way that makes it likely Box B contains the $1 million.
- Two-boxers argue that, regardless of the predictor’s decision, taking both boxes maximizes your money. If Box B already contains the $1 million, you get it plus the $1,000 from Box A. If it’s empty, you still get the $1,000.
There’s no definitive answer, and the paradox highlights tensions between causal decision theory and evidential decision theory.
Relevance of Newcomb’s Paradox to Finance, Trading, and Investing
In financial contexts, Newcomb’s Paradox challenges the expected utility mindset that many financial professionals rely on.
Here’s why:
Financial decisions are often based on the expected utility principle (expected value), which involves calculating the average or expected outcome of various choices.
In Newcomb’s Paradox, if we trust the predictor’s near-perfect accuracy, the expected utility of choosing only Box B is about $1,000,000 per game.
However, the strategic dominance principle, another pillar of game theory, suggests that one should choose an option that always yields a better outcome, regardless of other factors.
By this principle, choosing both boxes is superior since it always results in an additional $1,000 compared to just choosing Box B.
The paradox exposes a tension in financial decision-making.
While the expected utility mindset would advocate for the choice that statistically maximizes returns (Box B), the dominance principle would push for a strategy that always yields a marginally better outcome (both boxes).
This divergence highlights the complexities in financial decision-making and the need to consider both statistical outcomes and strategic advantages.
Operations Research
Operations research uses mathematical models to aid decision-making.
In finance, it can optimize trading strategies, portfolio allocations, and other financial operations.
Optimal Decision
An optimal decision maximizes the desired outcome.
In trading/investing, it refers to choices that offer the best returns for a given level of risk.
Preference
Preference refers to the ranking of outcomes based on desirability.
For example, for institutional traders/investors, understanding their investor preferences is important for product development and portfolio management.
Prospect Theory
Prospect theory, developed by Kahneman and Tversky, describes how people make decisions involving risk.
It suggests that individuals evaluate potential losses and gains relative to a reference point, often leading to irrational decisions.
Losses are felt more intensely than equivalent gains, causing risk-averse or risk-seeking behavior.
Quantum Cognition
Quantum cognition applies principles from quantum mechanics to cognitive processes.
Its implications in finance are still emerging, but it offers novel perspectives on decision-making in complex market environments.
Rational Choice Theory
This theory assumes individuals make decisions that maximize their benefits.
In finance, it’s used to predict market behaviors based on economic incentives.
Rationality
Rationality involves making logical and consistent decisions.
Rationality is often assumed in models, but real-world deviations (due to biases) can lead to market inefficiencies.
Secretary Problem
The secretary problem is a famous problem in probability theory and statistics about optimal stopping (i.e., when to make a decision).
In business, it can be likened to the challenge of how to hire well.
How many candidates should you interview, how do you discern between them, and when should you make a decision?
Signal Detection Theory
This theory deals with discerning signals from noise.
In trading, this means discerning genuine market trends amidst the daily fluctuations and noise, helping traders act on significant market signals.
Small-numbers Game
This refers to situations where decisions are based on small sample sizes.
In finance, it’s a cautionary concept, warning investors against making broad assumptions based on limited data.
Stochastic Dominance
Stochastic dominance is a form of order relation between random variables (investments).
It helps investors compare different investment opportunities based on their return distributions, aiding in risk assessment.
Trade-Off & Opportunity Cost
In finance, a trade-off involves balancing different factors, often risk and return.
Opportunity cost refers to the potential benefit lost when choosing one investment over another.
Essentially, opportunity cost is the return you forgo from the next best alternative when making a financial decision.
Two Envelopes Problem
The “two envelopes problem” is a paradox in probability and decision theory.
You’re given two envelopes, one containing twice as much money as the other.
Without knowing the amount in either, you pick one.
After seeing its contents, you’re given the option to switch.
Intuitively, it seems there’s no advantage to switching. However, a flawed mathematical argument suggests that the other envelope always offers a better expected value, tempting you to switch.
This creates a paradox, as the logic would apply even if you chose the other envelope initially, leading to an endless loop of switching.
While not directly applied in finance, it challenges traditional decision-making models and can inspire alternative thinking about risk and reward.
Expected Utility or Expected Value
Expected utility or expected value represents the average utility of an outcome, weighted by its probability.
In finance, it’s a foundational concept for many investment and pricing models, guiding decisions by weighing potential rewards against risks.
The main fault in expected utility is generally based on looking too closely at averages and not enough on outliers.
Example
For example, suppose you have the opportunity to play a game where you have a 90% chance of losing $1,000 and a 10% chance of winning $10,000.
Your expected value is: 0.90 * -$1,000 + 0.10 * $10,000 = +$100
However, your most likely outcome by quite a bit is losing $1,000.
So, to “correct” for this issue, you might want to:
- Ensure you can cover the loss if you do play this game
- Ensure a sufficient number of trials so that you’re almost sure you’ll have winning results over time
Time-inconsistent Utility Functions
This refers to situations where preferences change over time.
In finance, it can explain behaviors like short-term trading or the preference for immediate rewards over long-term gains.
In politics, it might involve policymakers choosing to have large deficits to pay for things now with unclear payoffs, which will lead to debt and issues down the road (that will fall into the hands of other policymakers).
Quasi-Hyperbolic Discounting
This concept describes how people often heavily discount future rewards in favor of smaller, immediate rewards.
In investing, it can explain why some might opt for lower short-term returns over potentially higher long-term gains.
