Quantitative Value Trading
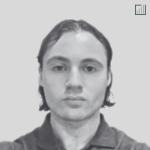
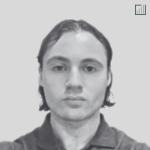
Quantitative value trading is a strategy that combines quantitative analysis techniques with traditional value investing principles to identify undervalued stocks and assets and achieve superior returns.
This approach leverages mathematical models, statistical methods, and computational power to enhance decision-making and optimize trading performance.
Key Takeaways – Quantitative Value Trading
- Data-Driven Analysis
- Quantitative value trading minimizes human bias by using mathematical models and statistical techniques to evaluate financial data and make trading decisions.
- Enhanced Identification of Undervalued Stocks
- This approach leverages traditional value investing metrics like P/E and P/B ratios (and others), combined with advanced quantitative analysis, to effectively identify stocks/assets trading below (or above) their intrinsic value.
- Scalable and Systematic Execution
- Quantitative strategies can be highly scalable (if sufficient capacity) and can be applied to large datasets across diverse markets.
- Helps facilitate efficient trade execution and portfolio management.
Fundamentals of Quantitative Value Trading
Value Investing Principles
Value investing is based on the idea that stocks have an intrinsic value, which can be estimated through fundamental analysis.
These types of investors look to buy stocks that are trading below their intrinsic value – and perhaps short those above their intrinsic value – believing that the market will eventually recognize and correct the undervaluation.
Key metrics used in value investing include price-to-earnings (P/E) ratio, price-to-book (P/B) ratio, and dividend yield.
Quantitative Analysis
Quantitative analysis involves the use of mathematical models and statistical techniques to analyze financial data and make trading decisions.
This can include regression analysis, machine learning algorithms, and factor models.
The goal is to identify patterns, trends, and relationships in the data that can inform trading strategies.
Value Metrics for Quantitative Value Investing/Trading
Quantitative value trading relies on a variety of financial metrics to identify undervalued stocks/assets with strong potential for returns.
Here is a list of value metrics a trader might use to produce a significant volume of metrics for analysis:
Fundamental Value Metrics
Price to Earnings Ratio (P/E)
Measures a company’s current share price relative to its per-share earnings.
Price to Book Ratio (P/B)
Compares a firm’s market value to its book value.
Price to Sales Ratio (P/S)
Analyzes a company’s stock price relative to its revenues.
Most useful for higher-growth companies with quality unit economics that are pre-earnings.
Price to Cash Flow Ratio (P/CF)
Compares the stock price to its operating cash flow per share.
Earnings Yield
The inverse of the P/E ratio, showing earnings as a percentage of the price.
Dividend Yield
Indicates how much a company pays out in dividends each year relative to its stock price.
Free Cash Flow Yield
Free cash flow per share divided by the stock price.
Enterprise Value to EBITDA (EV/EBITDA)
Compares enterprise value to earnings before interest, taxes, depreciation, and amortization.
Return on Equity (ROE)
Measures profitability by revealing how much profit a company generates with the money shareholders have invested.
Return on Assets (ROA)
Indicates how profitable a company is relative to its total assets.
Growth Metrics
Earnings Growth Rate
The annual compound growth rate of earnings from investments over a specified period.
Revenue Growth Rate
The rate at which a company’s revenue increases over a period.
Dividend Growth Rate
The annualized percentage rate of growth of a company’s dividend payments.
A key metric for those focused on dividends (a longer-term strategy).
Book Value Growth Rate
Measures the growth rate of the book value per share over a specific period.
Efficiency Metrics
Asset Turnover Ratio
Indicates how efficiently a company uses its assets to generate sales.
Inventory Turnover Ratio
Shows how many times a company’s inventory is sold and replaced over a period.
Receivables Turnover Ratio
Measures how efficiently a company collects its receivables.
Payables Turnover Ratio
Indicates how quickly a company pays off its suppliers.
Financial Health Metrics
Debt to Equity Ratio
Indicates the relative proportion of shareholders’ equity and debt used to finance a company’s assets.
Current Ratio
Measures a company’s ability to pay short-term obligations with its current assets.
Quick Ratio
Similar to the current ratio but excludes inventory from current assets.
Interest Coverage Ratio
Shows how easily a company can pay interest expenses on outstanding debt.
Altman Z-Score
A formula to predict the probability of a company going bankrupt.
Market Performance Metrics
Beta
Measures a stock’s volatility in relation to the overall market.
Sharpe Ratio
Indicates the average return minus the risk-free return divided by the standard deviation of return on an investment.
Treynor Ratio
Measures returns earned in excess of that which could have been earned on a riskless investment per each unit of market risk.
Sortino Ratio
Similar to the Sharpe ratio but uses downside deviation instead of standard deviation to focus on negative returns.
Quality Metrics
Piotroski F-Score
Combines nine accounting criteria to determine the strength of a firm’s financial position.
Beneish M-Score
A model to identify whether a company has manipulated its earnings.
Often monitored by short-sellers.
Ohlson O-Score
Predicts the probability of a firm’s bankruptcy within the next two years.
Custom Metrics
Economic Value Added (EVA)
Measures a company’s financial performance based on residual wealth calculated by deducting its cost of capital from its operating profit.
Magic Formula
Combines earnings yield and return on capital to rank stocks.
Graham Number
The maximum price that a defensive investor should pay for the stock.
Greenblatt’s Return on Capital
Measures the return on capital, adjusting for cash and liabilities.
Combining Metrics
Traders can create comprehensive models by combining these metrics, often weighted according to specific strategies. For instance:
- Composite Score – Combining P/E, P/B, and EV/EBITDA to create a single valuation score.
