High Dimensionality in Finance
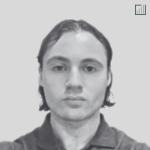
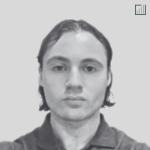
In the context of finance, the term “high-dimensional” refers to the characteristic of problems or models that involve a large number of variables or factors.
Financial markets are complex systems influenced by many elements and variables influencing them.
Many things are dependent on lots of other things.
Moreover, we have known unknowns (things we know we don’t know) and unknown unknowns (things we don’t know that we don’t know).
Incorporating these elements into financial models or analyses significantly increases the dimensionality of the problem.
High dimensionality poses unique challenges and opportunities in financial analysis and modeling.
Key Takeaways – High Dimensionality in Finance
- What High Dimensionality Is: High dimensionality in finance refers to the fact that there are a vast number of factors and variables at hand, influencing each other and the values of financial assets/instruments.
- This creates complex, multidimensional analytical and predictive challenges.
- Advanced Modeling: Requires more sophisticated models that can capture market dynamics and relationships between a larger number of assets.
- Computational Challenges: High dimensionality introduces computational complexity. Requires better algorithms and techniques for efficient data processing and analysis.
Large Number of Assets
In Portfolio Optimization
One prominent example of high dimensionality in finance is found in portfolio optimization.
This involves selecting the best mix of assets to maximize returns for a given level of risk or, conversely, to minimize risk for a given level of expected return (and higher-moment forms of this problem).
When dealing with a large number of assets, the complexity of the problem escalates rapidly as each asset adds a new dimension to the optimization problem.
This increase in dimensions exponentially grows the solution space.
This complicates the optimization process.
In Asset Allocation
Asset allocation decisions involve distributing investments across various asset classes, such as stocks, bonds, commodities, and real estate.
Each asset class, and further each individual asset within these classes, adds a dimension to the problem.
High dimensionality in this context arises from the need to consider the correlations and covariances among a large number of assets.
This is important for understanding the risk-return profile of the portfolio.
Multifaceted Economic, Social, and Political Factors
Financial markets are influenced by a variety of economic factors, including inflation, GDP growth, the cost of labor, and policy decisions.
There are also social factors and political factors (e.g., monetary and fiscal policy).
When building financial models, especially those intended for economic forecasting or macroeconomic analysis, all of these influences create multidimensionality.
High-dimensional models that include many such variables are more capable of capturing the complexities of real-world phenomena but are also more challenging to analyze and compute.
Technological and Market Developments
Growing Data Availability and Complexity
Advancements in technology and the proliferation of financial instruments have led to an explosion in the volume and variety of financial data.
This data includes market prices, trading volumes, news sentiment, social media influence, and more, each contributing to the dimensionality of financial analysis.
Algorithmic and High-Frequency Trading
Algorithmic and high-frequency trading adds another layer of complexity.
These trading strategies often involve analyzing large datasets to identify fleeting market opportunities.
This requires the processing and analysis of high-dimensional data in real-time.
Challenges and Solutions
Computational Complexity
One of the primary challenges of high dimensionality is the computational complexity it introduces.
Traditional analytical techniques often become impractical or ineffective as the number of dimensions increases.
Advanced Techniques
To extract insights from high-dimensional data and to make the computation tractable, financial analysts and quants employ techniques such as:
- machine learning
- dimensionality reduction methods (e.g., principal component analysis (PCA)), and
- more sophisticated optimization algorithms (e.g., heuristics, metaheuristics, gradient-based, nonparametric).
Conclusion
High dimensionality in finance is a reflection of the variety of factors influencing financial variables and financial markets.
Advanced analytical techniques, particularly those from the fields of machine learning and computational finance, are important for navigating and extracting value from high-dimensional financial problems.