What is Covariance? Applications in Trading & Portfolio Construction
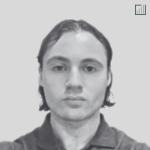
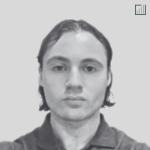
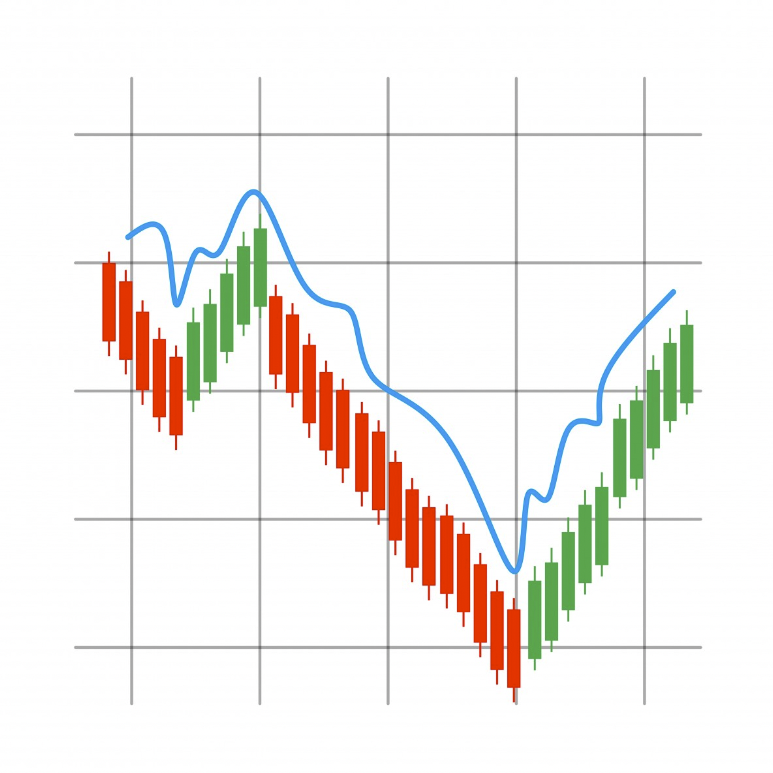
Covariance measures the directional relationship between two variables. Covariance is used in portfolio theory and modern portfolio theory.
Covariance is a statistical measure that calculates the degree to which two variables vary together. Covariance can be positive or negative, and it is typically represented by a covariance matrix.
Covariance is used in finance to measure the relationships between asset returns.
Covariance can be helpful in constructing efficient portfolios, as it can provide insight into which assets are likely to move in the same direction (positive covariance) or in opposite directions (negative covariance).
Modern portfolio theory uses covariance to help investors construct efficient portfolios that are diversified and have the potential to earn the highest return for a given level of risk.
Covariance is a statistical measure that calculates the degree to which two variables vary together.
Covariance can be positive or negative, and it is typically represented by a covariance matrix.
Understanding Covariance
Modern portfolio theory uses covariance to help investors construct efficient portfolios that are well-diversified and have the ability to earn high risk-adjusted returns.
By understanding how different assets move in relation to each other, investors can create portfolios that minimize risk while still providing the potential for quality returns.
Covariance is just one tool that traders and investors can use to measure risk and return potential, but it is an important one.
What Is a Covariance Matrix?
A covariance matrix is a table that shows the covariance between two or more variables.
The matrix can be used to calculate the variance of a portfolio, as well as the correlation between assets in the portfolio.
The covariance matrix can also be used to identify which assets are likely to move in the same direction (positive covariance) or in opposite directions (negative covariance).
This information can be helpful when building efficient, balanced portfolios.
Formula for Covariance
Covariance is typically represented by the following formula:
Cov(X,Y) = Σ [(Xi – Xbar)(Yi – Ybar)] / (n-1)
Where:
- X and Y are the two variables
- Xi and Yi are the individual values of X and Y
- Xbar and Ybar are the means of X and Y
- n is the number of observations
- Σ is the summation symbol
This formula can be used to calculate the covariance between two variables, X and Y.
The Covariance Matrix can be used to calculate the variance of a portfolio, as well as the correlation between assets in the portfolio.
What Is the Difference in Covariance vs. Correlation?
