Creative Trading Strategies
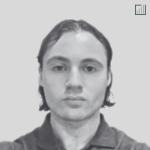
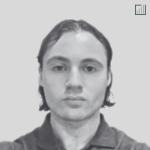
Traders are constantly seeking innovative ways to gain an edge.
Creative trading strategies go beyond conventional methods, leveraging unique analysis methods, technological advancements, and unconventional data sources to obtain information and identify cause-effect linkages.
Here we look at various creative approaches to trading that can potentially improve returns and manage risk more effectively.
Key Takeaways – Creative Trading Strategies
- Sentiment-Based Trading
- Social Media Analysis
- News Sentiment Analysis
- Alternative Data Trading
- Satellite Imagery Analysis
- Web Scraping for Market Insights
- Geolocation Data Analysis
- Algorithmic Pattern Recognition
- Fractal Analysis
- Machine Learning for Pattern Detection
- Behavioral Finance Strategies
- Contrarian Trading Based on Market Psychology
- Momentum Trading with Psychological Triggers
- Overreaction Strategy
- Herding Behavior Exploitation
- Cross-Asset Correlation Trading
- Inter-Market Analysis
- Pairs Trading Across Asset Classes
- Event-Driven Strategies
- Predicting and Trading Around Black Swan Events
- Calendar Anomaly Trading
Sentiment-Based Trading
Social Media Analysis
One of the most innovative developments in recent years is the use of social media sentiment to inform trading decisions.
This strategy involves:
- Monitoring platforms like Twitter/X, Reddit, and StockTwits for discussions about specific stocks or market trends.
- Using natural language processing (NLP) algorithms to analyze the sentiment of posts and comments.
- Generating trading signals based on sudden shifts in sentiment or unusual activity levels.
Traders using this strategy might, for example, take a long position in a stock that’s receiving an unusually high volume of positive mentions on social media platforms.
NLP development received a boost due to the GameStop meme stock rally in 2021.
News Sentiment Analysis
Similar to social media analysis, this approach examines news articles and press releases to determine market sentiment.
Advanced algorithms can process thousands of news items in real-time, assessing their potential impact on asset prices.
Traders can use this information to:
- Identify potential market-moving events before they’re fully reflected in prices.
- Adjust their positions based on the overall sentiment surrounding a particular asset or sector.
- Develop contrarian strategies by identifying situations where news sentiment might be overly optimistic or pessimistic.
Alternative Data Trading
Satellite Imagery Analysis
This innovative strategy uses satellite imagery to gather insights about economic activity. For example:
- Monitoring parking lot occupancy at retail stores to estimate sales figures.
- Tracking oil tanker movements to predict changes in oil supply and demand.
- This is standard for a lot of commodities to gain an edge.
- Assessing crop yields by analyzing agricultural land from space.
Traders can use this information to make informed decisions about investments in retail, energy, or agricultural sectors before official reports are released.
Web Scraping for Market Insights
Web scraping involves automatically extracting large amounts of data from websites.
Traders use this technique to:
- Track product prices and inventory levels on e-commerce platforms.
- Using Google search data trends to understand emerging trends and approximate product/service sales.
- Monitor job listings to gauge the health of various industries.
- Analyze customer reviews to understand brand sentiment and product popularity.
This data can provide early signals about a company’s performance or broader economic trends.
Geolocation Data Analysis
Geolocation data from smartphones and other devices can provide insights into consumer behavior and economic activity.
Movement is used as a proxy for economic activity, and thus money and credit flows that will impact market pricing.
This strategy involves:
- Tracking foot traffic to retail stores, malls, and restaurants to gauge consumer spending habits.
- Analyzing commute patterns to understand workforce movements and the health of different economic sectors.
- Monitoring visits to industrial sites to predict production levels and supply chain activities.
Via real-time location data, traders can make informed predictions about various sectors’ performance.
For example, an increase in foot traffic to a particular retail chain can indicate strong sales performance, while a decline in visits to manufacturing plants might signal production slowdowns.
Algorithmic Pattern Recognition
Fractal Analysis
Fractal analysis in trading is based on the idea that market patterns repeat themselves at different scales.
Traders using this approach:
- Identify recurring geometric patterns in price charts.
- Use these patterns to predict potential market reversals or continuations.
- Combine fractal analysis with other technical indicators for stronger trading signals.
- It’s best to avoid over-relying on any one form of analysis.
Machine Learning for Pattern Detection
Machine learning algorithms can identify complex patterns in market data that might be invisible to discretionary traders.
Well-designed algorithms can calculate faster, more accurately, and make less emotional decisions than humans.
This approach involves:
- Training models on historical price data, volume, and other relevant factors.
- Using these models to recognize emerging patterns in real-time market data.
- Generating trading signals based on the probability of certain price movements.
As machine learning models advance over time, they can incorporate an increasing number of variables, potentially leading to more accurate predictions.
Behavioral Finance Strategies
Contrarian Trading Based on Market Psychology
This strategy leverages insights from behavioral finance to identify situations where market participants might be acting irrationally.
Contrarian traders:
- Look for signs of extreme fear or greed in the market.
- Look for extrapolated conditions priced into markets that aren’t likely.
