Jim Simons Trading Strategy [Renaissance Technologies, Medallion Fund]
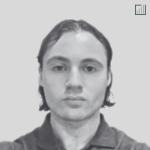
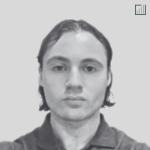
In finance, few names are as synonymous with success as Jim Simons, the head of Renaissance Technologies (RenTech) and its famed Medallion Fund.
By leveraging his background in mathematics and using the power of quantitative trading strategies, Simons built Renaissance into one of the most successful investment management firms.
In this article, we will explore the story of Jim Simons, his trading strategy, and the impact he’s had in finance through Renaissance Technologies and the Medallion Fund.
We’ll also look at quantitative trading and its role in shaping modern investment strategies.
Key Takeaways – Jim Simons Trading Strategy
- Jim Simons’ success in finance was largely due to his background in mathematics and its application into quantitative trading strategies.
- His approach largely involves finding patterns in financial data that repeat over time and modeling these into algorithms to profit from future price movements.
- Renaissance Technologies, the hedge fund co-founded by Simons, is known for combining finance, mathematics, and computer science.
- The Medallion Fund, Renaissance Technologies’ internal fund, isn’t open to outside investors and its trading strategies are closely guarded.
- While quantitative trading has many advantages, there are some risks and drawbacks, such as model risk, overfitting, and technological issues.
Who Is Jim Simons?
Jim Simons is an American mathematician, investor, and philanthropist best known as the co-founder of Renaissance Technologies, a quantitative hedge fund.
Born in 1938, Simons received his Ph.D. in Mathematics from the University of California, Berkeley, and went on to teach at several universities.
His work in mathematics, particularly in the field of geometry and topology, earned him widespread recognition.
In 1982, well into his 40s by that point, Simons co-founded Renaissance Technologies, transitioning from academia to finance, and quickly made a name for himself with his quantitative trading strategies.
What Is the Jim Simons Trading Strategy?
The Jim Simons trading strategy primarily revolves around quantitative trading, using mathematical models and algorithms to identify patterns and make investment decisions.
While this is all proprietary, Simons has described the basics of his trading strategy as identifying patterns in data that repeat over time, and modeling these into algorithms to profit from future price movements.
Simons described financial patterns as seeming somewhat random (i.e., to the naked eye when looking at historical price patterns), but not totally random.
With technology, the computer is able to process vast amounts of financial data, minimizing human biases and emotions while maximizing investment returns. Simons’ strategy relies on a diverse team of researchers, mathematicians, and computer scientists who continually develop and refine the models that drive the firm’s trading decisions.
Gregory Zuckerman wrote a book The Man Who Solved the Market on Simons’ and RenTech’s trading.
Nevertheless, it was lacking on technical insight and detail on what the actual strategy is.
Renaissance Technologies
Renaissance Technologies, founded in 1982 by James Simons and James Ax, is a hedge fund known for its quantitative trading approach.
Headquartered in New York, the firm manages billions of dollars in assets and has produced strong returns for its investors.
Its success has been attributed to the firm’s unique culture that combines finance, mathematics, and computer science.
Is RenTech a HFT Firm?
RenTech is not primarily classified as a high-frequency trading (HFT) firm.
RenTech is best known generally as a quantitative investment management company, using complex statistical and mathematical models and algorithms to analyze data and make trading decisions.
While they do employ sophisticated technology and rapid computation, their strategies are distinct from typical HFT firms, which focus on executing a large number of orders at extremely high speeds.
RenTech’s approach is more centered around statistical arbitrage and long-term pattern recognition in market data, rather than the ultra-short-term strategies characteristic of HFT.
Medallion Fund
The Medallion Fund is Renaissance Technologies’ flagship fund.
Launched in 1988, the fund is believed to have consistently generated impressive returns, often outperforming other funds and market benchmarks.
The strategies behind Medallion Fund’s success are said to be proprietary quantitative trading strategies, which are closely guarded secrets.
The fund is known for its exclusivity, primarily serving the employees of Renaissance Technologies.
