Artificial Intelligence in Currency Trading & Policy Forecasting
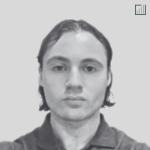
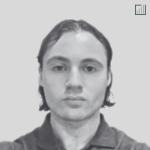
Currency markets today are now shaped not just by interest rates and macro data, but by algorithms that learn, adapt, and react faster than any human can.
What was once experimental is now routine, embedded in the architecture of both institutional trading desks and central bank research units.
In the past, AI and automation merely replicated what humans could do at higher speeds (e.g., execute trades). Now, it reveals relationships that conventional models can’t see, which transforms vast streams of unstructured data into insights that traders can actually use.
It helps decode complexity into probabilities and signals with a level of nuance that traditional econometrics can’t reach.
This article looks at how artificial intelligence is transforming currency trading through machine learning, sentiment analysis, and predictive modeling.
Key Takeaways – Artificial Intelligence in Currency Trading & Policy Forecasting
- What used to be high-end experimental modeling is now a daily necessity for institutional FX trading desks.
- AI models detect nonlinear patterns between macro data, market “sentiment,” and price action. It offers insights beyond traditional econometrics.
- NLP-powered algorithms track important documents and text data (e.g., central bank tone shifts), helping traders front-run policy pivots invisible to retail eyes.
- Reinforcement learning improves trade execution, dynamically routing orders based on real-time liquidity, slippage, and venue latency.
- AI builds predictive macro models from real-time data – from satellite imagery to electricity usage – giving traders a faster, more granular edge.
- We’re at a time where motivated individual traders can now leverage AI and machine learning to enhance their edge, using models to support their strategic decisions.
Pattern Recognition and Nonlinear Insight
Machine Learning and Complex Market Relationships
Pattern recognition is the foundation.
Machine learning (ML), particularly neural networks, excels at identifying nonlinear connections between disparate data sets.
Unlike traditional linear regression models, ML systems uncover subtle, dynamic relationships between commodity prices, bond spreads, capital flows, political risk, and other factors that go into markets.
For example, a deep learning model might detect that a rise in iron ore prices, paired with tightening US-Australian yield differentials and a certain tone in Chinese economic reports, precedes upward pressure on AUD/USD.
These models are always adapt, learning from new data as conditions shift – something static models can’t match.
Natural Language Processing and Sentiment Decoding
Central Bank Speech Analysis and Narrative Shifts
Financial analysis has traditionally been all about numbers.
Now, one of the most powerful AI applications in FX trading is Natural Language Processing (NLP).
For example, advanced NLP algorithms can now detect nuance in central bank communications – not just policy statements, but also informal remarks, testimonies, Q&A sessions, and leaked memos.
When the ECB president slightly shifts tone on “transitory inflation,” these models flag it in real time.
NLP tracks deviations from baseline sentiment established across months of prior speeches, enabling institutional desks to detect pivots before they hit headlines.
These systems digest tone, context, and emphasis with a level of granularity far beyond keyword-based sentiment tagging.
Fine-Grained Sentiment and Emotional Signal Weighting
Modern AI sentiment engines don’t merely tag texts as “positive” or “negative.”
They interpret degrees of confidence, caution, or alarm.
In other words, instead of binary word labels, a wide distribution that encompasses nuance. This matters in FX.
A cautiously optimistic tone from Chinese officials may drive the Australian dollar, while a confident hawkish stance from the Bank of England might lift the pound regardless of soft macro data.
AI distinguishes between tentative encouragement and strong conviction and translates that into probabilistic market outcomes.
High-Frequency Trading and Smart Execution
Millisecond Advantage and Reinforcement Learning
In high-frequency trading (HFT), AI is used to optimize execution down to the millisecond.
Reinforcement learning algorithms power smart order routers that dynamically select trading venues based on real-time liquidity, latency, and price slippage.
These systems learn from their own performance, constantly improving execution efficiency.
For large market makers, this leads to better spreads, faster fills, and lower market impact – advantages that compound over thousands of trades per day.
Many interbank desks now rely on AI-enhanced routing just to stay competitive in narrow-margin environments.
Real-Time Macro Forecasting
Moving Beyond Static Economic Models
AI’s impact extends beyond trading desks into macroeconomic forecasting.
Traditional models – often based on backward-looking data and linear assumptions – struggle to keep pace with modern economies.
In contrast, machine learning models can incorporate diverse, high-frequency indicators to forecast economic trends in real time.
Electricity usage, traffic patterns, retail footfall, shipping flows, and social media sentiment are just some of the inputs being used to construct nowcasting models for GDP, inflation, or employment.
These systems are dynamic and self-updating. They’re often termed “high-frequency indicators.”
In turn, this enables traders and policymakers to see shifts earlier than quarterly economic releases allow.
Central Banks as AI Experimenters
Institutions like the European Central Bank and the Bank of Canada have begun experimenting with supervised learning models to improve forecasting accuracy.