It is important to note the difference between covariance and correlation.
Covariance is a measure of the relationship between two variables, while correlation is a measure of the strength of that relationship.
Covariance can be positive or negative, while correlation is typically expressed as a positive or negative number between minus-1 (perfect negative correlation) and positive-1 (perfect positive correlation).
This means that two variables, for example, can have a high degree of covariance but a low degree of correlation.
For example, two assets may move in the same direction (positive covariance) but to different degrees (low correlation), such as GDP and revenue figures for a certain company, or GDP and stock prices.
Covariance is just one metric that investors can use to measure risk and return potential, but it’s an important one.
By understanding how different assets move in relation to each other, investors can build portfolios that lower risk while still providing the potential for quality returns.
Covariance and CAPM
Covariance is part of the Capital Asset Pricing Model (CAPM) via beta.
Covariance is a statistical measure that calculates the degree to which two variables vary together.
Covariance can be positive or negative, and it is typically represented by a covariance matrix.
Covariance is used in finance, investing, trading, and economics to measure the relationships between asset returns.
Covariance can be helpful in constructing efficient, well-diversified portfolios, as it can help inform analysts which assets are likely to move in the same direction (positive covariance) or in different directions (negative covariance).
The Capital Asset Pricing Model (CAPM) is a model that describes the relationship between risk and expected return.
The model states that the expected return of an asset is equal to the risk-free return plus a risk premium.
The risk premium is determined by the beta of the asset.
Beta is a measure of the volatility of an asset in relation to the market. Covariance is used in calculating beta.
Covariance and portfolio risk
Covariance is a statistical measure that calculates the degree to which two variables vary together.
Covariance can be positive or negative, and it is typically represented by a covariance matrix.
Positive covariance
Positive variance means that the variables move in the same direction, such as two different stocks.
Negative covariance
Negative covariance means that the variables move in opposite directions.
During certain times in history, stocks and bonds have had negative covariance, but this doesn’t always hold true.
Covariance vs. Variance
Portfolio risk is the risk associated with a portfolio of investments.
There are various types of risk, such as liquidity risk, credit risk, interest rate risk, currency risk, as well as general volatility.
Portfolio risk can be measured by calculating the variance of the portfolio.
The higher the variance, the higher the risk.
Covariance is used to calculate variance. Covariance is a measure of the relationship between two variables, while variance is a measure of the variability of a single variable.
Covariance can be positive or negative, while variance can only be positive. The lowest value it can take on is zero.
Covariance and standard deviation
Standard deviation is a measure of the variability of a data set.
Covariance is used to calculate standard deviation.
Covariance is a measure of the relationship between two variables, while standard deviation is a measure of the variability of a single variable.
Covariance can be positive or negative, while standard deviation can only be positive.
How to Calculate Covariance
Assume you have five quarters of GDP data that show how the growth of GDP (x) compares to the growth of the revenue of a company (y).
The data set appears as:
- Q1: x = 1, y = 7
- Q2: x = 2.5, y = 8
- Q3: x = 3.5, y = 10
- Q4: x = 3, y = 8
- Q5: x = 5, y = 12
The average x value equals 3, and the average y value equals 9.
To calculate the covariance, we need to add up the products of the xi values minus the average x value, multiplied by the yi values minus the average y values would be divided by (n-1), as follows:
Cov(x,y) = ((1 – 3) x (7 – 9) + (2.5 – 3) x (8 – 9) + (3.5 – 3) x (10-9) + (3 – 3) x (8 – 9) + (5 – 3) x (12 – 9)) / 4 = (4 + 0.5 + 0.5 + 0 + 6) / 4 = 2.75
As there’s a positive covariance in this case, we can make the conclusion that the growth of the company’s goods and services has a positive relationship with quarterly GDP growth.
Covariance – FAQs
What is a covariance matrix?
A covariance matrix is a matrix that shows the covariance between two or more variables.
The matrix is symmetrical, with the diagonal entries showing the variances of the individual variables.
The off-diagonal entries show the covariances between the variables.
What is an example of covariance?
An example of covariance would be if two stocks moved in the same direction.
If Stock A went up 10 percent and Stock B went up 5 percent, then there would be positive covariance between these two stocks.
How to calculate covariance in Excel?
To calculate covariance in Excel, you can use the COVAR function.