However, considering that the Medallion Fund is not open to outside investors, there are no regulatory filings about it, and no way to verify the return claims ever since it bought out its last outside investors, not much can be gleaned from it.
It has a reported fee structure of 5% of AUM and a 44% performance fee (higher than the typical “2 and 20”).
How does the Medallion Fund get such high returns?
Medallion Fund returns are not compounded over time, as the fund it capped at a certain level. All returns above this cap are distributed.
Some believe its purported high returns are from other income streams independent of standard trading, such as tax arbitrage.
Renaissance has had tax penalties of $7 billion in the past over a strategy known as basket options (explained below).
Others believe the Medallion Fund’s too-good-to-be-true returns are used as marketing for its funds that are open to outside investors (and don’t have anywhere near the same level of returns).
Performance gap between Medallion and other Renaissance funds (e.g., RIEF)
In 2008, the Medallion Fund reported a performance of +80% despite high fees.
On the other hand Renaissance Institutional Equities Fund (RIEF) – open to outsiders – declined by 16% that year, suffering losses like most funds during the financial crisis.
RIEF also lost money in 2009, while Medallion, of course, again reported extremely high returns after fees (+39%).
Why the gap?
Nobody knows, as it’s the quintessential “black box.”
Medallion & Renaissance Technologies Strategies
It’s believed that – and would accord with modern quantitative finance practices – the underlying philosophy of Simons’ approach hinges on the application of stochastic processes, machine learning, and various mathematical models to decode, analyze, and trade financial markets.
Below, we elaborate on the core components of this strategy:
1. Foundation in Stochastic Processes & Machine Learning
Initially, Renaissance Technologies distinguished itself by integrating stochastic processes and machine learning into financial analysis.
Stochastic processes are mathematical models that predict the probability of various outcomes under uncertain conditions, which are the basis of financial markets.
Machine learning, especially in its nascent stages within finance, offered a way to identify patterns and correlations in data that were not apparent to human analysts or traditional statistical and fundamental methods.
2. Development of Sophisticated Non-Linear Models
The progression towards non-linear and non-parametric models marked a significant evolution in their analytical capabilities.
Unlike linear models, which assume a direct proportional relationship between variables, non-linear models can capture more complex relationships, such as those influenced by external macroeconomic factors, market sentiment, and other non-quantifiable elements.
This complexity is better suited to the nature of financial markets, where linear assumptions often fall short.
3. Adoption of the Kernel Method
The kernel method is a breakthrough in pattern recognition and analysis within the context of Renaissance Technologies’ strategy.
It’s a technique in machine learning that allows the transformation of data into a higher-dimensional space to make it easier to model complex relationships between variables.
This method significantly enhanced their ability to predict market trends and price movements by identifying subtle patterns that weren’t detectable with simpler models.
4. Focus on Shorter-Term Trades
By focusing on shorter-term trades, Renaissance Technologies could capitalize on the statistical advantages from their models with reduced exposure to long-term market volatilities.
This approach minimizes the risk associated with holding positions over extended periods, where unforeseen macroeconomic and geopolitical events can have detrimental effects on trading returns.
5. Convergence Trades
RenTech is known for its use of convergence trades, which involve either relative value or arbitrage strategies.
In a convergence trade, they take a long position in an undervalued asset and a short position in a related overvalued asset, betting that the prices will eventually converge.
This could involve being long a stock and short a more expensive futures contract on the same stock, or being long an undervalued bond and short a more expensive but related bond.
By exploiting these temporary pricing inefficiencies, RenTech aims to generate consistent returns from the convergence of prices over time.
6. Scientific Approach to Market Prediction
Simons’ strategy has been likened to a scientific method of gambling, where mathematical probabilities, rather than intuition or speculative analysis, guide trading decisions.
This statistical edge is predicated on the law of large numbers and the central limit theorem, and provides a systematic way to achieve consistent returns over time.
7. Attraction of Multidisciplinary Talent
The recruitment of individuals like Ellyn Berlekamp, who brought expertise in game theory and information theory, showed the firm’s commitment to intellectual diversity and innovation.
A multidisciplinary approach enabled Renaissance to continually refine and enhance its models with fresh perspectives and methodologies.
