How Do Professional Traders Think and Trade?
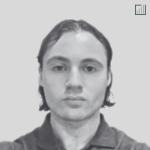
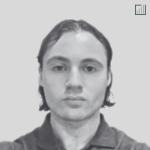
How do professional traders think and trade?
Everyone has different perspectives, but it’s by and large a function of their philosophies, beliefs, training, goals, risk tolerance, and analysis.
In financial markets, traders and investors rely on various indicators to make informed decisions.
These indicators are often referred to as market signals, and provide insight into market trends, potential opportunities, and risks.
For discretionary traders, it’s based on their own analysis, beliefs, processes, and intuition. They tend to not rely on algorithms or use them in limited ways.
Discretionary traders also make up less liquid markets, where there is less competition and systematic trading is less relevant.
For systematic traders, where increasingly more trading has gone, it’s based on taking these thought processes, writing them down, stress testing them, and writing them what’s valid into algorithms that can be applied in the markets and environments in which they’re relevant.
This article focuses most on the concept of market signals in trading, their types, and how they can be effectively used to improve trading strategies.
Key Takeaways – How Do Professional Traders Think and Trade?
- Professional traders rely on structured market signals to make quality decisions.
- Different types of signals include:
- value
- fundamental momentum
- inter-market action
- capital flows
- Combining various signals and using algorithms can improve trading strategies.
- Traders look for signals that are ideally uncorrelated or negatively correlated to improve the reward-to-risk ratios in their portfolio.
What Are Market Signals?
Market signals are indicators or pieces of information that traders use to predict future market behavior.
These signals can come from various sources, including economic data, technical indicators, fundamental analysis, and market sentiment.
By interpreting these signals correctly, traders try to make more accurate predictions about price movements and market trends.
Types of Market Signals
By and large, there are four main categories of signals that institutional traders tend to use.
Think of these as four different people having different perspectives on how to trade markets.
Who’s right?
They all have valid perspectives, so it’s important to consider them all and how they might fit into your approach.
1) Value Signals
Value signals are primarily used for long-term strategies.
While they may not provide much for short-term trading, they’re important for understanding the intrinsic worth of an asset.
What’s the intrinsic value of a stock, for example?
It’s a series of cash flows discounted back to the present.
From those calculations, traders can then determine whether the value of an asset makes sense.
For instance, you expect that an asset will pay you $1,000 per year. What would you pay for that?
Your answer depends on your discount rate. Those with a higher discount rate, will cite a cheaper price; those with a lower discount rate will be willing to pay more.
Key aspects of value signals:
- Price-to-earnings (P/E) ratio
- Book value
- Dividend yield supported by earnings
- Cash flow analysis
Value signals help traders identify undervalued or overvalued assets, which can be useful for long-term strategies.
However, value will not help you much over the next 6-12 months. It will matter a little bit, but value investors’ frustration tends to be a common theme during raging bull markets or bubbles.
For longer-term investors, it tends to be a quality approach assuming the analysis is good. For shorter-term traders, it’s less used because value considerations naturally have a less relevant impact on prices.
This is because there are various other approaches and realities to how assets move and are priced.
Related: Quantitative Value Trading
2) Fundamental Momentum Signals
Fundamental momentum signals focus on changes in the underlying economic and financial conditions that affect asset prices.
These signals are based on the movement of fundamental factors that drive market valuations.
Key components of fundamental momentum signals:
- Changes in discount rates
- Shifts in risk premiums
- Alterations in discounted growth expectations
- Inflation trends relative to discounted expectations
We explained this more in our article on the big four variables that impact asset class movements at the macro level.
There is also overlap between the forces mentioned here.
For example, if interest rates rise, the fundamental value of assets may fall due to the present value effect.
This is because higher interest rates reduce the present value of future cash flows, potentially leading to lower asset valuations.
This is both a value consideration, momentum consideration, and blends in with our next two as well.
3) Inter-market Action Signals
Inter-market action signals examine the relationships between different financial markets and how they influence each other.
Understanding these connections can provide insights into potential market movements.
Examples of inter-market relationships:
- Interest rates and all asset types
- Bond yields and stock prices
- Commodity prices and currency exchange rates (e.g., if oil goes up, how does that impact the yen (oil importer) or Canadian dollar (oil exporter)?)