- Ranking Systems – Using a multi-factor model to rank stocks by various criteria such as value, growth, and quality.
- Regression Models – Building predictive models to forecast returns based on historical relationships between these metrics.
Leveraging these metrics and combining them in new ways allows traders to improve their quantitative value trading strategies.
Key Components of Quantitative Value Trading
Data Collection and Processing
Quantitative value trading relies heavily on data.
This includes financial statements, stock prices, leading economic indicators, and other relevant data sources.
Data must be collected, cleaned, and processed for accuracy and reliability.
High-quality data is necessary for developing effective models.
Model Development
Developing a quantitative model involves selecting appropriate variables, constructing the cause-effect mathematical relationships, and testing the model’s predictive power.
Traders will include many of the ones included above and combine them into new metrics.
Models may incorporate various factors such as earnings growth, return on equity, debt levels, and market sentiment.
Backtesting the model on historical data helps validate its effectiveness and refine its parameters.
Forward testing on synthetic data can also be used to stress test the portfolio on new/custom scenarios.
Risk Management
Risk management is a critical aspect of all trading.
This involves setting risk limits (e.g., position sizing limitations), diversifying the portfolio, and using techniques such as options or stop-loss orders to reduce potential losses.
Quantitative value trading is a highly engineered and mathematical strategy that tends to avoid concentration and is geared toward specific returns and risk parameters.
Quantitative models can also incorporate different risk factors – e.g., skew, kurtosis of probability distributions – to adjust position sizes and optimize the risk-reward balance.
Execution
Executing a quantitative value trading strategy requires efficient trade execution to minimize transaction costs and slippage.
This can involve the use of automated trading systems, algorithmic trading, and advanced order types.
Timely and accurate execution is essential for capturing the anticipated value from trades.
Advantages of Quantitative Value Trading
Objectivity
Quantitative value trading reduces human biases by relying on data-driven models and systematic processes.
This objectivity helps avoid emotional decision-making and allows consistency in the approach.
Scalability
Quantitative strategies can be scaled more easily than traditional value investing approaches.
Once a model is developed and validated, it can be applied to large datasets and diverse markets.
It also depends on the capacity of the strategy.
Enhanced Performance
By combining value investing principles with quantitative analysis, traders/investors can potentially identify undervalued stocks more effectively and achieve superior returns.
The increasing use of machine learning models and computational power better enables the detection of subtle patterns and relationships that may be missed by traditional methods.
Challenges of Quantitative Value Trading
Model Risk
Quantitative models are based on historical data and assumptions, which may not always hold true in the future.
Model risk arises when the model’s predictions are inaccurate or when the future is different from the past but the models are optimized on past data.
Data Quality
The effectiveness of quantitative value trading depends on the quality of the data used.
Inaccurate or incomplete data can lead to erroneous conclusions and poor trading decisions.
Complexity
Developing and maintaining quantitative models requires specialized knowledge and expertise in finance, mathematics, and programming.
The complexity of these models can also make them difficult to understand and manage (“black box”).
This is especially true for individual traders or smaller firms.
Let’s look at some trades involving quantitative value trading.
To illustrate quantitative value trading, let’s consider a hypothetical example involving two stocks: Stock A and Stock B.
We will outline the steps for putting on the trade, including specific amounts and processes involved.
Example Trade
Step 1: Data Collection and Initial Screening
Collect Data
Gather financial data for Stock A and Stock B, including:
- price-to-earnings (P/E) ratios
- price-to-book (P/B) ratios
- earnings per share (EPS)
- revenue growth, and
- other relevant metrics of the trader’s choice
For this example, we’ll assume the following data:
- Stock A: P/E ratio = 8, P/B ratio = 0.9, EPS = $5, revenue growth = 5% per year
- Stock B: P/E ratio = 20, P/B ratio = 3.5, EPS = $2, revenue growth = 2% per year
Initial Screening
Use quantitative filters to identify undervalued stocks based on value investing principles.
In this case, Stock A appears to be undervalued relative to Stock B based on its lower P/E and P/B ratios.
Step 2: Model Development and Validation
Develop Model
Construct a quantitative model that incorporates key value metrics.
For simplicity, let’s use a basic value score combining P/E and P/B ratios.
The formula for the value score could be:
- Value Score = (P/E ratio * 0.5) + (P/B ratio * 0.5)
Calculate Scores
Apply the model to calculate value scores for both stocks:
- Stock A Value Score: (8 * 0.5) + (0.9 * 0.5) = 4.45
- Stock B Value Score: (20 * 0.5) + (3.5 * 0.5) = 11.75
Validation
Backtest the model using historical data to be sure it accurately identifies undervalued stocks and predicts positive returns.
Assume backtesting results support the model’s validity.
Step 3: Trade Execution
Position Sizing
Determine the amount to trade based on portfolio allocation and risk management principles.
Assume a total investment capital of $100,000, with 50% allocated to this strategy.
Allocate Funds
- Total Allocation = $50,000
- Stock A Allocation = $50,000 (since it is identified as undervalued)
Trade Placement
- Current Price of Stock A = $40 per share
- Number of Shares to Purchase: $50,000 / $40 per share = 1,250 shares
- Execute Trade: Place a buy order for 1,250 shares of Stock A at $40 per share.
Step 4: Risk Management and Monitoring
Set Stop-Loss Order
To manage risk, set a stop-loss order at 10% below the purchase price. For Stock A, this would be:
- Stop-Loss Price: $40 – ($40 * 0.10) = $36 per share
Monitor Performance
Regularly review the performance of Stock A, re-evaluate its value metrics, and adjust the position as needed based on new data and market conditions.
Rebalance Portfolio
Periodically rebalance the portfolio to maintain the desired allocation and manage overall risk.