Research shows that these models often outperform autoregressive benchmarks in predicting inflation, unemployment, or even short-term exchange rate movements.
Emerging Markets: Filling Data Gaps with Proxy Modeling
Satellite Imagery and Alternative Data
In emerging markets, where official data is often delayed or unreliable, AI is proving highly useful.
Traders use satellite imagery – such as night-time lights or port congestion – to estimate economic activity.
ML models turn these unconventional data sources into reliable proxies for industrial output, energy demand, or consumption levels.
For example, AI may detect rising night-light intensity in Vietnamese industrial zones, signaling economic acceleration and triggering a shift in VND asset positioning.
These proxy-based systems allow traders to act ahead of official releases, pricing risk more precisely in opaque markets.
Flow-Based Models and Positioning Dynamics
Learning Capital Flow Patterns
Institutional capital flows (e.g., those from pension funds, sovereign wealth funds, and insurance firms) are sticky, slow-moving, and often mean-reverting.
For example, pension funds typically have allocation targets and will trim or add depending on how far away from the target they are.
AI models trained on flow data can detect when market positioning is stretched and vulnerable to reversal.
If, for instance, euro long positioning reaches an extreme and sentiment peaks, an ML model might flag downside risk despite strong macro data.
This type of model doesn’t replace other forms of analysis but adds a layer of statistical flow awareness that enhances entry and exit timing.
Forecasting Policy Shifts with Machine Learning
Policy Probability Models and Market Mispricing
Machine learning is also being used to estimate the probability of monetary policy changes.
By ingesting thousands of inputs – e.g., labor slack, inflation expectations, commodity prices, real wages, fiscal policy trajectories, and external financial conditions – AI models assign confidence levels to rate hikes, cuts, or holds.
These models measure how markets are priced versus what should be expected.
It basically compares a trader’s distribution of what’s expected versus the market-discounted distribution.
When a divergence occurs – such as markets pricing a cut when the model sees rising inflation pressure – it creates potential trading opportunities in FX and rates markets.
The Limits and Risks of AI in Currency Markets
Regime Shifts and Model Blindness
AI models are only as good as the data they’re trained on.
When central banks change their frameworks – as the Fed did with Average Inflation Targeting in 2020 – models built on old patterns can misfire.
Recognizing these shifts requires human judgment.
That’s why the most effective trading systems today are hybrid:
- AI handles signal extraction and pattern learning…
- …while experienced traders apply discretion and interpret matters that aren’t priced into algorithms.
For example, in response to the April 2025 tariff announcement, algorithmic models initially underreacted, but as discretionary traders entered and interpreted the broader implications, market moves accelerated beyond what the algorithms had anticipated or were equipped to process.
The Explainability Challenge: From Black Boxes to Transparency
Deep learning models, particularly neural networks, often lack transparency.
They can forecast accurately without revealing the logic behind their predictions.
In environments where portfolio managers must justify decisions or comply with regulations, this opacity is a liability.
To handle this, quants increasingly use tools like SHAP values (SHapley Additive exPlanations) and LIME (Local Interpretable Model-agnostic Explanations), which clarify which variables influenced a model’s output.
These interpretability layers allow for scenario testing and enhance trust in AI-driven decision support systems.
Retail Traders and AI Access
The Growing Divide Between Retail and Institutional Use
AI in retail FX trading usually takes the form of signal services, black-box expert advisors (EAs), or paid subscriptions.
Some retail traders experiment with neural networks or use AI tools to parse Commitments of Traders (COT) reports and news feeds, but they often lack access to quality data, professional infrastructure, or scalable execution systems.
Most retail systems remain opaque, poorly backtested, or overfitted.
Discretionary traders often continue to rely more on technical analysis – e.g., chart patterns, Fibonacci levels, or indicator convergences/divergences – than AI.
This highlights a growing capability gap unless AI tooling becomes more democratized and user-friendly.
Central Banks and Systemic Risk Monitoring
Using AI to Detect Financial Stress and Market Fragility
Central banks are also turning to AI to monitor systemic risk.
During events like the March 2020 liquidity crunch, machine learning models helped identify correlations and contagion channels between credit and FX markets.
These insights influenced coordinated actions, such as the use of dollar swap lines.
Although central banks are cautious about using AI for policymaking, they increasingly rely on it for internal research, risk surveillance, and communication analysis, especially in volatile global environments.
Model Crowding and the Risk of Algorithmic Herding
Feedback Loops and Flash Crashes
AI systems, if widely used and similarly trained, can lead to “model crowding.”
When multiple institutions rely on overlapping models, they may all reach the same conclusions and enter or exit trades simultaneously.
This behavior has been linked to flash crashes, such as the 2016 British pound plunge and the 2019 yen spike.
As liquidity becomes more concentrated in AI-driven execution systems, market fragility during shocks becomes more of an unknown.
Traders and regulators now actively monitor algorithmic herding as a systemic risk.
The Future: Augmentation, Not Automation
The traders and analysts who use AI most effectively understand its limits.
They don’t view it as a replacement for human judgment – but as a force multiplier.
