Multiple-Criteria Decision Analysis (MCDA)
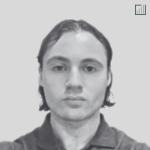
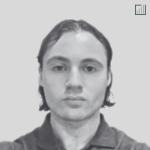
Multiple-Criteria Decision Analysis (MCDA) is a decision-making process involving multiple, often conflicting criteria.
It’s used in fields where decisions can’t be made based purely on a single criterion, like in economics, finance, policymaking, and management.
Key Takeaways – Multiple-Criteria Decision Analysis (MCDA)
- Holistic Evaluation
- MCDA facilitates decision-making by evaluating options against multiple (often conflicting) criteria.
- Weighted Criteria
- It employs weighting systems to prioritize criteria based on their relative importance.
- Aligns decision-making with strategic objectives.
- Diverse Techniques
- Techniques like Analytic Hierarchy Process (AHP), Technique for Order of Preference by Similarity to Ideal Solution (TOPSIS), and Multi-Attribute Utility Theory (MAUT) are used.
- Each are suitable for different decision contexts.
- Quantitative and Qualitative Integration
- MCDA combines quantitative data with qualitative judgments.
- Stakeholder Engagement
- By involving stakeholders in the criteria weighting and evaluation process, MCDA ensures decisions are transparent and consensual.
- Involving various stakeholders enhances acceptability and implementation success.
Core Principles of MCDA
Framework and Methodology
MCDA operates on a structured framework, where decision-makers assess various alternatives based on a set of criteria.
This framework often involves:
- defining the problem
- identifying alternatives
- establishing criteria, and
- evaluating each alternative against these criteria
Criteria Weighting
An important aspect of MCDA is the weighting of criteria.
Different criteria have varying levels of importance, and MCDA methods include techniques for assigning weights to reflect this.
Techniques like Analytic Hierarchy Process (AHP) and pairwise comparison are commonly used for this purpose.
Techniques in MCDA
Multi-Attribute Utility Theory (MAUT)
MAUT is a technique in MCDA used for quantifying and aggregating preferences.
It involves converting different criteria measurements into a single utility value.
This makes it easier to compare different alternatives.
For example, if a trader was following Magical Formula Investing, they might take the two criteria (earnings yield and return on capital) and combine them into a single metric for easy comparison across securities.
Analytic Hierarchy Process (AHP)
AHP simplifies complex decisions by breaking them down into a hierarchy of more manageable sub-problems.
Each element within the hierarchy is compared pairwise to others.
These comparisons are then used to determine the weights of criteria.
Technique for Order of Preference by Similarity to Ideal Solution (TOPSIS)
TOPSIS is a MCDA method that ranks alternatives based on their geometric distance to an ideal solution.
It emphasizes the closest to the ideal and farthest from the worst-case scenario.
Goal Programming
Goal programming is used in situations where decision-makers aim to achieve specific goals.
In this technique, the focus is on minimizing the deviations from these goals across various criteria.
Applications of MCDA
Business and Finance
In finance, MCDA aids in trading and investment decisions, portfolio management, and risk assessment.
It allows for a comprehensive evaluation of options considering multiple financial indicators.
Public Policy and Planning
MCDA is instrumental in public policy, particularly in areas like environmental planning and healthcare, where decisions must balance various societal, economic, and ecological factors.
Technology and Innovation
In technology management, MCDA assists in strategic planning and technology assessment, considering factors like market potential, technological feasibility, and societal impact.
Example Application of MCDA in Trading
In trading, MCDA can be used to select a portfolio by evaluating stocks against multiple criteria such as return rate, risk level, liquidity, and size (market capitalization).
Each criterion is weighted according to its importance to the trader’s strategy.
For instance, a risk-averse investor might assign higher weights to stability and liquidity.
The MCDA process then ranks stocks based on how well they satisfy these weighted criteria.
This can help the trader/investor in choosing a diversified portfolio that aligns with their risk tolerance, investment goals, and market expectations.
Challenges & Limitations
Complexity and Subjectivity
One of the main challenges in MCDA is the complexity involved in handling multiple criteria.
These criteria can be subjective and difficult to quantify.
Data Requirements
MCDA often requires extensive data.
The quality of the decision is heavily dependent on the accuracy and completeness of this data.
Especially in trading, there are various known unknowns (things you know you don’t know) and unknown unknowns (things you don’t even know you don’t know).
Finance is characterized by problems with high dimensionality.
Decision-maker Bias
The process can be influenced by the biases of decision-makers, particularly in the weighting of criteria and the assessment of alternatives.
Conclusion
Multiple-Criteria Decision Analysis is used for making informed decisions in complex scenarios involving multiple criteria (that often represent trade-offs).
Its effectiveness lies in its ability to break down complex problems and objectively assess different alternatives against a set of weighted criteria.
Nevertheless, its application requires careful consideration of its methodologies, the quality of data used, and the potential biases in decision-making.