Volatility-Based Betting Strategies in Trading
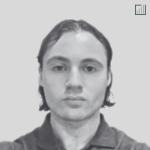
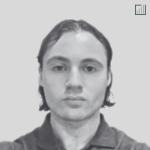
Volatility-based betting strategies are more sophisticated approaches to trading that adjust position sizes based on the underlying volatility of assets or return streams.
These methods try to optimize risk-adjusted returns by either sizing based on risk levels or dynamically changing exposure as market conditions change.
Traders who use these strategies recognize that volatility isn’t just a measure of risk but also a metric for position sizing and risk management.
Key Takeaways – Volatility-Based Betting Strategies
- Dynamic Position Sizing
- Adjust trade sizes based on market volatility to maintain consistent risk exposure.
- Volatility Normalization
- Standardize returns across assets by dividing by volatility (adjusting for risk, returns are fairly similar).
- This allows fair comparison and allocation between high and low volatility instruments.
- Regime Awareness
- Recognize distinct market volatility regimes.
- Adapt strategies for high vs. low volatility periods to optimize performance and risk management.
- Risk Premium Capture
- Exploit the spread between implied and realized volatility.
- Selling options and delta-hedging can potentially profit from overpriced implied volatility.
- We give a portfolio example in the article.
The Importance of Volatility in Trading
Volatility creates opportunities for profit and poses risks of loss.
Understanding and harnessing volatility can be a viable strategy for any serious trader.
Taking volatility into account can help traders potentially improve returns while managing downside risk (e.g., drawdowns, length of underwater periods) more effectively.
Fundamental Concepts
Defining Volatility
Volatility measures the dispersion of returns for a given security or market index.
It quantifies the degree of variation in a trading price series over time.
High volatility indicates rapid and significant price fluctuations, while low volatility suggests more stable prices.
Note that volatility generally applies to liquid markets.
Illiquid markets aren’t regularly marked to market so there are other measures of risk quantification for such markets (e.g., operational risk, credit risk, liquidity risk).
Types of Volatility Measures
Several volatility measures exist, each with its strengths and applications:
- Historical volatility – Calculated using past price data.
- Implied volatility – Derived from options prices, reflecting market expectations of future volatility.
- Realized volatility – The actual volatility observed over a specific period.
- Conditional volatility – Estimated using models like GARCH, which account for volatility clustering.
- Tail risk – Not necessarily volatility, but a type of risk related to how much can be lost.
The Relationship Between Volatility and Risk
While volatility and risk are related, they’re not synonymous.
Volatility can create opportunities for skilled traders.
It also nonetheless increases the potential for large losses.
The key is to use volatility as a framework for calibrating position sizes and managing overall portfolio risk.
Core Principles of Volatility-Based Betting
The Kelly Criterion
The Kelly Criterion is a popular framework in many volatility-based betting strategies.
It suggests that the optimal bet size is a function of the edge (expected value) and the odds.
In trading, this translates to adjusting position sizes based on the expected return and the underlying volatility of the asset.
Volatility Normalization
Volatility normalization is a technique used to create comparable risk exposures across different assets or time periods.
Dividing returns by their corresponding volatilities enables traders to create a standardized measure of risk-adjusted performance.
This allows for more consistent position sizing across diverse market conditions.
Example
Dividing a stock’s return by its volatility standardizes risk.
This makes it easier to compare with other assets and compares how much return you’re getting per unit of risk.
For example, if a stock gets 6% return at 15% annualized volatility, that’s 0.4% units of return per each percentage point of volatility.
If a bond gets them 5% return for 10% annualized volatility, that’s 0.5% units of return per 1% of volatility.
For risk parity traders, they might want to figure out a way to leverage the bonds portion to make it commensurate with the risk of the stocks/risk assets portion.
This helps prevent the stocks part dominating in terms of risk and having better diversification, which improves one’s returns relative to the risks.
The Inverse Volatility Rule
A simple yet effective approach is the inverse volatility rule.
This strategy allocates capital inversely proportional to an asset’s volatility.
Assets with lower volatility receive larger allocations, while more volatile assets get smaller positions.
This method tries to equalize risk contributions across a portfolio.
Practical Implementation Strategies
Volatility Targeting
Volatility targeting involves adjusting position sizes to maintain a constant level of portfolio volatility.
As market volatility increases, position sizes are reduced, and vice versa.
This approach can help smooth out returns and manage risk more consistently over time.
Steps in Volatility Targeting
- Set a target portfolio volatility (e.g., 10% annualized).
- Estimate the current volatility of the asset or portfolio.
- Adjust position sizes to align the current volatility with the target.
Example
If the target volatility is 10% and the current market volatility is 20%, the position size would be reduced by half (10% / 20% = 0.5) compared to a normal market environment.
Those who want to have more volatility will use leverage while those who want less can hold cash against assets.
Volatility Breakout Systems
Volatility breakout systems use volatility to define entry and exit points.
These strategies often use multiples of the average true range (ATR) or standard deviation to set stop-loss and take-profit levels.
Position sizes are adjusted based on the current volatility, so that the risk per trade remains constant in terms of portfolio value.
Time-Based Volatility Adjustments
Markets often exhibit predictable patterns of volatility throughout the trading day or week.
Some traders adjust their bet sizes based on these temporal patterns.
For instance, they might increase position sizes during typically calmer periods and reduce them during known volatile times, like market opens or economic releases.
Example Portfolio
Let’s do an example portfolio of how to allocate risk if these are your returns and risks (these are just example, not necessarily what matches the real world):
- Stocks: 6% returns, 15% vol
- Bonds: 4% returns, 10% vol
- Commodities: 10% returns, 25% vol
- Gold: 8% returns, 20% vol
From these, we can see equal return/risk ratios.
