Randomness in Trading
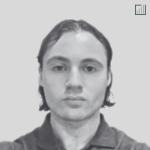
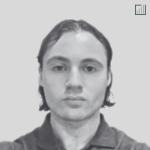
Understanding randomness is important for traders and investors.
It shapes risk management strategies, the effectiveness of trading algorithms, and decision-making processes.
The skill vs. luck question is especially relevant for new traders, as any initial success or failure may be attributed to one or the other without actually knowing what’s causing or leading to what.
Here we look at randomness in trading, examining its sources, implications, and strategies traders use to deal with it.
Key Takeaways – Randomness in Trading
- Recognize Randomness – Markets are unpredictable will be affected by factors beyond whatever form(s) of analysis you’re using.
- Skill vs. Luck – Early wins or losses may stem from luck rather than skill, especially for new traders. Understanding this prevents misattribution. Trading, no matter the timeframe (e.g., day trading, swing trading, investing), is a long game.
- Risk Management – In light of what can’t be known, prepare for unexpected outcomes with strategies like diversification, using options, stop-loss orders, and careful position sizing.
- Probabilistic Thinking – Thinking in probabilities rather than certainties allows for better decision-making.
- Stay Emotionally Resilient – Losses and drawdowns are inevitable. Focusing on long-term strategy helps maintain discipline and reduce stress.
Examples of Randomness in Markets
Let’s start with some examples.
Take a look at these three profit/loss graphs showing a strategy’s performance over one year (approximately 252 trading days for most stocks and bonds).
Graph A
This strategy didn’t do too well, down more than 20% after one year, and had a drawdown of nearly 30%.
Graph B
This strategy was mixed.
At one point it was up almost 20%, before going all the way back into the red slightly before the year was almost over, before finishing up close to 10%.
Graph C
This strategy did the best, up over 30% at one point before finishing up around 25% for the year.
After an initial dip at the beginning, it went up fairly well consistently.
Of All These Strategies, Which Would You Choose?
We want whatever Graph C is, right?
Well, the deal is… they’re all the same strategy!
In this case, I simply coded an example where a trader starts with $10,000, bets 1% of his balance on each trade, and has a 50/50 likelihood of being right or wrong – not far off what most traders deal with.
It’s basically no different than coin-flipping.
What can seem like skill may simply be favorable variance.
Randomness in Trading vs. Randomness in Poker
Let’s make a comparison we’ve made before – trading and poker.
They’re both games of exploiting edges, risk management, and dealing with randomness.
Both traders and poker players share an understanding of the relationship between signal, statistically relevant outcomes, and randomness.
Here’s a breakdown:
In trading…
Signal vs. Noise
Top traders are good at identifying real signals (indicators of future price movements) versus the noise of random market fluctuations. (At least noise in the sense that it’s outside the purview of their trading strategy.)
They develop strategies based on an understanding of the cause-effect mechanics of what they’re trying to do, data analysis, and backtesting, not hunches or fleeting trends.
They’re looking for something that’s ideally replicable over time.
They know that consistently profitable trading comes from exploiting statistically significant edges.
Risk Management
Even with a strong signal and a high-probability trade, randomness can still lead to losses in the short term.
In a separate article, we looked at the idea of how long would you need to test a trading strategy with various types of edges based on trade frequency.
For example, a 51% edge on an even gain/even loss bet – assuming that holds after transaction costs – is winning in the long run. But it might take a while to see the results.
For a casino, a single hand of blackjack might give them a 1-2% edge if the customer is playing perfect basic strategy, but the short-term variance can still expose them.
This is why they view things over a long-term time horizon.
Top traders understand this and manage their risk.
They size their positions appropriately to withstand inevitable losing streaks and protect their capital through various measures – e.g.:
- diversification
- watching their correlations
- using options to limit/eliminate tail risk
- stop-losses
- having sufficient liquidity
They focus on the long game, knowing that their edge will play out over time if the analysis is correct.
For poker players…
Expected Value
Top poker players think in terms of expected value (EV).
They calculate the long-term profitability of their decisions based on probabilities and potential payouts.
They know that even if they have the best hand, they might still lose sometimes due to the luck of the draw.
However, by consistently making +EV decisions, they ensure profitability in the long run.
Bankroll Management
Variance (short-term fluctuations in results) is a constant in poker.
Top players understand this and manage their bankroll carefully.
They avoid risking too much on any single hand or tournament.
That way, they can weather downswings and stay in the game.
The Common Thread
Both top traders and poker players:
- Embrace probabilistic thinking – They understand that the world is uncertain and make decisions based on probabilities, not guarantees.
- Focus on process over outcome – They prioritize making sound decisions based on their strategy, knowing that positive results will follow in the long run.