- Global equity market correlations
- Sector rotation within equity markets
By analyzing these relationships, traders can gain a broader perspective on market dynamics and potential opportunities or risks across different asset classes.
4) Capital Flow Signals
Capital flow signals focus on the movement of money in and out of markets.
This gives traders insights into the behavior of different market participants.
Key aspects of capital flow signals:
- Identification of major buyers and sellers
- Analysis of participant motivations (e.g., value, momentum, regulatory, buying/selling for non-economic reasons, etc.)
- Assessment of participant balance sheets – how big are the players in these markets?
- Understanding of funding sources and credit conditions. Where do they get their money and credit?
Basically it boils down to who’s selling and who’s buying and for what reasons.
Capital flow signals help traders understand the underlying forces driving market movements beyond long-term cash flow effects.
For instance, large institutional investors may make decisions based on factors such as regulatory requirements or portfolio rebalancing, which can create short-term market distortions.
Combining Signals for Effective Trading Strategies
To develop robust trading strategies, it’s essential to combine various market signals effectively.
This process involves several key steps:
Signal Quality Assessment
Not all signals are created equal.
Traders must evaluate the quality of each signal based on its historical accuracy, relevance to current market conditions, and potential impact on asset prices.
Risk Budget Allocation
Determining how much weight to assign to each signal within a trading strategy is important. This process involves considering the following factors:
Signal correlation
Signals that are highly correlated may receive less weight to avoid overexposure to a single theme.
For instance, directional exposure within a single asset class tends to be tightly correlated.
Treasury bonds of various maturities tend to highly correlate. Stocks tend to highly correlate with each other.
Diversification value
Uncorrelated signals may receive more weight to enhance portfolio diversification.
Signal reliability
More reliable signals may be given greater importance in the overall strategy.
More causal factors lining up
For example, let’s say a trading firm, through their research, has identified 250 different variables – of varying importance and weighting within their algorithm – that influence bond yields.
If at a certain point 175 of them line up (70% of them equal-weighted), then that’s likely to give a statistically more reliable signal than when only 125 of them line up (50% of them).
This is especially more important if the importance-weighted version is, say, 85% vs. just 55%.
Beginning traders, on the other hand, are more likely to trade based on very limited data based on subjective gut judgments that they don’t stress test and without appropriate risk controls.
Overall
Think of it this way:
Signal * Quality * Risk budget = Weighting in portfolio
Exposure Management
The combination of weighted signals contributes to the desired exposure in a trading strategy.
This exposure represents the overall market position based on the interpretation of various signals.
Selective Focus
It’s important to note that traders don’t need to have a view on every aspect of the market. It’s impossible and you can’t.
Focusing on areas where one has a competitive advantage or unique insights can be more effective than trying to analyze every possible signal.
This goes for both systematic and discretionary traders.
The Role of Algorithms in Signal Processing
As financial markets become increasingly complex, algorithms become more important in processing and interpreting market signals.
The analysis approaches are also changing. Machine learning is becoming more important over time.
Advantages of Algorithmic Signal Processing
- Speed – Algorithms can analyze vast amounts of data and generate signals much faster, more accurately, and cheaper than human traders.
- Consistency – Well-designed algorithms apply trading logic consistently, without being influenced by emotions or biases.
- Scalability – Algorithmic systems can monitor and trade multiple markets simultaneously. A human brain might be more creative, but it lacks the bandwidth and raw calculation ability of the computer.
- Continuous improvement – Algorithms can be refined and optimized based on new data and understanding.
Universal Applicability
Algorithms with well-defined logic can be applied across various markets and asset classes.
This universal applicability allows traders to leverage their strategies across different geographies and market segments without requiring in-depth knowledge of each specific market.
Of course, expert judgment has to be made.
If you have a USD-specific trading algorithm, you might not apply the same logic to the drivers of the Thailand baht (THB).
Focus on Missing Elements
When core trading logic is embedded in algorithms, traders can focus on identifying and incorporating new factors that may not be captured by existing models.
This might include:
- Geopolitical tensions
- Changes in trade patterns
- Regulatory shifts
- Emerging market trends
By continuously learning and adapting, traders can enhance their algorithms to account for these additional factors, which can lead to more effective trading strategies.
Algorithms can be continually improved while humans tend to reach a certain level of competence and then stagnate or even decline.