8. Incorporation of Market Realities
Understanding and accounting for execution impacts and slippage was another factor.
Execution impact refers to the effect that large trades have on the market, potentially moving the price against the trade’s favor.
Slippage describes the difference between the expected price of a trade and the price at which the trade is executed.
By modeling these realities, Renaissance could more accurately predict net returns and optimize trade execution strategies.
Basket Options
Basket options are financial derivatives that allow investors to gain exposure to a portfolio of assets, such as stocks or commodities, rather than a single asset.
This type of option provides a more diversified risk profile than a standard option.
They were notably used by Simons and Renaissance as part of a strategy to convert short-term capital gains, which are taxed at a higher rate, into long-term capital gains, which enjoy a lower tax rate.
This approach was used to optimize the tax implications of their short-term trading activities.
Naturally, this practice has been scrutinized for its legality and ethical considerations.
RenTech & Basket Options
- Basket Options – RenTech reportedly used “basket options” with Deutsche Bank. These options are based on a collection of underlying assets (like a portfolio), rather than a single stock.
- Tax Motivation – One alleged reason for this structure was to defer taxes on gains. RenTech could essentially “lock in” a price without immediately selling, delaying tax implications.
- Regulatory Scrutiny – This practice garnered attention from the IRS and Senate investigations, questioning its legitimacy as a tax-deferral strategy.
Quant Trading
Quantitative trading, or quant trading, is a method of trading that uses mathematical models and algorithms to analyze financial markets and make investment decisions.
This data-driven approach allows quant traders to process and evaluate large amounts of information quickly and efficiently, identifying patterns and opportunities that may not be readily apparent to human traders.
Quant trading has grown in popularity over the years due to its ability to minimize human biases and emotions while generating consistent returns.
There are generally two forms of quant trading:
- expert systems (humans come up with the algorithms based on their understanding of the world)
- machine learning (the machine studies the data and derives algorithms from the patterns it spots)
What Mathematical Work Is Jim Simons Known For?
Jim Simons, before his finance career, was renowned for his work in mathematics, particularly in geometry and topology.
His most notable contribution is the Chern-Simons Theory, which he co-developed with Shiing-Shen Chern.
This theory, now part of differential geometry and topology, involves the study of 3-dimensional manifolds and the properties of geometric structures in differential space.
In basic terms, it provides a way to understand and classify the complexities of geometric shapes and spaces, particularly in how they twist and curve.
This mathematical framework is used not only in pure mathematics but also in theoretical physics, particularly in string theory and quantum field theory.
Jim Simons Quotes
“We don’t start with models. We start with data. We don’t have any preconceived notions. We look for things that can be replicated thousands of times.”
Instead of starting with a theory or hypothesis, Simons prioritizes analyzing raw data to identify patterns and trends.
They avoid imposing any pre-existing beliefs or biases, allowing the data to speak for itself.
Replicability highlights the importance of finding statistically significant patterns that hold true consistently across a large number of instances, so that the observed results are not due to chance or isolated incidents.
This approach is common in quantitative finance, where historical data is used to identify trading strategies with a statistical edge.
At the same time, others might be reluctant with this approach because such strategies that come out of this might struggle on forward data.
“Let’s suppose you have a coin that is 70/30 heads. Well, if you get to bet heads, you are going to win 7 times out of 10. Three times out ten you are going to lose, and that’s bad luck. So you need a measure of good luck to avoid a long run of tails when you have a 70/30 coin that’s heads. At a certain point the luck evens out. Of course there’s luck in our business, but so far we’ve had a nice edge.”
Here, Simons acknowledges the role of luck in any probabilistic endeavor, even when the odds are in your favor.
Using the analogy of a biased coin, he illustrates that even with a 70% chance of winning, losses are still inevitable.
Extended losing streaks can occur due to random chance, even with a statistical advantage.
For example, if you do 1,000 trials with a 70/30 win/loss probability, there’s still more than an 80% chance you’ll have at least one losing streak of 5 or more.
But over the long term, luck tends to balance out, and such an “edge” (the advantage provided by the biased coin) will lead to consistent gains.