Loss Functions
In finance, loss functions measure the difference between predicted and actual outcomes.
They’re important in model optimization, helping traders and investors refine their strategies.
Risk Functions
Risk functions evaluate the potential downsides or uncertainties of decisions.
In portfolio management, they’re used to assess the potential volatility or loss associated with investment choices.
Admissible Decision Rules
These are decision rules that are considered reasonable or acceptable.
In finance, they guide investment strategies by setting boundaries on acceptable risks and returns.
Antecedent Distributions
In Bayesian statistics, these are prior distributions.
They represent initial beliefs about parameters before new data is considered, aiding in predictive modeling.
Bayesian Procedures
These procedures update probabilities based on new evidence.
They’re used to refine predictions and strategies as new market data emerges.
It basically involves updating prior beliefs and making incremental changes rather than starting from scratch.
Minimax Procedures
Minimax aims to minimize the maximum possible loss.
It’s a strategy to safeguard against worst-case scenarios, especially in volatile markets.
This might involve holding options in a portfolio to lop off left-tail risk or better balancing a portfolio to reduce lumps in returns.
Decision Analysis: Maximax, Maximin, Minimax Regret
Allais Paradox
This paradox reveals inconsistencies in decision-making under risk.
It highlights that investors’ choices might not always align with expected utility theory, indicating behavioral biases.
Ellsberg Paradox
This paradox demonstrates aversion to ambiguity.
It can explain why investors might avoid assets or markets with unclear outcomes, even if the potential rewards are high.
Anchoring
Anchoring refers to the human tendency to rely heavily on the first piece of information encountered.
It can lead to biases, like holding onto a stock based on its initial price rather than its current value.
Intertemporal Choice
This involves decisions about when to consume or invest.
In finance, it’s central to decisions about saving versus spending or investing for short-term versus long-term gains.
Distinction Bias
Distinction bias occurs when comparing two options side by side, exaggerating the differences between them.
In investing, it might lead to overvaluing or undervaluing assets when compared directly.
Heuristics
Heuristics are mental shortcuts used to make decisions quickly.
They can lead to biases, potentially resulting in suboptimal investment choices.
For example, an investor might overvalue a company that markets itself as an “AI company” rather than deeply understanding how exactly it will drive earnings.
Gambler’s Fallacy
This is the belief that past events influence future probabilities.
In trading, it can lead to misguided expectations, like expecting a stock to rise because it has fallen repeatedly.
Possibility Theory
This theory deals with uncertain events.
It offers tools to evaluate trades or investments in situations where probabilities are not clearly defined.
Dempster-Shafer Theory
This theory is a mathematical framework for modeling uncertainty.
It can be used to assess the reliability of evidence when making investment decisions.
Info-Gap Decision Theory
This theory addresses decisions under severe uncertainty.
It can guide investment strategies in highly volatile or unpredictable markets.
Known Unknowns and Unknown Unknowns
This distinction refers to things we know we don’t know (known unknowns) and things we don’t even realize we don’t know (unknown unknowns).
In finance, it underscores the importance of risk management and the limitations of predictive models.
In financial modeling and forecasting, we just have distributions of expected outcomes. We don’t know anything for sure.
Probabilities of Probabilities
This concept deals with the meta-probability or the probability of a probability.
It’s the uncertainty about the underlying probability itself, often encountered in situations with limited data or inherent unpredictability.
In finance, especially in areas like options pricing or risk assessment, understanding not just the direct probabilities but also the uncertainties around those probabilities is important.
For instance, when assessing the risk of rare events, analysts might not only consider the event’s likelihood but also the confidence level of that likelihood estimate.
This layered approach to probability can lead to more robust financial models and strategies.
Ludic Fallacy
This fallacy involves basing decisions on simplified models that don’t account for real-world complexities.
In finance, it’s a caution against over-reliance on models that don’t consider certain criteria that are relevant.
Necktie Paradox
The Necktie Paradox is a thought experiment in probability theory.
It revolves around two people randomly choosing neckties for each other from a set, and the counterintuitive result that both can have a higher chance of choosing the same tie for the other than they do of choosing different ties.
While not directly applied in finance, the Necktie Paradox serves as a reminder of the counterintuitive results that can arise in probability and decision-making.
It underscores the importance of thorough analysis in financial modeling and the potential pitfalls of making assumptions without rigorous examination.
Bertrand’s Paradox
Bertrand’s Paradox is a problem within the classical interpretation of probability theory.
It presents a situation where different methods of calculating probabilities can lead to different results, emphasizing the importance of clear definitions and assumptions.
The paradox serves as a cautionary tale for financial analysts and modelers.
It emphasizes the need for clear assumptions and methodologies when calculating probabilities, especially in complex financial instruments or derivatives where different models might yield different valuations.
Siegel’s Paradox
Siegel’s paradox highlights a counterintuitive scenario.
When there’s uncertainty about future prices or exchange rates, rational consumers might be incentivized to temporarily trade their preferred goods or currency for non-preferred ones.
The intention behind this seemingly irrational behavior is to trade back to their preferred goods or currency once the future prices or exchange rates become clearer.
It challenges traditional notions of rational behavior and highlights potential inefficiencies or arbitrage opportunities in currency markets.
However, note that real-world factors like transaction costs, geopolitical events, and central bank interventions can influence these theoretical outcomes.
Conclusion
Decision theory offers a variety of concepts and tools that can be applied to finance, trading, and investing.
By understanding these concepts, professionals can make more informed and rational decisions, optimizing their strategies for better outcomes.