- Have self-awareness – Self-awareness is hard in trading at first. It’s fairly easy to dive in and make trades and maybe even have some initial luck. But new traders unfortunately won’t know what they don’t know. Experienced traders know that the range of knowns is low in relation to the range unknowns relative to what’s discounted in the price. They not only try to know what they don’t know, but also recognize they don’t know what they don’t know.
- Manage risk effectively – They understand the role of randomness and protect their capital from unacceptable outcomes.
- Continuously learn and adapt – They analyze their performance, identify areas for improvement, and refine their strategies over time.
Essentially, they’re both skilled at making calculated decisions in environments with inherent uncertainty, focusing on long-term profitability rather than short-term results.
Even though the “day” in day trading may emphasize the day itself, it’s one big long session.
Understanding Randomness in Financial Markets
So randomness, in the context of financial markets, refers to the lack of a predictable pattern or discernible order in asset price movements.
Some factors, such as economic indicators and corporate earnings, may have predictable influences, but many events are random or influenced by variables that aren’t even factored into the analysis.
This unpredictability stems from, e.g., human behavior, economic complexities, external events.
Randomness vs. Determinism in Trading
Financial markets are neither entirely random nor entirely deterministic.
Determinism suggests that every outcome is the result of specific causes.
In a purely deterministic market, traders could predict prices with certainty based on all relevant variables.
However, markets exhibit elements of both determinism and randomness.
We know that certain actions create certain outcomes – e.g., money and credit spent divided by the quantity of it available determines price.
But there’s a lot of variance in far too many variables to know anything precisely.
Types of Randomness in Trading
Randomness in trading can be classified into two broad categories:
- Systematic Randomness – This stems from complex interactions within the financial system, like interest rates, macroeconomic indicators, and geopolitical events. Systematic randomness affects multiple assets and markets simultaneously.
- Idiosyncratic Randomness – Specific to individual assets or events, such as a company’s earnings report or the resignation of a CEO. Idiosyncratic randomness affects single stocks or commodities without necessarily impacting the broader market.
Both forms of randomness contribute to a level of randomness in markets, requiring strategies to manage their impacts.
Random Walk Theory
The Random Walk Theory posits that stock prices move in an unpredictable pattern, which by extension means it asserts it’s impossible to predict future prices based on past performance.
This theory has implications for traders, especially those who rely heavily on historical data.
Implications of the Random Walk Theory
If stock prices truly follow a random walk, then traditional forms of analysis might be limited in their effectiveness.
Some proponents of the random walk view advocate for passive strategies, arguing that since stocks are unpredictable, it’s better to go with diversified portfolios rather than attempting to outperform the market.
This gets into the efficient market hypothesis. While flawed, it’s a good starting point for most market participants, as it’s not at all easy to have an edge.
Limitations of the Random Walk Theory
Despite its popularity, the Random Walk Theory has limitations.
Certain traders do have replicable advantages in markets, whether that’s due to an analytical edge, informational edge, technological edge, or something else.
How to Measure Randomness in Trading
Randomness in trading can be measured through several analytical methods and statistical techniques/concepts/frameworks designed to quantify unpredictability and evaluate the role of chance in price movements.
Since randomness and its degree is so important in trading outcomes, understanding its measurement is relevant for risk management, strategy formulation, and understanding the reliability of trading patterns.
Here are some key methods for measuring randomness in trading.
1. Volatility Analysis
Volatility is a commonly used measure of randomness and uncertainty in the market.
High volatility indicates larger price fluctuations, which suggests a higher degree of randomness in the asset’s movements.
Volatility can be measured using historical volatility (calculated from past price data) or implied volatility (derived from options prices, reflecting market expectations for future volatility).
The more volatile an asset is, the less predictable it becomes, which means a higher level of randomness in short-term price movements (and potentially long-term too).
Traders often calculate the standard deviation of returns to assess volatility.
A higher standard deviation suggests a greater dispersion of price movements, reflecting more randomness.
Many trading strategies target volatility, as one form of risk.
2. Autocorrelation Tests
Autocorrelation tests measure the correlation of an asset’s returns over time, indicating whether patterns in price movements are statistically significant or random.
If an asset’s price movements show low or no autocorrelation, it suggests that the returns are random and follow a pattern similar to a random walk, where each price change is independent of the last.
Common autocorrelation tests include the Ljung-Box test and Durbin-Watson test.
These statistical tests assess whether there’s any significant autocorrelation at different time lags in asset prices.
High autocorrelation may indicate predictability, while a lack of it suggests randomness.
3. The Random Walk Hypothesis
To test the Random Walk Hypothesis, traders and analysts often use statistical methods such as variance ratio tests and unit root tests (like the Augmented Dickey-Fuller test).
If the tests indicate that price movements resemble a random walk, it reinforces the view that randomness plays a dominant role in asset prices.
4. Monte Carlo Simulations
Monte Carlo simulations involve generating multiple hypothetical price scenarios based on price data (historical or manually inputted) and probabilities, which helps traders understand the range of possible outcomes and their distribution (i.e., which are more or less common).