We did a similar exercise in our article “How Long To Test a Trading Strategy” to simulate various types of edges.
In short, the smaller the edge, the longer it needs to be tested in order to be sure it actually is an edge – and to profit from it. And that’s assuming it lasts and doesn’t close altogether.
“There’s no such thing as the goose that lays the golden egg forever.”
This conveys the idea that no investment strategy or system can generate profits indefinitely.
At least in an alpha context (beta strategies should be passive and enduring).
Markets are constantly evolving with all the various types of buyers and sellers, the products traded, their sizes, their motivations, and so on.
He cautions against complacency and relying on past successes, implying that strategies must be constantly monitored and adjusted to maintain their edge.
It’s the same in other zero-sum games, like sports (i.e., wins and losses are zero-sum).
Strategies are always changing to get an edge, which means you’ll get evolution in the sport over time.
“Patterns of price movement are not random. However, they’re close enough to random so that getting some excess, some edge out of it, is not easy and not so obvious.”
While acknowledging the existence of patterns in price movements, it emphasizes that these patterns aren’t easily predictable.
Especially for a discretionary, non-data-dependent trader.
Simons suggests that markets exhibit a degree of randomness that makes it challenging to consistently extract profits.
Finding an “edge” requires sophisticated analysis and a deep understanding of financial markets.
It requires more than simply identifying basic trends.
It also emphasizes the competitive nature of financial markets, where various participants are trying to exploit the same patterns.
FAQs – Jim Simons Trading Strategy
How did Jim Simons transition from a career in mathematics to finance?
After a successful career in academia, Jim Simons saw potential in applying mathematical concepts to finance.
He co-founded Renaissance Technologies in 1982, where he focused on developing quantitative trading strategies.
His background in mathematics provided a strong foundation for creating and refining the algorithms that underpin the firm’s trading strategies, as well as identifying hires that could do well in such an environment.
What makes Renaissance Technologies’ approach to quantitative trading different from other hedge funds?
The firm employs a diverse team of researchers, mathematicians, and computer scientists who work together to develop, test, and refine mathematical models and algorithms.
Many hedge funds (especially quant hedge funds) don’t communicate much with the media or put out a lot of information publicly.
And their employees are often under NDAs, so it’s difficult for competitors to replicate.
How has the Medallion Fund maintained its impressive performance over the years?
The Medallion Fund’s consistent performance is due to its proprietary quantitative trading strategies, which are company IP.
The fund’s team refines its models and algorithms, ensuring that they stay ahead of market trends and competitors.
Additionally, the fund’s exclusivity and capped AUM help maintain its performance.
Can I invest in the Medallion Fund?
No. The Medallion Fund is not open to outside investors. The fund primarily serves the employees of Renaissance Technologies and their immediate families.
The AUM is capped to prevent overcrowding and dilution of returns. It’s much easier to have higher returns on a lower capital base than a high one, given the quantity of high-return opportunities thins out.
Nonetheless, there are other investment opportunities available in the quantitative trading space, and you can explore those options with the help of a financial advisor or broker.
What types of data do quantitative trading models use to make decisions?
Quantitative trading models rely on a wide range of data sources, including historical price data, financial statements, economic indicators, and even alternative data like weather patterns or social media sentiment.
This diverse set of data helps quantitative models identify hidden patterns and trends in the market.
Are there any risks or drawbacks associated with quantitative trading?
While quantitative trading has many advantages, there are some risks and drawbacks.
One potential risk is model risk, which occurs when an algorithm fails to accurately predict market behavior, leading to losses.
Another risk is overfitting, which happens when a model is too specific to historical data and doesn’t perform well in real-world conditions.
Overfitting is especially dangerous when the future is different from the past.
Additionally, quantitative trading can be susceptible to technological issues, such as software bugs or hardware failures, which can impact trading performance.
What types of mathematical models does RenTech use?
Renaissance Technologies employs a variety of mathematical models, including:
- statistical models for pattern recognition
- probability theories
- stochastic processes
- machine learning and nonlinear/non-parametric models, and
- algorithms for predictive analytics
These models analyze vast datasets to identify and exploit market inefficiencies and trends.