Heteroskedasticity – Meaning, Types, and Applications
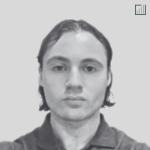
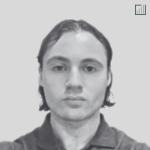
Heteroskedasticity is a condition in which the variance of a statistical variable is non-constant across values of that variable.
In other words, heteroskedasticity occurs when the size of the error term varies with the values of the independent variables.
Heteroskedasticity – Key Takeaways
- Heteroskedasticity is a type of statistical error that results in inconsistent or incorrect estimates of parameters in a regression analysis. This can lead to inaccurate predictions and sub-optimal decision-making.
- There are several methods for dealing with heteroskedasticity, including transforming the data, using weighted least squares regression, and using robust standard errors.
- Heteroskedasticity can be caused by a variety of factors, including outliers, misspecification of the model, and non-normal distributions.
- Heteroskedasticity is a common issue in econometric studies, so it is important to be aware of its effects and how to deal with it.
- In general, heteroskedasticity is not a serious problem if the estimates are unbiased and the standard errors are correctly estimated. However, it can still lead to some problems with inference if it is not taken into account.
If you’re doing any kind of statistical analysis, it’s important to be aware of heteroskedasticity and how it can affect your results.
Types of Heteroskedasticity
There are two main types of heteroskedasticity:
- conditional and
- unconditional
Conditional heteroskedasticity exists when the variance of the error term is a function of one or more independent variables.
Unconditional heteroskedasticity exists when the variance of the error term is not a function of any independent variables.
Applications of heteroskedasticity include improving forecasting accuracy, detecting omitted variable bias, and detecting model misspecification.
Additionally, heteroskedasticity can be used to improve the efficiency of estimators.
For example, in the case of OLS estimators, heteroskedasticity-consistent (HC) standard errors can be used to correct for the bias that arises from heteroskedasticity.
What Causes Heteroskedasticity?
There are a number of factors that can cause heteroskedasticity.
One common cause is omitted variable bias. Omitted variable bias occurs when an important explanatory variable is not included in the model.
This can lead to underestimation of the true effect of the omitted variable on the dependent variable, and consequently, to heteroskedasticity.
Example of omitted variable bias and heteroskedasticity
For example, if one was building a model of the factors that lead to high outside temperature and the lone independent variable was ice cream consumption, the model might say that ice cream consumption causes hot weather.
Obviously, this is not the case and the effect of omitted variables would lead to high hetereoskedasticity.
Another common cause of heteroskedasticity is model misspecification.
This occurs when the functional form of the model is not correctly specified. For example, if a linear model is used to model a non-linear relationship, this will lead to heteroskedasticity.
Additionally, incorrect data transformations can also lead to model misspecification and consequent heteroskedasticity.
For example, using logarithmic transforms on variables that are already logarithmically transformed will lead to heteroskedasticity.
Finally, outliers can also cause heteroskedasticity.
Outliers are extreme values that lie far from the rest of the data. They can have a significant impact on estimation and, in some cases, can lead to heteroskedasticity.
How to Test for Heteroskedasticity?
There are a number of methods that can be used to test for heteroskedasticity.
White test
The most common method is the White test, named after Halbert White who developed the test in 1980.
The White test is based on the OLS estimator and tests whether the variance of the error term is constant across all values of the independent variables.
To perform the White test, one first calculates the following statistic:
R^2 / (1 – R^2) * (n – k – 1)
Where:
- R^2 = the coefficient of determination
- n = the number of observations in the sample
- k = the number of independent variables in the model
This statistic is then compared to a chi-squared distribution with k-1 degrees of freedom.
If the statistic is greater than the critical value from the chi-squared distribution, then heteroskedasticity is present.
Breusch-Pagan and Goldfeld-Quandt test
Other methods for testing for heteroskedasticity include the Breusch-Pagan test and the Goldfeld-Quandt test.
How to Correct for Heteroskedasticity?
There are a number of methods that can be used to correct for heteroskedasticity.
Weighted least squares (WLS)
One common method is weighted least squares (WLS). WLS is a variant of OLS where the error term is weighted by the inverse of the variance.
This has the effect of giving more weight to observations with lower variance and less weight to observations with higher variance.
Robust standard errors
Another common method is using robust standard errors. Robust standard errors are standard errors that are computed in a way that is robust to heteroskedasticity.
There are a number of different methods for computing robust standard errors, but one of the most common is the White estimator.
Heteroskedasticity and Financial Modeling
Heteroskedasticity can have a number of implications for financial modeling.
Incorrect estimates of model parameters
First, it can lead to incorrect estimates of model parameters.
This is because OLS estimators are biased when heteroskedasticity is present.
Incorrect inferences about the model parameters
Second, it can lead to incorrect inferences about the model parameters.
This is because the standard errors of the model parameters are also biased when heteroskedasticity is present.
This means that, in a statistical sense, one might erroneously conclude that a parameter is statistically significant when it is not, or vice versa.
Incorrect forecasting performance
Finally, heteroskedasticity can also lead to incorrect forecasting performance.
This is because OLS-based forecasting models are also biased when heteroskedasticity is present.
Thus, it is important to be aware of the potential impact of heteroskedasticity on financial modeling, and to take steps to correct for it if necessary.
Heteroskedasticity summary
FAQs – Heteroskedasticity
What is heteroskedasticity?
Heteroskedasticity is a type of statistical error that can occur when modeling data.
It occurs when the variance of the error term is not constant across all values of the independent variables.
What are some common causes of heteroskedasticity?
There are a number of common causes of heteroskedasticity, including incorrect data transformations, outliers, and misspecified models.
How can I test for heteroskedasticity?
There are a number of methods that can be used to test for heteroskedasticity, including the White test, Breusch-Pagan test, and Goldfeld-Quandt test.
How can I correct for heteroskedasticity?
There are a number of methods that can be used to correct for heteroskedasticity, including weighted least squares and robust standard errors.
Conclusion – Heteroskedasticity
Heteroskedasticity is a type of statistical error that can occur when data points are not evenly distributed.
This can happen for a variety of reasons, but heteroskedasticity often occurs when there is a large amount of variability in the data.
Heteroskedasticity can be problematic because it can lead to inaccurate results in statistical tests.
For example, if a data set is heteroskedastic, the standard error of the mean will be underestimated. This can cause confidence intervals to be too narrow, and tests of statistical significance to be inaccurate.
Heteroskedasticity can be corrected with a variety of methods, including transformation of the data, weighting of the data points, or use of more robust estimation methods.
Correcting for heteroskedasticity is important in order to ensure that statistical results are accurate.
Related