AGI in Finance, Markets & Trading
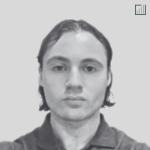
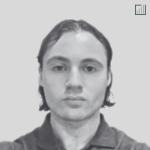
Artificial General Intelligence (AGI) in the context of finance, markets, and trading refers to the development of AI systems that possess the ability to understand, learn, and apply intelligence across a wide range of financial activities in a manner similar or better to human intelligence.
AGI would differ significantly from the current state of AI, which is largely Narrow AI, specialized in specific tasks.
Key Takeaways – AGI in Finance, Markets & Trading
- AGI (a still-theoretical concept) could alter the future of trading by synthesizing vast market data for better prediction and decision-making, beyond current AI/ML capabilities.
- Real-time, dynamic risk management and strategy adjustment in trading could be significantly enhanced with AGI’s advanced cognitive abilities.
- AGI’s potential in offering personalized financial advice and portfolio management could outperform existing automated systems and help tailor strategies to individual profiles.
Applications of AGI in Finance, Markets & Trading
In finance, markets, and trading, the potential applications of AGI are vast:
Market Analysis and Prediction
AGI could synthesize and analyze vast amounts of data, including market trends, economic reports, and news, to make predictions about market movements.
It could identify potential trading/investment opportunities or risks.
AGI could also help improve asset allocation decisions.
Automated Trading
AGI could manage complex trading strategies across multiple markets and asset classes, dynamically adjusting strategies based on changing market/economic conditions.
Risk Management
AGI could evaluate and manage risks in real-time.
It could consider a broad range of factors from market volatility to geopolitical events and provide a stronger risk assessment than current models.
Personalized Financial Advice
AGI could offer highly personalized financial advice and consider an individual’s complete financial situation, personal goals, and market conditions.
Regulatory Compliance and Fraud Detection
AGI systems could monitor transactions to detect and report suspicious activities.
It could improve fraud detection and regulatory compliance while adapting to new regulatory changes.
Portfolio Management
AGI could manage diverse portfolios, continuously optimizing asset allocation based on changing markets, individual investor goals, risk profiles, and long-term financial goals.
Economic Analysis and Forecasting
AGI could analyze global economic trends and provide forecasts.
This could help governments and organizations make informed policy and business decisions.
Challenges of AGI in Finance
The implementation of AGI in finance has challenges:
Complexity of Financial Markets
Financial markets are influenced by many factors and have significant dimensionality, and the relationships between variables is often non-linear and complex.
Regulatory and Ethical Considerations
The use of AGI in finance raises significant regulatory and ethical questions – particularly regarding accountability, transparency, and data privacy.
Technical Challenges
Building an AGI system that truly understands and adapts to the dynamic nature of financial markets is a significant technical challenge.
AGI vs. Machine Learning & Deep Learning
AGI and machine learning (including deep learning) represent different scopes and capabilities within the AI spectrum.
AGI
AGI refers to the theoretical intelligence of a machine that could successfully perform any intellectual task that a human being can – and beyond that by advancing intelligence itself.
It implies an ability to understand, learn, and apply its intelligence broadly and flexibly, akin to human cognition.
Machine Learning & Deep Learning
Machine learning, on the other hand, is a subset of AI focused on algorithms that enable computers to learn from and make predictions or decisions based on data.
It includes a range of techniques, with deep learning being a subset that uses layered neural networks to analyze various factors in large data sets.
Deep learning excels in pattern recognition and has driven significant advancements in areas like image and speech recognition.
Machine learning and deep learning have seen widespread practical application and have achieved great results, but within specific tasks.
But they still operate within the confines of their training and predefined parameters.
As another example, LLMs are a popular example natural language processing (NLP) technology, but their focus is mainly on the structure of text rather than about whether what they say is true.
Many AI/ML/DL applications have to be combined with various tools to take advantage of what they’re good at and protect them from what they’re bad at.
For example, LLMs are great at theorizing about things and emulating human intuition, but have to be combined with, e.g., more statistical tools to ensure their accuracy is kept high.
Likewise, if you feed a computer lots of historical market data, you gain a lot of statistical understanding from that.
But it’ll have limited ability to understand, e.g., concepts like “greed,” “fear,” and so on because it isn’t aware of the human condition in which those results are produced.
This is where LLMs can provide a perspective of this because it’s read more or less everything there is to read (to the extent of its training data) about the emotions in the context of those market conditions.
Machine learning and deep learning applications lack the general-purpose, adaptive intelligence proposed by AGI, which would theoretically enable a system to tackle any problem with (super)human-like understanding and flexibility.
Conclusion
AGI has not yet been realized and remains a theoretical concept.
Today’s quantitative trading systems are generally categorized as expert systems (humans derive the algorithms) and data mining systems (computers derive the algorithms).
The current AI applications in finance are still considered Narrow AI, specialized in specific tasks with limited generalization capabilities.