Stratified Random Sampling
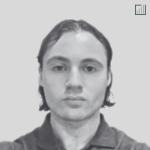
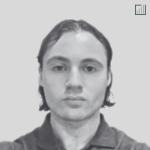
Stratified Random Sampling is a method of sampling that is used when dividing a population into sub-groups, or strata, is necessary.
This technique is often used when there is a need to ensure that the sample accurately represents the characteristics of the entire population.
To stratify a population, researchers first identify the key characteristics that are important for the study. Then, the population is divided into strata based on these characteristics.
Once the population has been stratified, a random sample is taken from each stratum. This ensures that each sub-group is represented in the sample.
Stratified random sampling is an effective way to obtain a representative sample, but it can be time-consuming and expensive.
How Stratified Random Sampling Works
There are four steps in stratified random sampling:
Identify the strata
The first step is to identify the key characteristics that are important for the study (e.g., age, race, income). These characteristics will be used to divide the population into strata.
Divide the population into strata
Once the key characteristics have been identified, the population can be divided into strata. Each stratum should be homogeneous with respect to the characteristics that are being studied.
Select a random sample from each stratum
After the population has been stratified, a random sample is taken from each stratum. This ensures that each sub-group is represented in the sample.
Calculate the results
Once the data has been collected, it can be analyzed to answer the research question.
This method is often used when there is a need to ensure that the sample accurately represents the characteristics of the entire population.
Stratified Random Sampling & Use in Trading and Financial Markets
How is stratified random sampling used in trading and financial markets?
There are many applications for stratified random sampling in the financial world. For instance, it can be used to create a more representative sample of consumers for market research purposes.
By stratifying the population by factors such as age, income, and location, researchers can ensure that the resulting sample is more reflective of the population as a whole. This is important in fields like marketing, where understanding consumer behavior is essential to a company’s success.
In addition, stratified random sampling could be used to create models of financial market participants. By taking into account the different characteristics of different types of traders and investors, stratified random sampling can help to create more accurate predictions about market behavior by taking into account their characteristics and likely motivations.
For instance, if a country’s retail trader and investor base is getting progressively older on average, how might that impact market dynamics?
China’s equity markets are known to be more momentum-driven than those of the US because there is a higher retail base and more of that retail base is younger, which fuels more risk-taking and self-reinforcing dynamics.
The process is also often used for studying population demographics and life expectancy, which are variables that often tie into insurance markets, in particular.
For example, if a study is interested in examining the relationship between income and health, the population could be stratified by income level.
Simple Random vs. Stratified Random Sample: What’s the Difference?
There are two main types of random sampling:
- simple random sampling and
- stratified random sampling
Simple random sampling is the most basic form of random sampling. Each member of the population has an equal chance of being selected for the sample.
This method is quick and easy, but it can be less effective than other methods, such as stratified random sampling.
Stratified random sampling is a more complex method involving dividing the population into sub-groups, or strata, based on specific characteristics they have.
A random sample is then taken from each stratum to help ensure that each sub-group will be represented in the sample.
The main difference between simple random sampling and stratified random sampling is that stratified random sampling involves dividing the population into sub-groups before taking a sample, while simple random sampling does not.
Both methods are used to select a random sample from a population. But stratified random sampling is often used when there is the need to ensure that the sample accurately represents the characteristics of the whole population.
Advantages and Disadvantages of Stratified Random Sampling
There are both advantages and disadvantages to using stratified random sampling.
Some of the advantages include:
- More accurate representation of the population
- Ability to study sub-groups within the population
- More efficient use of resources
Some of the disadvantages include:
- Can be time-consuming and expensive
- Requires a large amount of data to be collected
- May not be appropriate for all research questions
Simple vs. Stratified Random Samples – Which is Best?
There is no one-size-fits-all answer to this question. The type of random sample that is best for a particular study depends on the research question and the population.
Simple random sampling is the most basic form of random sampling. Each member of the population has an equal chance of being selected for the sample.
This method is quick and easy to complete, but it can often be less effective than other methods, such as stratified random sampling.
Stratified random sampling is a more complex method that involves dividing the population into sub-groups, or strata, based on certain characteristics.
A random sample is then taken from each stratum. This ensures that each sub-group is represented in the sample.
Stratified random sampling is a good way to get a representative sample, but it can take time and is expensive.
FAQs – Stratified Random Sampling
When would you use stratified random sampling?
There is no one answer to this question. It depends on the research question and the population.
Stratified random sampling is often used when there is a need to ensure that the sample accurately represents the characteristics of the whole population.
What is proportionate and disproportionate sampling?
Proportionate sampling is a type of stratified random sampling in which the size of the strata is proportional to the size of the population.
Disproportionate sampling is a type of stratified random sampling in which the size of the strata is not proportional to the size of the population.
How are strata chosen for stratified random sampling?
Strata are chosen based on the characteristics of the population.
For example, if a study is interested in examining the relationship between income and travel behavior, the population could be stratified by income level.
Other examples of strata include age, gender, race, and physical location.
How do you use a simple random sample to study a larger population?
A simple random sample can be used to study a larger population by using the results of the sample to make inferences about the population.
This is done by using statistics, such as means and proportions, to estimate the characteristics of the population.
What is the difference between systematic sampling and cluster sampling?
Systematic sampling is a type of probability sampling in which every kth member of the population is selected for the sample, where k is the sampling interval.
Cluster sampling is a type of probability sampling in which groups, or clusters, of members are selected for the sample.
The main difference between these two methods is that systematic sampling involves selecting individuals from the population, while cluster sampling involves selecting groups of individuals.
Both methods are used to select a random sample from a population.
What is the difference between stratified random sampling and random assignment?
Stratified random sampling is a method of probability sampling in which the population is divided into sub-groups, or strata, based on certain characteristics.
A random sample is then taken from each stratum.
Random assignment is a method of assigning individuals to groups, such as treatment and control groups, in a study.
The main difference between these two methods is that stratified random sampling is used to select a sample from a population, while random assignment is used to assign individuals to groups.