- Take positions opposite to the prevailing market sentiment.
- Profit when market psychology shifts back towards a more balanced state.
For example, a trader might buy stocks during a market panic, betting that the selloff is overdone and a rebound is likely.
Momentum Trading with Psychological Triggers
While traditional momentum trading focuses on price trends, this creative approach incorporates psychological factors:
- Identify key psychological price levels (e.g., round numbers, all-time highs).
- Monitor trading volume and price action as these levels are approached.
- Look at option volume at various price levels to help understand likely pinning zones (i.e., option activity dictating trading activity in the underlying due to hedging activity).
- Enter trades based on the market’s reaction to these psychological triggers.
This strategy attempts to capitalize on the self-fulfilling nature of certain psychological barriers in the market.
Overreaction Strategy
This strategy takes advantage of the tendency for markets to overreact to news, both positive and negative.
Traders can understand that such overreactions often correct themselves, such that they can position themselves to benefit from the subsequent price adjustments.
- Identify significant news events that cause sharp price movements.
- Analyze historical data to determine typical overreaction patterns in similar situations.
- How long does the overreaction take (e.g., 7 minutes post-release) and when does it retrace to more fundamental levels once the data is digested?
- Take a position contrary to the initial price movement, expecting a reversion to the mean.
For example, if a stock drops significantly due to a negative news event but the fundamentals remain strong, a trader might buy the stock anticipating a recovery as the market corrects its overreaction.
Herding Behavior Exploitation
Herding behavior occurs when traders follow the actions of a larger group, often leading to mispricing in the market.
This could be due to hype, emotion, and promotion.
These are often called “story stocks” where the price is mostly based on narratives rather than the actual reality of the business.
This strategy involves identifying and exploiting these trends:
- Monitor large-scale buying or selling activity that seems driven by herd mentality rather than fundamentals.
- Analyze social media, news sources, and trading volumes to identify herd behavior.
- Take a position opposite to the herd, expecting that the irrational behavior will eventually correct itself.
For instance, if a stock’s price rises sharply due to hype on social media without substantive fundamental support, a trader might short the stock – anticipating a decline once the hype subsides and the herd moves on.
Naturally, this type of “greater fool” price action is most common in equities and less so in bonds, commodities, currencies, and other asset classes.
Cross-Asset Correlation Trading
Inter-Market Analysis
This strategy involves analyzing relationships between different asset classes to inform trading decisions.
Traders might:
- Monitor correlations between stocks, bonds, commodities, and currencies.
- Take positions based on the expectation that correlations will perform a certain way.
- Understand that diversified returns streams improve return relative to risk in a portfolio.
- Learn how to engineer returns streams to have low, zero, or negative expected correlation to each other.
- Different asset classes, spread trades, options/derivatives are all viable.
For instance, a trader noticing a temporary breakdown in the long-term non-correlation between stocks and bonds might position in the expectation that stocks and bonds are still inherently diversifying with respect to growth (e.g., bonds outperforming expectations when growth is below expectations and stocks underperforming in that environment, and vice versa).
Pairs Trading Across Asset Classes
While pairs trading is often applied within a single asset class, creative traders extend this concept across different types of assets:
- Identify pairs of assets from different classes that historically move together.
- Monitor the spread between these assets.
- Trade when the spread deviates significantly from what makes sense analytically.
An example might be trading the spread between gold mining stocks and the price of physical gold.
Event-Driven Strategies
Predicting and Trading Around Black Swan Events
This high-risk, high-reward strategy involves:
- Identifying potential low-probability, high-impact events.
- Positioning portfolios to benefit from these events if they occur.
- Using options or other derivatives to express the position and limit downside risk.
Traders might, for example, buy out-of-the-money options that would profit significantly from a sudden market crash (e.g., 2008, Covid) or lurch in a certain direction.
Calendar Anomaly Trading
This approach capitalizes on historical patterns related to specific times of the year, week, or even day:
- Analyze historical data to identify recurring patterns (e.g., the January effect, month-/quarter-/year-end rebalancing).
- Develop strategies to profit from these calendar-based anomalies.
- Monitor whether these anomalies persist or disappear over time.
- Analyze whether they can be front-run.
A trader might, for instance, adjust their portfolio allocation at certain times of the year based on historical performance patterns.
Conclusion
Creative trading strategies offer unique ways to approach the markets to potentially provide an edge.
However, these strategies often require sophisticated technology, access to unique data sources, and analytical skills that cover various layers.
Moreover, as markets evolve and more traders adopt these approaches, their effectiveness may diminish over time.
Many edges don’t last long.
Successful implementation of creative trading strategies typically involves:
- Continuous research and adaptation to changes in markets. If you find some type of edge, you can ride a wave, but it likely won’t be permanent.
- Backtesting and forward testing before real-money implementation.
- Careful risk management to protect against the unique risks associated with each strategy.
- A deep understanding of the underlying principles and potential limitations of each approach.
Financial markets will evolve like they always have, driven by technological advancements, new understanding, and changing global dynamics.
The most successful traders will likely be those who can creatively combine various strategies, adapt quickly to new information sources, and maintain a disciplined approach to risk management.