Does Quant Trading Pose an Existential Threat to Day Traders?
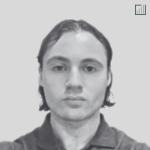
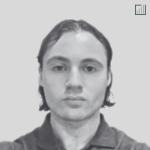
The idea that computer- or algorithmic-driven trading (sometimes called ‘quant trading’) will drive out human traders, or at least make it extremely difficult for them to compete in the markets, is not new. Computers react to news and information within milliseconds that no human trader could ever do. For this reason, it’s practically impossible to trade the news in a way that relies on quick thinking in response to whatever was released.
For example, the jobs report is released in the US on the first Friday of each month. If the number of job adds increases beyond consensus, then the US dollar is likely to see an initial breakout to the upside (i.e., pertaining to reasons of a higher national income relative to expectations). And vice versa if the number of job adds comes in below consensus. When the results are released, the market reaction happens so quickly that a human trader can’t react to the initial report and reliably ride along.
In many ways, the brain is not able to compete with computers in trading and investing pursuits. Computers have much more resolve than any human. They will work around the clock for you. They can process more information, they can do it more accurately, they can process it more quickly, they can produce a wider variety of interpretations and possibilities, and they can do it without the subjective and emotional bias in a way that no human could ever be able to.
When humans interact in groups, the dominant thinking or behavior tends to conform to that of the overall group. Computers are immune to these types of biases and don’t proffer an output simply because it’s popular or part of the consensus-driven narrative.
They also don’t panic or do things that humans have a propensity to do. For example, if stocks go down, humans are often likely to view them as being “bad” investments and commit the fatal mistake of buying high and selling low. A computer, depending on how it’s programmed, is likely to view lower stock prices as containing a higher risk premium (that is, more return relative to a competing asset) and view them as cheaper investments.
Where computers and quant trading are likely to falter
The most common aim in algorithmic trading is to have powerful computers take in enormous amounts of data and look for patterns that can then be used to generate trade ideas or signals.
This is dangerous because it often uses data to over-fit models based on what’s worked in the recent past. Conceptually, this is not all that different from using recent chart patterns to assume that behavior from the past is likely to hold in the future. However, when the future is different from the past and the models are based on relationships that rely on the past being extrapolated into the future in order for it to work, then there’s a problem.
For example, one popular belief in the market currently is that stocks and bonds are inversely correlated. This relationship has largely held since the 1990s and has continued throughout the post-crisis period.
Below is the relationship between SPY (S&P 500 fund) and TLT (long dated US Treasury fund):
This has also pushed down the term premium for longer dated Treasuries.
Term premium refers to the compensation required to take on duration risk. It is largely attributed to investors viewing Treasury bonds as a positive-carry hedge against stocks. In other words, if stocks falter, it is widely believed that safe bonds will help pick up the slack. This is also a cheaper way to hedge than buying put options, bear spreads, and other such hedging structures that cost money. The prospect of being able to generate a return off a hedge has made Treasuries a popular way of reducing equity risk exposure.
However, over the long-run we know that bonds and stocks have little to no correlation. This was true in 2018 when the vast majority of hedge funds lost money. At times, they will positively correlate. So, this isn’t a relationship that we should indefinitely rely on.
Investment rules that are not built upon having deep knowledge of the actual mechanics and cause-and-effect relationships that drive asset prices are at high risk of performing faultily or failing altogether.
At points, what we believe is true about how assets behave, how assets move in relation with each other, and so forth, will not be true. If you looked at a chart of home prices in the US from 1890 to around 2006 you might have extrapolated that they almost never go down.
However, when you look at what a house is as an asset and how it should logically be valued, it is not immune to material drops in its value. And as a large-ticket purchase that is typically done with ample amounts of debt, a drawdown in its value can be devastating to an economy when it happens at a pervasive enough level.
A piece of real estate is an asset whose price is a function of the hypothetical cash flows it would throw off (i.e., if it were to be rented out) discounted back to the present based on an expected return, which is a function of interest rates. Doing these calculations would have shown that prices were very high in the mid-2000s and the speculative lending and leveraging practices of the time were very dangerous and not sustainable.
This is no different than the dynamic that affects all asset classes. However, for those using data mining and extrapolating trends based on the past and overfitting models to rely on what’s mostly happened in the past, they would have missed this altogether. It’s the same for trading systems that look at past charts and try to guess where price will move based on support, resistance, moving averages, and various other conversions of price and volume. While looking at charts can be helpful, using that as a standalone system is naïve because what led to that type of price behavior in the past isn’t likely to transpire when the future is different.
Moreover, when certain decision outputs become used frequently enough in a market, this impacts the price. Any value inherent in it will disappear. And those using it without having the deep knowledge of the cause-and-effect relationships to understand whether it’s logical to apply it or not won’t know if the past is relevant to the current situation. And even if such a thing were to have value, they wouldn’t know if it has lost its value. Without knowing the fundamental relationships that cause assets to move like they do and be valued what they are, they won’t know if what they’re using is simply data-mined bunk.
As another example, for a long time, many traders have relied on relationships such as the Australian dollar’s correlation to iron ore. As a commodity exporter, Australia’s terms of trade improve when iron ore is priced higher. However, there are many other exogenous factors that influence the price of the AUD – e.g., interest rates, inflation rates, current account surplus / deficit, balance of payments, politics, speculative activity, economic growth rates – that are all outside the price of any given commodity. This relationship has recently broken down and hurt traders who had excessively relied on it holding.
Conclusion
Computers have great advantages over humans in many respects and will take the place of human traders in various contexts (e.g., trading the news). But the output is only as good as how well the system is programmed. Too many “trading signals” generated by institutional programs are generated based on correlations that are not actually inherently causal in any manner. Leveraging over-optimized strategies that have not been well stress-tested can be destructive.
Computers may be able to crunch more data faster and more accurately than any human. But they also lack common sense and can draw spurious correlations. Ice cream doesn’t cause hot weather, though a computer might infer that if the two datasets were fed into it. The number of computer science graduates and the number of flat-screen TV owners have some positive correlation to each other (both going up), but that doesn’t mean one causes the other.
When considering whether a trading system is likely to have everlasting value, one must consider whether the algorithms and decision rules fed into it are deeply logical.