The Compounding Effect of Knowledge in Algorithmic Trading
Just as investments can compound over time, so too can the knowledge and effectiveness of algorithmic trading systems.
This compounding effect occurs through several mechanisms:
Continuous Learning
As algorithms process more data and encounter diverse market conditions, they can be refined to improve their accuracy and effectiveness – depending on how they’re set up.
Efficiency Gains
Algorithmic trading systems become more efficient over time as they are optimized for speed and accuracy.
This increased efficiency can lead to better execution of trades and improved overall performance.
Error Reduction
Well-designed algorithms are less prone to errors compared to human traders.
As these systems are refined, the likelihood of errors decreases further, leading to more consistent and reliable trading outcomes.
Scalability
As algorithmic trading systems improve, they can be applied to a broader range of markets and asset classes.
This scalability allows traders to leverage their knowledge and strategies across diverse opportunities.
A single human brain can only keep track of so much.
The system can be immediately aware of certain things that a human trader won’t.
Challenges & Considerations
There are also challenges and considerations to keep in mind:
Signal Reliability
Not all market signals are equally reliable, and their effectiveness can vary over time.
The performance of different signals needs to be monitored and adjust their strategies accordingly.
Overfitting Risk
When developing algorithmic trading systems, there’s a risk of overfitting models to historical data if that’s what they’re based on.
This can lead to strategies that perform well in backtests but fail or perform poorly in real-world trading conditions.
Are the algorithms logical or are they simply data-mined?
Market Adaptability
Financial markets are dynamic and constantly evolving.
Traders must ensure that their signal-based strategies can adapt to changing markets and new sources of information.
Technological Dependencies
Reliance on algorithmic trading systems introduces technological risks, such as system failures or connectivity issues.
Risk management and backup systems are essential to reduce these risks.
How Discretionary Traders Can Come Out Ahead
Being systematic and doing it well requires a certain level of sophistication.
But not everyone can or wants to trade that way.
Discretionary traders can carve out a niche in today’s algorithm-dominated markets by focusing on areas where human judgment and specialized knowledge provide a competitive edge.
Here’s how they can approach this:
Discretionary Traders Can Excel by…
- Specializing in specific sectors or asset classes where they have deep expertise.
- Cultivating niche knowledge that’s not easily quantifiable or accessible to algorithms.
- Focusing on qualitative factors that algorithms struggle to assess, such as:
- Quality of company management
- Corporate culture and employee satisfaction
- Potential for innovation and adaptability
- Leveraging private information or insights gained through industry connections.
- Analyzing geopolitical events and their potential market impacts. (This is still not represented well in algorithmic trading, but will get better over time.)
- Identifying emerging trends before they’re reflected in widely available data.
Intuition is often cited as a competitive advantage of man over machine, but has to be honed through years of training and preparation.
Strategic and Passive
When it comes to active trading, algorithms have increasingly large advantages.
Discretionary traders can adopt a more strategic, passive, and balanced approach where the playing field becomes more level:
- Focusing on longer-term trades/investments where you’re simply capturing risk premiums and not tactically trying to outperform.
- Avoiding higher-frequency trading and other areas where algorithms’ raw calculation capacity and execution can’t be matched.
- Complementing algorithmic strategies rather than competing directly with them.
- Developing hybrid approaches that combine human judgment with algorithmic tools.
- Concentrating on less liquid or more complex assets where algorithmic trading is less prevalent and there’s less competition.
Emphasizing these human-centric advantages and adopting a thoughtful approach to market participation can help discretionary traders can maintain relevance and profitability in an increasingly systematic trading environment.
Conclusion
Professional traders prioritize identifying and using structured signals that enable them to consistently replicate and optimize their performance.
By combining various types of signals – e.g., value, fundamental momentum, inter-market action, and capital flows – traders can develop trading strategies that account for multiple aspects of market behavior.
The integration of algorithmic trading systems has changed the way market signals are processed and used.
These systems offer advantages in terms of speed, consistency, and scalability, allowing traders to apply their strategies across markets and asset classes.
However, successful signal-based trading requires ongoing learning and adaptation.
Traders have to continually refine their algorithms or processes, incorporate new sources of information, and remain vigilant to changing market dynamics.
The ability to effectively interpret and act on market signals will remain a key differentiator for successful traders.