This function calculates the Covariance of two supplied sets of values.
For example, to calculate the covariance from the above example, you would use the following formula, assuming the data were laid out in cells A1 to A5 and B1 to B5:
=COVAR(A1:A5,B1:B5)
This would return a Covariance value of 2.75.
What is covariance in statistics?
In statistics, covariance is a measure of how two random variables change together.
Covariance can be positive, negative, or zero.
A positive covariance means that the variables tend to move in the same direction, while a negative covariance means that the variables tend to move in opposite directions.
What is the difference between variance and covariance?
The main difference between variance and covariance is that variance is a measure of the variability of a single variable, while covariance is a measure of the relationship between two variables.
Covariance can be positive or negative, while variance can only be positive.
Another key difference is that correlation is a measure of the strength of the relationship between two variables, while Covariance is a measure of the variability of two variables.
What is the difference between covariance and correlation?
The main difference between covariance and correlation is that covariance is a measure of the variability of two variables, while correlation is a measure of the strength of the relationship between two variables.
Covariance can be positive or negative, while correlation can only be positive.
How do you interpret covariance?
Covariance can be interpreted as a measure of how two variables move together.
A positive covariance means that the variables tend to move in the same direction, while a negative covariance means that the variables tend to move in opposite directions.
A high covariance value indicates that there is a strong relationship between the two variables, while a low covariance value indicates that there is a weak relationship between the two variables.
What are some real-world applications of covariance?
Covariance can be used in portfolio management to measure the risk of a portfolio.
Covariance can also be used in regression analysis to determine which independent variables are most predictive of the dependent variable.
Covariance can also be used to calculate the correlation between two variables.
What are some limitations of covariance?
One limitation of covariance is that it can be difficult to interpret.
Another limitation is that covariance does not take into account the units of measurement for the variables, which can make it difficult to compare two variables.
Finally, covariance only measures linear relationships between two variables, and does not take into account non-linear relationships.
What are some other measures of variability?
Some other measures of variability include standard deviation, range, interquartile range, and variance.
Can covariance be negative?
Yes, covariance can be positive or negative.
A positive covariance means that the variables tend to move in the same direction, while a negative covariance means that the variables tend to move in opposite directions.
What is an advantage of the correlation coefficient over the covariance?
The correlation coefficient is a standardized measure of the relationship between two variables, which means that it is easier to compare the relationship between two variables.
The correlation coefficient is also easier to interpret than covariance. For example, it’s more intuitive to interpret what a correlation coefficient of +0.20 means (weak positive correlation) versus a covariance of 2.
What are some other measures of association?
Some other measures of association include the Pearson correlation coefficient, Spearman rank correlation coefficient, and Kendall tau correlation coefficient.
What is sample covariance?
Sample covariance is a measure of the variability of two variables that is calculated using a sample of data.
What is population covariance?
Population covariance is a measure of the variability of two variables that is calculated using all the data in a population.
Population covariance can be positive or negative, and is used to estimate the population variance.
What is the difference between sample Covariance and population Covariance?
Population variance refers to the value of variance that is calculated from population data, and sample variance is the variance calculated from sample data.
How do you calculate Covariance?
Covariance can be calculated by taking the product of the deviation of each variable from its mean, and then dividing by the number of observations.
Conclusion – Covariance
Covariance is used in statistics as a measure of how two random variables change together.
Covariance can be positive, negative, or zero.
In terms of how to interpret covariance, a positive covariance means that the variables tend to move in the same direction, while a negative covariance means that the variables tend to move in opposite directions. A covariance of zero suggests neither.
Covariance is also used in portfolio management to measure a portfolio’s risk and in regression analysis to determine which independent variables are most predictive of the dependent variable.
Covariance is a measure of variability, but there are other measures of variability including standard deviation, range, interquartile range, and variance.
The correlation coefficient is a standardized measure of the relationship between two variables, which means that it is easier to compare the relationship between two variables. The correlation coefficient is also easier to intuitively understand than covariance, which is a big part of its popularity.
Some other measures of association include the Pearson correlation coefficient, Spearman rank correlation coefficient, and Kendall tau correlation coefficient.
Sample covariance is a measure of the variability of two variables that is calculated using a sample of data while population covariance is a measure of the variability of two variables that is calculated using all the data in a population.