AI shines at parsing complexity, surfacing signals, and adapting to high-dimensional data.
But it falters during regime shifts (when the future is different from the past), seemingly unprecedented events (as far as data goes), geopolitical shocks, and basically anything that’s outside of its training.
The best discretionary macro traders treat AI as an extension of their thinking. In other words, a tireless research assistant that scans everything, learns fast, and never sleeps, but still needs direction.
AI’s edge will grow not from autonomy, but from the synergy between machine precision and the expert human wisdom that handles its strategic and creative direction.
For example, machines can calculate instantly how tariff rates mathematically impact asset prices. But it still takes a human to understand the driving forces that make tariff policies popular in certain macro, political, and geopolitical contexts and other policies that are derivative of the same circumstances.
Most Common AI Models Used in Currency Trading
Let’s look at some of the most common AI models used in FX trading:
Neural Networks (NNs)
Neural networks are widely used in currency trading for their ability to detect nonlinear relationships between variables like macroeconomic indicators, price movements, and sentiment.
Deep learning models, such as LSTM (Long Short-Term Memory) networks, can process sequential time-series data, making them especially useful for predicting FX trends.
These models adapt as new data arrives, making them valuable in fast-changing markets.
They can also be trained on vast datasets that include structured data, sentiment signals, and even audio transcripts, allowing for highly multidimensional modeling of currency dynamics.
Random Forests
Random forests are ensemble learning models that use multiple decision trees to improve predictive accuracy.
They’re popular in FX forecasting for their robustness against overfitting and ability to handle noisy datasets.
Traders often use them for classification tasks like predicting directional moves or ranking potential trade setups.
Their built-in feature importance scores also help identify which inputs – such as yields, spreads, or geopolitical indicators – contribute most to forecast outcomes.
Support Vector Machines (SVMs)
SVMs are used to classify complex data with clear boundaries, such as whether a currency pair is likely to trend or reverse.
While less flexible than neural networks, they’re effective when training data is limited or when interpretability is a priority.
Their performance tends to shine in structured data environments.
Gradient Boosting Machines (GBMs)
Gradient boosting models like XGBoost or LightGBM are popular in FX for their speed and precision in supervised learning tasks.
These models work well with tabular data and are often used for ranking signals or forecasting short-term price direction.
They’re most useful when combining diverse features such as sentiment, flow data, and macro indicators.
K-Means Clustering
K-means is an unsupervised learning method used to group similar patterns or market regimes.
In FX, it can help identify periods of high or low volatility, cluster currency behavior based on macroeconomic conditions, or segment trading days by market structure.
It’s often used for preprocessing or exploratory analysis.
Reinforcement Learning (RL)
Reinforcement learning is increasingly used in smart execution systems and algorithmic trading strategies where adaptive decision-making is important.
These models learn optimal actions – e.g., trade entries, exits, or order routing – by receiving rewards or penalties from market feedback.
Natural Language Processing (NLP) Models
NLP models such as BERT, and custom-trained transformers are used to extract insight from central bank speeches, economic reports, and financial news.
They help decode tone, sentiment, and shifts in policy language that may impact currency valuation.
These models turn unstructured text into quantifiable inputs for trading algorithms.
Bayesian Models
Bayesian models are favored for probabilistic forecasting, especially when estimating policy decisions or risk scenarios.
They allow traders to incorporate prior beliefs and update probabilities as new data comes in.
This approach provides transparency and a framework for uncertainty management in macro forecasting.
They’re also useful for scenario analysis, helping traders assess how likely different economic outcomes are under changing assumptions.
Bayesian inference is helpful in low-data or regime-shift environments, where traditional models may fail to recalibrate effectively.
Autoencoders
Autoencoders are used for anomaly detection and dimensionality reduction in large FX datasets.
They learn to compress and reconstruct data, making them useful for identifying rare market conditions or cleaning noisy features.
Some desks use them to pre-process inputs before feeding them into trading models.
Decision Trees
While simple, decision trees remain useful for building interpretable rule-based FX models.
They’re often embedded in more complex ensemble methods like random forests or boosting models.
Traders sometimes use them to prototype logic for conditional strategies.
K-Nearest Neighbors (KNN)
KNN is occasionally used in FX to find historical market conditions that are similar to the current one.
While computationally expensive and less common in real-time systems, KNN can be valuable for backtesting and constructing analog-based trading strategies.
Related
- Currency Trading Strategies Used by Professionals
- How to Design an Algorithmic Trading System for Currencies
- Encyclopedic List of Quant Methods & Techniques in Finance
How Can Individual Traders Integrate AI and Machine Learning into Their Trading?
An individual trader can experiment with machine learning platforms like Python’s scikit-learn or no-code tools to build simple models using historical price and indicator data.
With today’s tools, it’s becoming easier to do this faster. It’s less about “do you know how to code?” and more “what do you want to code?”
Most importantly, traders should focus on integrating AI as a decision-support system – not a replacement – by combining its insights with their own goals and strategic judgment.