Using the inverse volatility rule, we’ll allocate capital inversely proportional to each asset’s volatility.
This approach tries to equalize risk contributions across the portfolio.
Calculate Inverse Volatilities
Calculate inverse volatilities:
- Stocks: 1/15 = 0.067
- Bonds: 1/10 = 0.10
- Commodities: 1/25 = 0.04
- Gold: 1/20 = 0.05
Sum the inverse volatilities: 0.0667 + 0.1000 + 0.0400 + 0.0500 = 0.2567
Calculate Allocation Percentages
- Stocks: 0.0667 / 0.2567 = 26.0%
- Bonds: 0.1000 / 0.2567 = 38.9%
- Commodities: 0.0400 / 0.2567 = 15.6%
- Gold: 0.0500 / 0.2567 = 19.5%
This allocation equalizes risk contributions, with lower volatility assets receiving larger allocations.
Bonds get the highest allocation due to lowest volatility, while commodities get the lowest due to highest volatility.
Expected portfolio return = (26.0% * 6%) + (38.9% * 4%) + (15.6% * 3%) + (19.5% * 5%) = 4.6%
The trader could then calculate the expected volatility of the portfolio, but this would require correlation analysis and/or backtesting.
Leverage would become a factor if they wanted to engineer higher or lower risk.
Note: This approach focuses solely on volatility. In practice, consider other factors like correlations, expected returns (if they aren’t proportional), and goals when constructing a portfolio.
Advanced Volatility-Based Strategies
Volatility Risk Premium Harvesting
The volatility risk premium (VRP) is the difference between implied and realized volatility.
Sophisticated traders often design strategies to capture this premium.
One approach involves selling options (which are priced based on implied volatility) and delta-hedging to capture the spread when realized volatility is lower than implied.
This is hard to do efficiently without software and due to transaction costs.
Volatility Regime Switching
Markets tend to exhibit distinct volatility regimes – periods of high and low volatility that can persist for extended times.
Advanced traders use statistical techniques to identify these regimes and adjust their strategies accordingly.
During high-volatility regimes, they might reduce overall exposure or shift to more defensive assets or diversify with more market-neutral or negative correlation strategies.
Cross-Asset Volatility Arbitrage
This strategy exploits inconsistencies in volatility pricing across related assets.
Traders might identify pairs of assets where the relative volatility levels seem out of sync with historical norms or fundamental relationships.
They then take opposing positions, betting on a convergence of volatility levels.
Risk Management in Volatility-Based Betting
Tail Risk Protection
Volatility-based strategies can improve risk-adjusted returns, but they’re not immune to extreme events.
Using tail risk protection is important.
This might involve:
- purchasing out-of-the-money options
- using dynamic hedging techniques, or
- maintaining a portion of the portfolio in safe-haven assets – i.e., thinning out the left tail has to do with careful structuring of the portfolio
Correlation Considerations
Volatility-based strategies often assume a degree of independence between assets.
However, correlations may spike during market stress when a lot of things get liquidated.
Crypto, a type of speculative alternative form of currency, may correlate highly with riskier stocks (and stocks in general).
Sophisticated traders incorporate correlation modeling into their volatility-based approaches and adjust position sizes to account for potential correlation breakdowns.
Liquidity Management
Volatility can dry up market liquidity, especially for less liquid assets.
Traders have to consider how changes in volatility might affect their ability to enter or exit positions.
For this reason, quants will often integrate liquidity metrics into the position sizing algorithm can help reduce this risk.
Challenges & Limitations
Model Risk
Volatility-based strategies often rely on complex statistical models.
These models aren’t practical for all traders to build and may not be effective when important variables aren’t in them, especially during regime shifts or extreme market events.
Regular backtesting and out-of-sample validation are essential to reduce model risk.
Transaction Costs
Frequent position adjustments, which are common in volatility-based strategies, can lead to high transaction costs.
These costs can erode returns, especially in less liquid markets due to the spread.
Traders must carefully balance the benefits of dynamic sizing against the drag of transaction costs.
Behavioral Challenges
Implementing volatility-based betting strategies requires discipline and emotional control.
Traders may find it psychologically difficult to increase position sizes during periods of low volatility (low vol doesn’t last) or to dramatically reduce exposure when volatility spikes (high vol may be temporary).
Developing strong systems and adhering to predefined rules can help overcome these behavioral hurdles.
Even for discretionary traders, rules can be important.
The Future of Volatility-Based Trading
As markets evolve and technology advances, volatility-based betting strategies will become increasingly sophisticated.
Machine learning algorithms uncover new patterns in volatility behavior, which lead to more nuanced position sizing models.
High-frequency trading firms are already using microsecond-level volatility measures to inform their strategies.
Moreover, the growing availability of volatility-based financial products, such as VIX futures, options, and OTC derivatives, provides traders with new methods for implementing and hedging volatility strategies.
Conclusion
Volatility-based betting strategies offer a framework for managing risk and better optimizing returns in trading.
Dynamically adjusting position sizes based on market conditions or the risk associated with an asset enables traders to potentially achieve more consistent performance across various market regimes.
It requires an understanding of statistical concepts, strong risk management practices, and the discipline to adhere to more systematic approaches.
As with any trading strategy, there’s thorough research, careful testing, and ongoing monitoring.
Volatility-based betting can improve trading outcomes, but it’s just one idea in a broader toolkit to getting the most out of your portfolio.
Related: How to Build a Balanced Portfolio