In trading, Monte Carlo simulations are used to model the likelihood of various trading scenarios and to measure the impact of randomness on portfolio performance.
By running thousands of simulations, traders can understand how randomness and market variability may impact long-term results.
For example, Monte Carlo simulations can estimate the probability of an asset reaching a certain price level within a set period, factoring in randomness through randomly generated price paths.
5. Entropy and Information Theory
Entropy is a measure from information theory that quantifies uncertainty or randomness in a data set.
Applied to trading, entropy can measure the unpredictability of asset price movements.
A high entropy level indicates that there is significant uncertainty in the data, suggesting randomness/dispersion.
Conversely, a lower entropy level implies more predictability and structure within the price movements.
Traders and analysts use entropy to evaluate whether certain patterns or trends in price data hold important information or are simply noise.
Techniques like Shannon entropy or approximate entropy can help understand the degree of randomness in an asset’s price history.
6. Hurst Exponent
The Hurst exponent is a statistical measure used to determine the degree of randomness in a time series.
Values close to 0.5 indicate a random walk (pure randomness), values above 0.5 suggest persistent trends, and values below 0.5 suggest mean-reverting behavior.
From calculating the Hurst exponent for an asset’s price series, traders can gauge whether price movements are purely random or show any underlying trends.
Sources of Randomness in Trading
Randomness in trading can come from various sources, including economic factors, human psychology, technological influences, and geopolitical events.
Economic and Market Factors
Economic data releases, such as employment reports, inflation figures, and interest rate announcements, are often unpredictable (even for those whose jobs are to accurately predict them), injecting randomness into the market.
For example, an unexpected increase in inflation could trigger sudden shifts in stock prices as traders adjust their expectations about interest rates and economic growth.
There are also multi-order effects and interdependencies that are difficult to predict.
The market has many different kinds of buyers and sellers, who have all sorts of motivations for doing what they’re doing.
Psychological and Behavioral Factors
Psychology contributes significantly to randomness.
Human emotions like fear, greed, and overconfidence can cause market behavior that doesn’t make sense from traditional analysis perspectives.
Behavioral finance studies, such as those focusing on herding or overreaction, show how collective human behavior adds a layer of unpredictability to markets.
For instance, during a market rally, traders may follow the crowd and buy simply because that’s what everyone else is doing, causing price movements that don’t align with fundamentals.
Technological Influences
Many algorithms react to market data within milliseconds, leading to rapid price changes that discretionary traders can’t anticipate.
Geopolitical Events
Geopolitical events, such as elections, trade conflicts, military conflicts, or diplomatic tensions, are difficult to predict but have impacts on financial markets.
For instance, an unexpected tariff announcement can cause currency fluctuations and impact commodity prices.
Geopolitical randomness often triggers high volatility, especially in sensitive sectors like energy and defense.
Implications of Randomness for Traders
Risk Management
Traders have to acknowledge the possibility of adverse outcomes even with well-informed decisions.
Strategies such as diversification, using options, stop-loss orders, and position sizing help limit potential losses caused by unpredictable events.
Risk management accounts for randomness by preparing for both expected and unexpected market movements.
Importance of Probabilistic Thinking
Traders benefit from thinking probabilistically rather than relying on certainties.
Probabilistic thinking involves estimating the likelihood of various outcomes and preparing for multiple scenarios.
This mindset helps traders avoid overconfidence and enables them to weigh potential gains against possible risks.
For example, traders may use Monte Carlo simulations to model different market scenarios and understand the range of possible outcomes.
They can use that information to help them do things better, such as diversifying so that they’re balanced toward various outcomes.
Limits of Predictive Models
Predictive models are important, but their effectiveness is limited by the inherent randomness of markets.
Even the most sophisticated models are simplifications of reality and can’t account for all variables, particularly those influenced by human behavior or unexpected news events.
We discussed in a previous article that markets are what chaos theory refers to as “Level 2” systems, where human predictions and expectations become part of the system itself, which is what makes forecasting markets so much different than forecasting “Level 1” systems like the weather.
The Psychological Impact of Randomness on Traders
Randomness can take a psychological toll on traders, as it can affect their decision-making and emotional resilience.
Understanding how randomness influences emotions is important for sticking to your plan.
Overconfidence and Randomness
Overconfidence is a common psychological bias in trading, leading traders to believe they can predict outcomes with certainty.
Randomness in the market can exacerbate this bias – i.e., traders may attribute random successes to skill rather than luck.
Experience tends to teach traders to remain humble and avoid overestimating their abilities.
Dealing with Losses and Uncertainty
Randomness can lead to frustration and emotional stress, particularly after unexpected losses.
Traders who understand the role of randomness and variance are better able to view losses as part of the trading process rather than personal failures.
Having a probabilistic mindset helps traders remain resilient and focus on long-term success rather than individual outcomes.